Hi @OmegaHard ,
I think you might be graphing something different than what you're looking at in the table. In the tables, you're looking at the confidence interval on the mean, not the prediction. Read about some of it here. What I think you're intending to look at is what JMP call the "indiv" confidence interval. According to JMP's online help:
Mean Confidence Limit Formula
Creates two new columns in the data table called Lower 95% Mean <colname> and Upper 95% Mean <colname> where colname is the name of the Y variable. These columns contain both the formulas and the values for lower and upper 95% confidence limits for the mean response.
Indiv Confidence Limit Formula
Creates two new columns in the data table called Lower 95% Indiv <colname> and Upper 95% Indiv <colname> where colname is the name of the Y variable. These columns contain both the formulas and the values for lower and upper 95% confidence limits for an individual prediction.
Note also that there is a difference between Confidence Shaded Fit (confidence region for the mean expected response) vs Confidence Shaded Indiv (confidence region for an individual prediction). I believe the want the Indiv and not the Fit.
Confid Shaded Fit
(Not available for all fits.) Shows or hides a shaded confidence region for the expected response (mean).
Confid Shaded Indiv
(Not available for all fits.) Shows or hides a shaded confidence region for an individual prediction.
If you look at the Indiv CI shaded and saved formulas, you get exactly what you expect. Notice the difference between the Fit vs Indiv -- the Fit is much narrower because it's about the overall mean response, whereas the Indiv is an individual prediction. This is all copied from your data tables you shared.
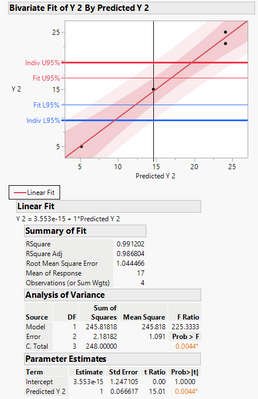
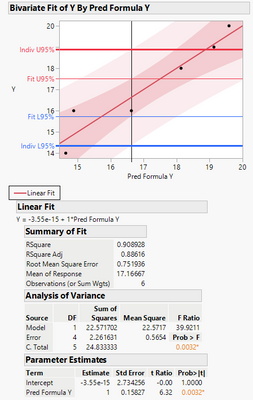
Also, make sure that when you're comparing the CIs for your actual by predicted plots, and those saved to the data table, that you do it with the actual by predicted data and not accidentally save the CI formulas from the Fit Y by X (using X as the regressor).
The actual calculation of the 95% confidence intervals for the indiv prediction is somewhat complicated and you can see it by looking at the formula in the relevant column, for example:
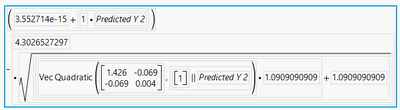
Calculating it by hand is not very easy, but it's possible. A quick Google search brings up this, which might help you get started, but doing this calculation isn't something easy like just adding a plus or minus.
Here's the data table I used when trying to replicate your issue.

Hope this helps,
DS