As analytic practitioners we rely on data to make judgments in everything we do. But does your data reflect reality? What am I getting at? What more truth is there than raw data? And what is more exciting than turning that data into actionable information? To answer this question, let me tell you a story about myself that some of you might be able to relate to. Over the years I have put on a few excessive pounds that I am trying to get rid of. As a good statistician, I gather data on my progress daily by stepping on the scale. Here is the dirty little secret that most of you can relate to: if I don’t like the number that I see, I often step off the scale and get back on and guess what half the time I see a better answer!
When we get data we are looking at the truth through the prism of a measurement process and just like any other process it can have issues with Precision (variability) and Accuracy (bias). This begs the question of what is a data geek supposed to do? Luckily JMP supports a process called Gauge R&R that helps us to set up an experiment and understand the Accuracy and Precision of our measurement system.
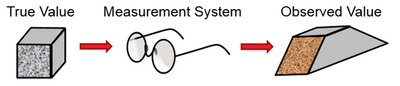
To answer the Accuracy question, we need to obtain the truth by getting a NIST traceable standard. Now this standard should be as representative of what we will be measuring as possible. This can sometimes take a bit of creative thinking as standards usually aren’t objects that we are measuring in our processes. I will leave that discussion for another time, but trust me if you think creatively about what you are measuring you can usually get something that is representative of your process.
Your experiment for estimating the accuracy or bias should be done in a short time frame by measuring the standard multiple times in a short period of time under as similar conditions as possible. This also gives us an estimate of the short term variability called Repeatability. To estimate the bias use the “Distributions” platform in JMP on the measurements you take on the standard.
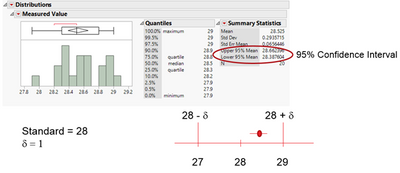
To understand if the bias is acceptable compare the 95% CI to the true standard and see how that interval corresponds to the standard +/- acceptable bias.
An estimate of the short term variability is the Std Dev from the data you took in the above step. This also gives you a chance to do a preliminary assessment on if the short term variability is too variable for your process or not.
The overall variability of the measurement system is a combination of both the short term and long term variability of your measurement system and can be represented by the equation:
Measurement tool variation (MS) = s2MS= s2Repeatability+ s2Reproducibility
To understand the long term variability or Reproducibility we need to take measurements over the varying conditions that the measurement system will be used. This may include multiple times of day, multiple days, multiple operators, etc. Be sure to capture your sources of variability in your data table and understand if your variables are crossed or nested. Below is an example data set.
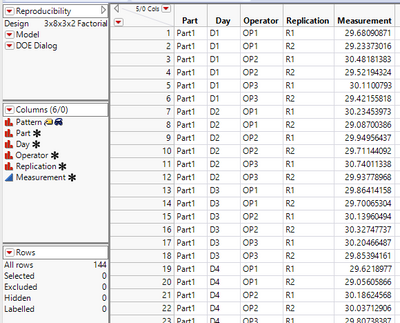
JMP under the Analyze > Quality and Process > Variability / Attribute Gauge Chart does all of your analysis for you.
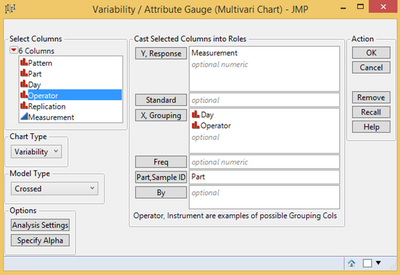
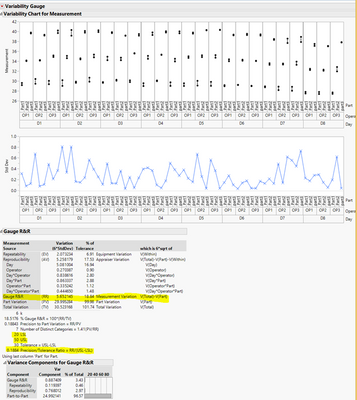
The output gives us estimates for our variability due to the different sources (useful for making improvements to the process) and an estimate of the Process to Tolerance ratio or P/T ratio. The P/T ratio is the amount of variation due to the measurement system to the length of the distance between the Specification Limits. P/T = 6sMS/(USL – LSL). The Guidelines for acceptable values of P/T are that it should be less than 30%.
Now that we have an acceptable measurement system we should track it over time via control chart methodologies to ensure it does not drift and become unacceptable.
So in conclusion with the help of JMP we can answer the question of Data! What is it good for? If our P/T ratio and bias are acceptable then our data is worthwhile and trustworthy. Otherwise the answer is absolutely nothing, and I am not going to say it again.