My analysis produced the below p-value table. I have made some conclusions based upon the results, but am unsure whether I am on the right track given the multiple significant interactions and main effects, specifically if I have reached too far.
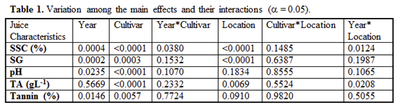
Preliminary results of this study indicate that soluble solids content and specific gravity differed across years, cultivars, and locations, but for SSC the difference in location depended on year. Titratable acidity differed across the cultivars and locations, and the difference in locations also was dependent on year. Juice pH and total tannins differed across years and cultivars. Significant variation in annual factors could explain the interactions observed for SSC and TA (Table 3; Figure 1).
TO MAKE IT CLEAR- I AM NOT LOOKING FOR ADVICE ON THE P-VALUES OR INTERPRETATION, RATHER MY ABILITY IN MAKING CONCLUSIONS GIVEN THE SIGNIFICANT OR LACK THEREOF INTERACTIONS AND MAIN EFFECTS.
1) For SSC, I reran the model eliminating the Cultivar*Location interaction as it was insignificant ( p = .149, a = 0.05), and the Year*Cultivar interaction became insignificant. So, was it correct to make the conclusion that the main effects (Year, Cultivar, and Location) were significant, but that the effect of Location was dependent on Year? I believe I am.
2) For TA, I followed the same steps as SSC and came up with the same conclusion in that the effect of Location was dependent on Year. However, in this case the main effect of Year was actually never significant as it was for SSC (p = 0.567 vs. p = 0.0004). Is my conclusion still fine?
Thank you ahead of time for any input!!!