I am glad that my explanation helped some.
When it comes to the cubic term, what does a plot of the raw data versus the factors look like? Knowing the science behind your data, does it make sense that you would see a cubic relationship? It looks like you have one point that was not predicted well when the prediction of stress was near 1700. Have you verified that the data is real and not an outlier? Cubic terms do not appear that often, so people often question when they do appear. But it very well could be real, and based on your residual plot, I understand why you added a cubic term.
As for the D and I-optimality stuff, it is easy to think that the most precise parameter estimates (D-optimal) will naturally lead to the lowest prediction error (I-optimal), but that is not necessarily the case. Consider the case of only one predictor variable, X, and a total of 9 runs. If I want a quadratic model, we will need to study 3 levels of X. If I want the best possible parameter estimates, I would put 3 runs at the lowest setting for X, 3 runs at the middle setting, and 3 runs at the highest setting. I need to put more runs at the extremes to better model that linear term. This is what a D-optimal design does.
What about for prediction error? Well, prediction error is "minimized" somewhere between the low and mid settings and again somewhere between the mid and high settings:
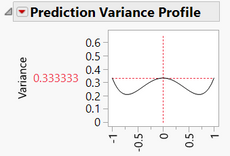
How could I get rid of the hump in the middle? Move some points from the ends to the middle. This is what the I-optimal design does. It uses two points at the ends of the X-range and places 5 points in the center. This will lower the prediction variance in the center, but the ends will be raised.
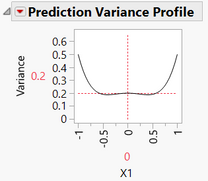
Remember, I-optimal is for integrated variance, so the "area under the curve" is minimized this way. This is why when people are trying to optimize a process, they want the optimum to be somewhere near the middle of the design space because an I-optimal design provides the best predictions in that area.
If you like mathematics and matrix algebra, remember that your model is:
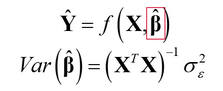
D-optimal designs work on minimizing Var(B-hat).
The I-optimal designs focus on the left-side of that equation. Specifically,
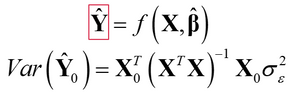
and minimize the integrated variance of Y-hat. Notice that the variance in this formula is for a specific point of your inputs (X sub-0). The variance is calculated over the entire design space and integrated for I-optimal designs.
I hope this helps.
Dan Obermiller