Visualization is crucial throughout the process of data analysis, from initial exploration through communication of important findings and insights. We discover patterns, uncover lurking relationships, and communicate visually.
With high-dimensional data — that is, data tables with many columns and rows — it can be challenging to create graphs that summarize the data enough to reveal overall patterns and also show the scale and extent of the variability in the data. Let’s consider some of the ways of balancing these demands.
To illustrate, we will look at some airline departure and arrival data for all US domestic flights in March, 2019. The data table is provided with my book Practical Data Analysis with JMP, 3rd Ed. The full data table contains 38 columns and over 632,000 rows. First, we consider arrival delays, reported in minutes. In Graph Builder, we drag the ArrDelay column to the Y drop zone and produce the default graphic, an outlier boxplot.
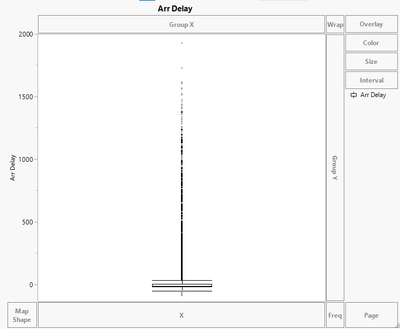
This plot shows a highly right-skewed distribution of delays, with a median near zero. Perhaps 50% of flights arrive ahead of schedule, and 75% have only modest delays. Checking the 5 Number summary box tells us more, and one might wonder about the maximum value of 1,928 minutes, or a 32-hour delay.

The upper whisker in an outlier boxplot is constructed at Q3 + 1.5*(Interquartile range), which in this case is 31.5 + 1.5(21) = 37.5. A large, but indeterminate number of observations lie above 37 minutes. If we use the Arrow tool to select the points above the whisker, we learn that there are nearly 54,000 outliers, but in this initial rendering of the graph, we see a dark line with some attenuated points at the higher values.
A bigger issue is that the outliers can visually dwarf the fact that the large majority of flights arrived within a few minutes of their scheduled times. If our priority is to communicate the center and shape of the distribution, we might prefer a histogram or violin plot. The latter is a Contour plot option. Here are the two graphs side by side.
These visualizations highlight the location and shape of the entire distribution, without overemphasizing the outlying points. On the other hand, they really don’t show any of the data points at all.
Of course, we can add every individual point to any of these plots by right-clicking on the graph and choosing Add > Points. Here’s the violin plot with points overlaid on the contour.
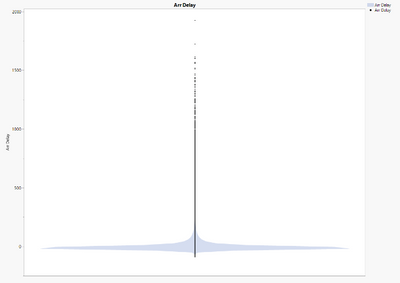
The problem is that there so much overplotting that most of the points are not visible. What to do?
Here are five simple tactics:
1. Divide and conquer. Making a set of small graphs may not be your first choice, but we start with this one because it helps to more clearly illustrate the other tips. If there is excessive overplotting in an unidimensional graph, include another dimension. For example, our data contains a categorical variable identifying the principal cause of the delay when a delay has occurred. In this dataset, only about one third of the flights were delayed, so we are seeing approximately 200,000 points rather than 600,000.
Using Main Cause as a grouping variable helps to identify the underlying dynamic reasons for variation in delays and it also more clearly displays the individual data values. For example, it’s immediately clear that most delays seem to be caused by the carriers and late equipment, that the longest delays are attributable to the carrier, and that Security delays are rare and brief.
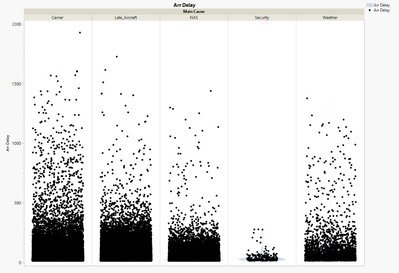
Note that in this rendering, the points are no longer aligned vertically, but have been jittered. That is, they have been repositioned slightly left and right to put white space between them. This bring us to the second tactic.
2. Add or adjust jitter. In this univariate graph, some points have been uniformly shifted to the left or right. The default jitter pattern spreads the points apart so that we can get a sense of how many flights there were at each value, but the points cover the contours.
In Graph Builder, the Jitter controls provide options.
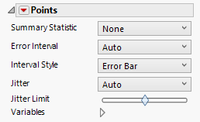
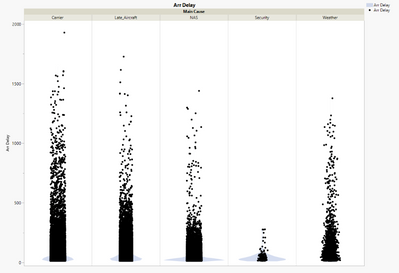

If, for example, we choose Random Normal jitter and reduce the Jitter Limit, we can reveal the violins again:
Reducing the jitter uncovers the violins, but increases overplotting. The next three tactics deal more directly to reduce the ink density. We can invoke these options by right-clicking on the black dot and Arr Delay in the legend in the upper right of the graph.
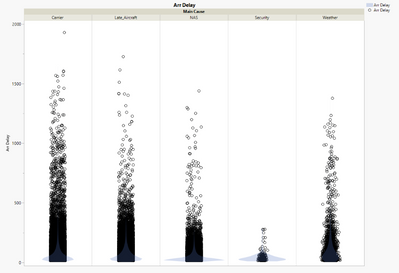
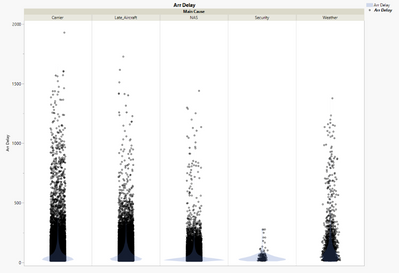
3. Use open markers. Choose Marker > and select the open circle rather than the solid dot. Now proximate points visibly overlap, rather than overlay one another.
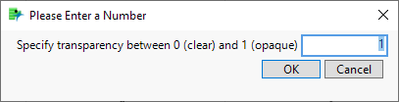
4. Use smaller markers. Even with open markers, the density of the points leads to considerable overplotting. Sometimes simply using smaller dots leaves more white space, allowing individual points to be more visible. We do this by right clicking on the black circle in the legend and choosing Marker Size > 1, Small.
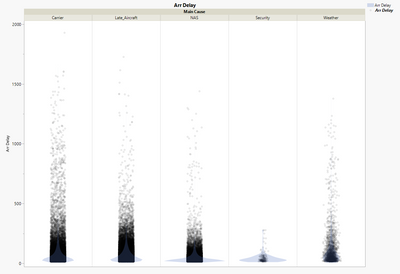
5. Use translucent markers. Open markers let us see through the center of a point, but the outline of the points is opaque. To reduce the visual confusion, we can make the points semi-transparent. Again, right-click the black circle in the legend, and choose Transparency… to open this dialog:
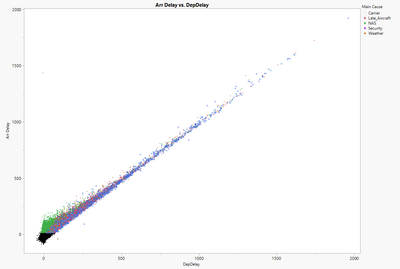
Enter a value between 0 and 1 as indicated. Here is the result of entering 0.2, for 20% marker density.
In this version, the very sparse uppermost points fade nearly into obscurity, but the abundance of points at lower values are accentuated.
These five basic tactics work equally well with bi- and multi-variate plots. Here, for example, is a scatterplot of Arrival Delay vs. Departure Delay, using small open markers. To add a third dimension, we color the points according to the main cause of the delays.
We now are plotting all 612,000 points, the large majority of which are quite near or below the origin. The mass of black points in the lower left are flights that arrived early or on time, and hence have no main cause of arrival delay. The comparative frequency and severity of the delays are visible by cause. More importantly, despite the thick density of the data, we can detect the overall patterns as well as the individual points.
You must be a registered user to add a comment. If you've already registered, sign in. Otherwise, register and sign in.