I am trying to set up a DoE in my organization. This is the first time ever we are using this approach, there a couple of people watching and I want to get this right to underline the value of DoE and JMP. Therefore, I hope it is acceptable to post this question here. Example tables are attached.
This is the situation. We have:
- 4 continuous responses:
- Yield_A
- Yield_B
- Impurity_A
- Impurity_B
- 4 continuous factors:
- Reagent concentration
- Reaction time
- Reaction temperature
- Reagent-to-reactant ratio
- 2 covariates for the raw material to be used for testing, both continuous
- Concentration_compound_X
- Concentration_compund_Y
- One 2-level nominal factor, which I presume can be interpreted both as blocking factor and covariate. It is suspected to influence the response, and it is directly tied to Concentration_compound_X and Concentration_compound_Y via a batch ID:
We want to create a DoE for a model considering main effects, all 2-way interactions, the two covariates as well as the ProcessType. Note again, that the raw material used in this experiment can come from two different processes (Type _1 and Type_2). Each raw material batch has its unique set of Concentration_compound_X and Concentration_compound_Y levels that will be used as covariates in the design.
I am having issues understanding how to best add the covariates and ProcessType to the design. I am not able to add ProcessType as a blocking variable. After initial investigation we decided to go for a custom design. My understanding fails me after having loaded the four continuous responses and the four continuous factors into the DoE dialogue. This is were the saga begins.
Case 1 (Adding both covariates and ProcessType as “easy to change” covariates):
Factors and responses are already in the dialogue window. Then, I press the “Select Covariate Factors” button and add the covariates as shown below. The covariate table has 900 rows, and each row represents an individual batch.
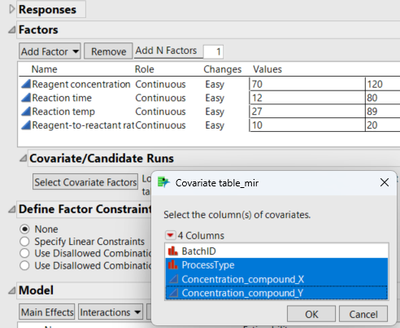
I leave all factors at the default setting “Changes = Easy”:
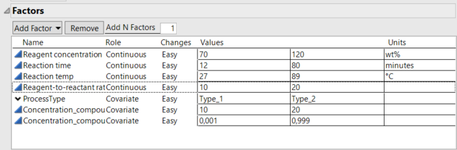
After adding the interactions, the design ends up with 900 runs.
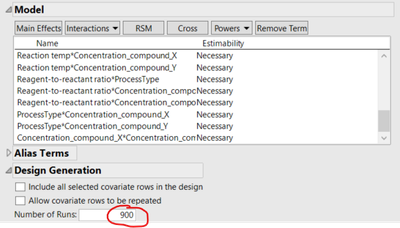
Clearly, this is a bogus design. No one will perform 900 runs, at least not voluntarily.
Case 2 (Adding both covariates and ProcessType as “hard to change” covariates)
In this example, the covariates and ProcessType were all added with role “Covariate” again, but factor change was set to hard. This creates a whole plot design with default 45 runs:
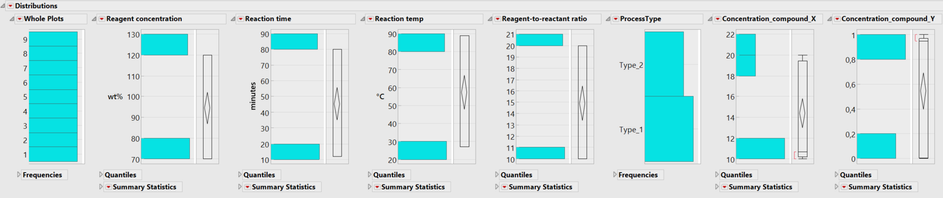
JMP allows the user to reduce the number of runs further, which is good. However, there are no center points in the design and I also can’t add replicates …
Thoughts & questions:
- Is case #2 an acceptable design?
- Between the two cases above, the sensible design obviously is case #2 but I am wondering why JMP doesn’t allow me to add center points and/or replicates.
- Setting factor changes to hard for the two covariates and ProcessType appears realistic, as it is possible that manufacturing will be stuck with a single raw material batch. But is this choice correct? We can pick almost any batch we like for the experiment and the only reason why factor change was set to hard is to avoid generating a 900-run design.
- I am a little bit surprised with the 900 runs generated in case #1. Am I misled in believing the design generating algorithm should pick a suitable number of batches and runs? Or is it my responsibility as user to tell JMP the number of runs I find suitable, evaluate the design and decide whether I need to improve on it by adding more batches from the covariate table?
Thank you for enlightenment!