So you designed an experiment for a computer simulation? That is, the factor levels are used as parameter values in the computer simulation?
I instead interpret your question to be about regression results. The two-level fractional factorial design in 16 runs with 2 center points and 2 ad hoc runs does not support estimating the full quadratic model. In this case, you must not try to estimate the parameters for the quadratic terms.
The Screening platform is intended only for two-level factors. You used a two-level design but you added a third or fourth level with the center points and ad hoc runs. This platform won't necessarily give you wrong information but it won't necessarily give you correct information when there are more than two levels.
Still, it can be useful as an exploration tool and a starting point leading to a full regression analysis. I used it to find a model for Output A with the linear predictor including Input E, Input D, Input A, and Input E*Input E. So it detected a non-linear response but attributing it to Input E is arbitrary. It is based on the heredity principle and nothing more. It cannot be established with this experiment. Output B does not appear to have any fixed effects from these factors. Output C has the most complex model. It includes two quadratic effects, which is the most that might be estimated and the choice of which terms is again arbitrary. The usual action at this stage is to augment the two-level design specifically to estimate all the quadratic terms for a final decision.
Another approach is to use the Stepwise platform starting with all the potential terms (main effects and interactions) that might be estimated. Here is the prediction profiler launched with the model formulas saved for Output A and Output C using stepwise regression to select the model.
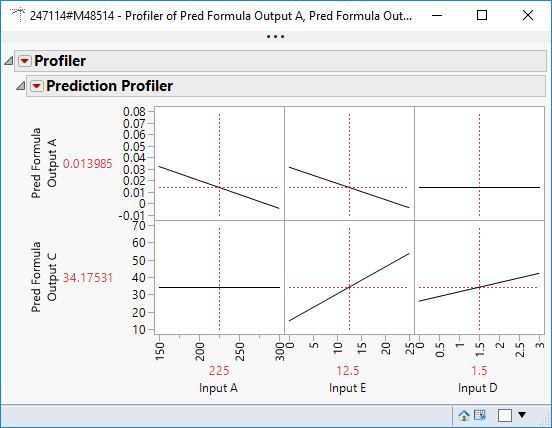
Another approach is to start with the main effects only, remove the unimportant factors, then add and test interactions involving the important factors. This approach is based on the hierarchy and heredity principles.
Just remember that there are many confounded and correlated effects using this design. It provides incomplete information about the response. So augmentation might be the best next step to clarify the important effects. Regardless, the final model selected here or after augmentation must be empirically verified with new, independent runs for as yet untested treatments.