Have you ever been tasked with predicting who will cancel their services with your company? Or which patients will discontinue their medication? Or perhaps which individuals will be the early adopters of your new product? You might answer these business questions with the same method, carefully preparing data and building different models to compare and choose the best based on specific statistical criteria. Piece of cake, right? Maybe.
But what if you need to pinpoint the main contributing factor to a prediction for a single person? Some modeling algorithms do not easily answer this. Traditional regression models are great for answering this kind of question because there is an equation with coefficients for each parameter estimate. What about for tree-based algorithms or even more complicated models like neural networks? Explaining an individual prediction is more complex and often difficult to explain. And even though one of these more complex algorithms is more reliable for predicting an outcome in many cases, often, stakeholders scrap your hard work.
What if you could get the best of both worlds? The best performing model AND a way to easily explain the individual predicted probabilities to your stakeholders so that your work has a better chance to be used as a business tool. Meet Shapley Values—a new feature in JMP 17.
Shapley Values provide the solution we have all been looking for to solve this kind of situation. These values quantify the contribution of each factor in your model down to the row level. Using the average prediction as a baseline, each row of data can be broken down into a sum of probabilities by factor. This means you can say that Sean has a 79% chance of discontinuing his medication, and compared to the average patient, the primary reason for his probable discontinuation is his age.
Why is this such a big deal? Without Shapley Values, we could speak more generally about the driving factors for an outcome in the population of interest, but comparing across individuals with similar characteristics and understanding why the prediction differs between them is not so straightforward. In tree-based algorithms, the prediction formula is created based on individual leaves or trees. And let’s be honest, they can get quite messy. Shapley Values solves this issue by breaking down the prediction into individual contributions for each factor, which looks across the entire model instead of leaf by leaf, tree by tree, or node by node.
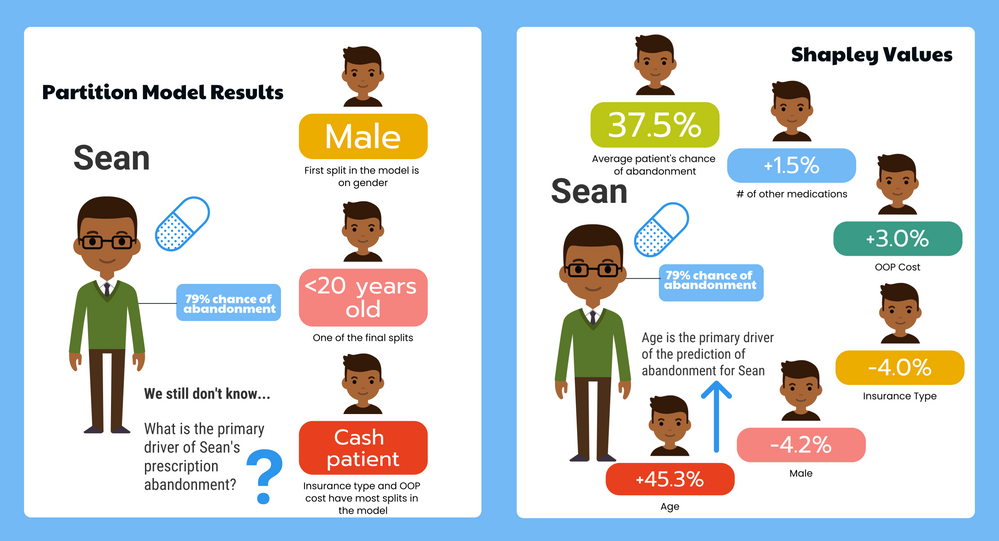
How can you get started exploring this new feature? It’s simple. Add the Profiler to your model results and select the option for Save Shapley Values. This will save individual columns to your data table for each factor/response level combination.
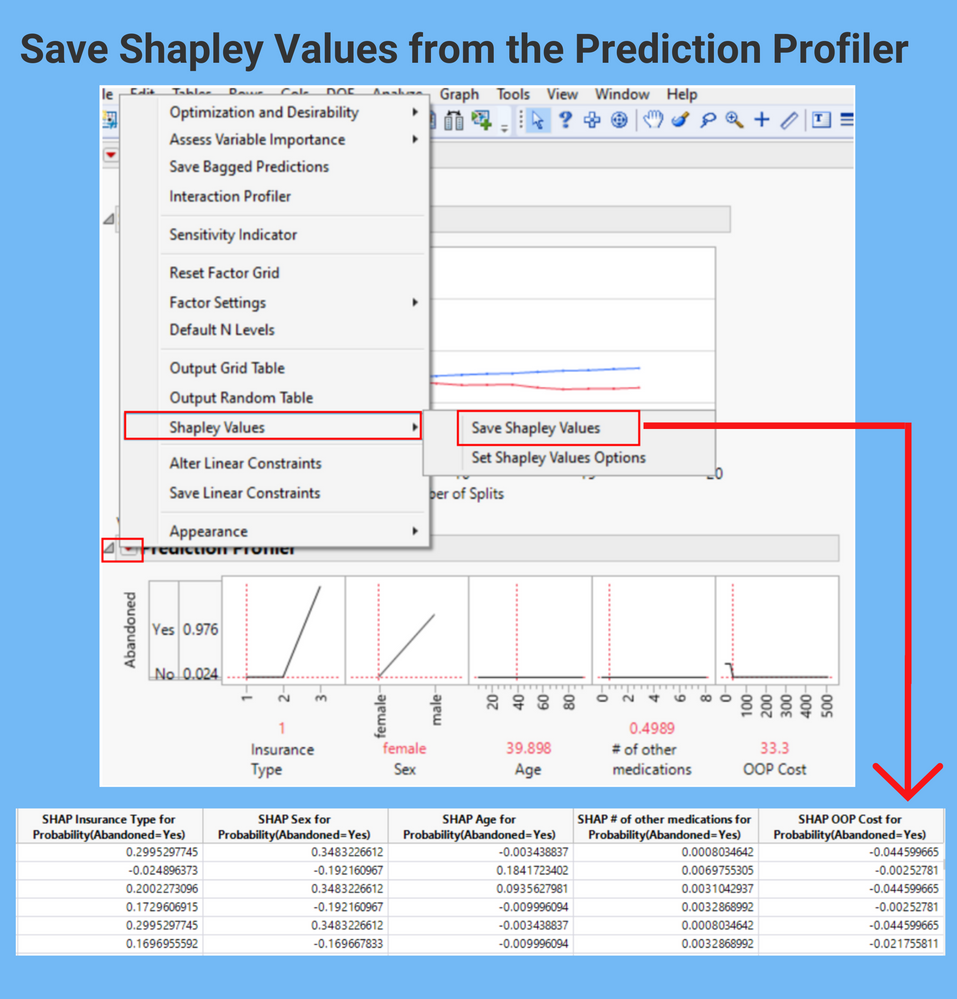
So the next time your stakeholders ask if you can pinpoint which patients are most likely to discontinue a medication based on a factor that can be influenced by a pharmacist’s actions or a marketing tactic, you can feel confident that you can say yes, no matter what type of model you’re creating.
Medication Discontinuation Shapley Values Example.jmp