Hi @anne_sa ,
From what it sounds like, you have one factor that is discrete numeric, call it X1 with four levels. You also have another factor that is a two-level categorical, call it X2. X1 can take on values [1, 2, 3, 4] these are the coded values, not the actual experiment values that you would really use. Similarly, your X2 categorical variable takes on [0, 1], which are again the coded values -- in the experiment, X2 is going to actually be A or B. Putting this into a typical custom DOE, you should get 8 runs where X1 varies from 1 to 4 and X2 will either be 0 or 1, will also get the interactions as well. The interpretation then is that 0=A and 1=B.
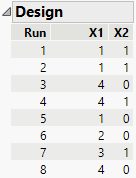
On the other hand, if you have two components A and B that can both either be present (1) or absent (0), then you'll want to have an additional categorical variable X3, which also takes values [0, 1], but now the [0, 1] means off or on, respectively. If you now generate the new DOE with three factors, you'll need at least 16 runs to also get the interactions. Again, in this case, X1 runs from 1-4 and the other two categorical variables for component A or B will either be on, off, or mixtures of on/off, e.g X2=0 (off), X3=1(on). Adding the additional term allows you to see if there are any interactions between component A and component B, along with concentration.
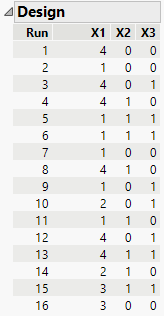
These are not necessarily optimal DOEs, especially for minimizing correlation of the interactions, etc. You might need to have more center runs, run replicates or change the design to what works best for you.
I hope I understood your issue correctly.
Hope this helps!,
DS