Your first constraint is inconsistent and it creates a singularity in the design. First of all, none of the first three factors can exceed 8,000, so an upper limit of 10,000 does not make sense. Secondly, you only have 2 independent factors. The third factor is completely determined by the sum of the first two factors. So you could design the experiment with just the first two factors and then use a column formula to determine the setting of the third factor in order to make a run or you can design the experiment with all three factors but remove the third from any model.
Here are my results by way of an example.
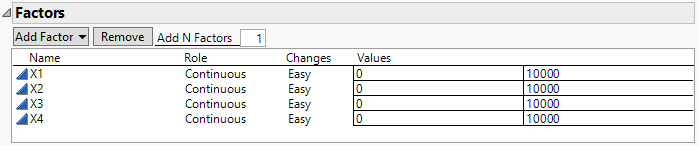
The upper bounds are not realistic for your experiment.
Here is the definition of the constraints that I used.
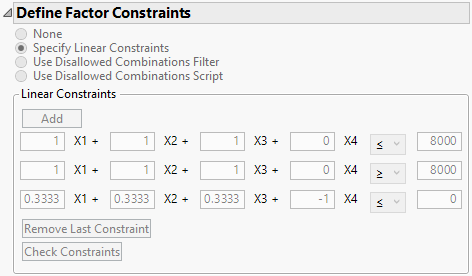
Together with the model containing only the intercept and main effects, I get this design with warnings.
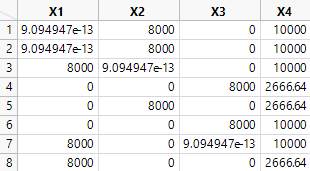
The very small values can be replaced by zero. I simulated the data to show the analysis.
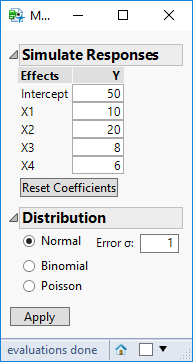
I begin the analysis as usual but I remove any terms with X3. Here is the result.
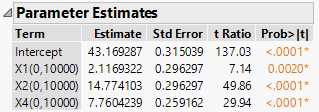
Note that these estimates won't match the simulation because they used coded factor levels. The model does, though, predict very well.
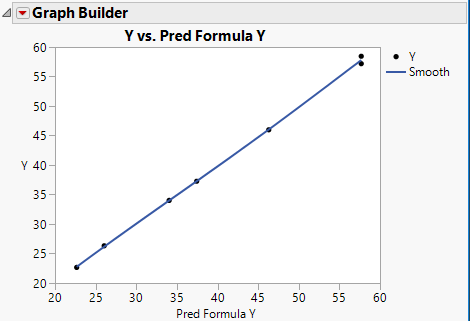
Another approach that might work is to treat this experiment as a mixture design. That approach will require you to rescale your factors as 0 to 1 or 0.8. Then change the mixture sum to 0.8. You would only enter X1 and X2, not X3, in this approach. You would only need one constraint for the X4 requirement with a modification to cover that X3 is no longer explicitly in the design.