Hi @Josh1,
As @P_Bartell mentioned, it is probably best to start with a U-chart to see a shift in % scrap over time. WK as X, % Scrap as Y and Before and After as Phase in control chart builder. IF you think you have enough weeks data collected for after and seen a shift downwards, then there is probably a positive change in the process.
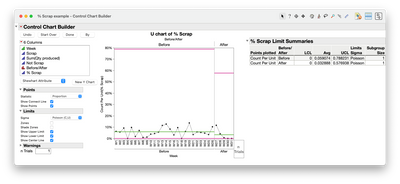
A naive statistical test you can use is a contingency analysis to see if there is an association between before and after change like you have have started to try. WARNING: This is not the best test for your data since there is time dependency in the first few weeks of the change (adaptation to new process) so I would be careful of interpret the results. There could be a statical difference, but the first few weeks will likely throw it off. After collecting some more weeks of data, you may come back to this test with the caveat of removing the first two to three weeks of data.
You are on the right path for using fit Y by X for contingency analysis. The data entered is not quite correct. You need a stacked table. X would be Before/After and Y would be Scrap/Not Scrap and the frequency would be count.
To do this, you would first subtract Scrap qty from Qty Produced to get Not Scrap count. This can be done by selecting both columns the using a right click and choosing New Column Formula>Combine>Difference (reversed order)
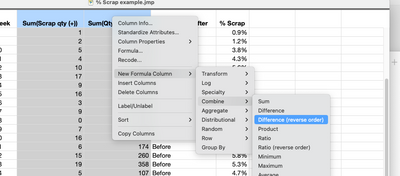
I renamed the columns to Before/After, Scrap and Not Scrap.
Next is to summarize Scrap and Not Scarp using Sum within Summary under tables and using Before/After as Group (this is similar to what you have done before). Then stack Sum(Scrap) and Sum(Not Scrap) so you now have 3 columns (using Stack under Tables menu)
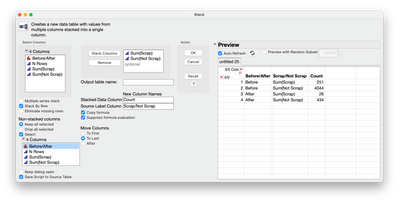
Now you can use the new table for Fit Y by X like you did before.
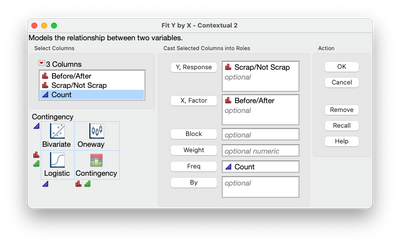
If you then use Two Sample Test for Proportions and also look at analysis of means for proportions, it will look like there is no difference, but the after decisions limits are quite wide due to the sample size and the first two weeks being higher than the next three weeks (a trend in the data).
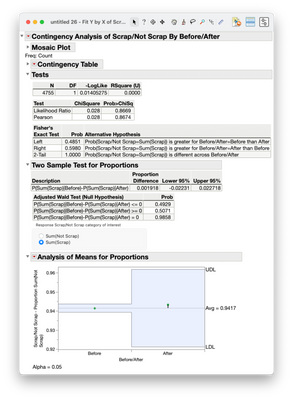
I would not trust this result due to the trend in the data as seen in the U-chart above (as @P_Bartell mentioned is the first step). But I think the U-chart is telling that something is beginning to happen with the change in process and is worth showing if some evidence is needed for preliminary validation of the process change is having an affect.
Hope this helps at least how one might go about it in JMP. I think more weeks of data is needed or use another type of statistical test that can accommodated trends in data. I cannot advise which tests but I am sure others here can.
Best,
Chris Kirchberg, M.S.2
Data Scientist, Life Sciences - Global Technical Enablement
JMP Statistical Discovery, LLC. - Denver, CO
Tel: +1-919-531-9927 ▪ Mobile: +1-303-378-7419 ▪ E-mail: chris.kirchberg@jmp.com
www.jmp.com