JMP has a long track-record of adding useful methods to aid the work of scientists, engineers, researchers and analysts. What inspires the addition of these new methods? Often, there is a story behind the inspiration to include new methods, and in the case of A-optimal designs, it starts with the dissertation of a PhD student named Jon Stallings, who is now Assistant Professor of Statistics at North Carolina State University.
Our own Bradley Jones, Distinguished Research Fellow in the JMP division, served on the dissertation committee for Jon, whose dissertation, General Weighted Optimality of Designed Experiments, includes the motivating use case for adding A-optimality criteria to the custom design capabilities in JMP.
Jon shared his thoughts on the inclusion of A-optimality designs in JMP 14:
“Calling a design optimal can be very misleading for practitioners. It is important for practitioners to understand that a design is found to be optimal with respect to a summary measure of that design’s analysis performance. The summary comes with a loss of information about that design’s performance and practitioners should be aware of how the optimality criterion relates to their analysis goals. The D-criterion is by far the most popular criterion and works very well for response surface designs which place equal emphasis on estimating main effects and interactions. If one wanted to place greater emphasis on estimating some effects over others, the D-criterion would not be appropriate. Including weighted-A criteria in JMP 14 gives practitioners more flexibility in design selection and allows them to tailor a design to their specific goals. JMP’s powerful Custom Design platform allows practitioners to quickly generate and compare competing designs under these criteria and become more involved in design selection.”
As Brad explains, “A-optimal and D-optimal designs are similar, but A-optimal designs allow for putting different emphasis on groups of parameters through weighting and the optimality criteria is easy to understand. Depending on the situation, you may want to emphasize main effects more, or second-order effects and weighted A-optimal designs give you the flexibility to do that.”
In this example comparing A-optimal and D-optimal designs, the A-optimal design has more desirable properties. The D-optimal design has messier correlation structures and the design diagnostics for the A-optimal design are much better.
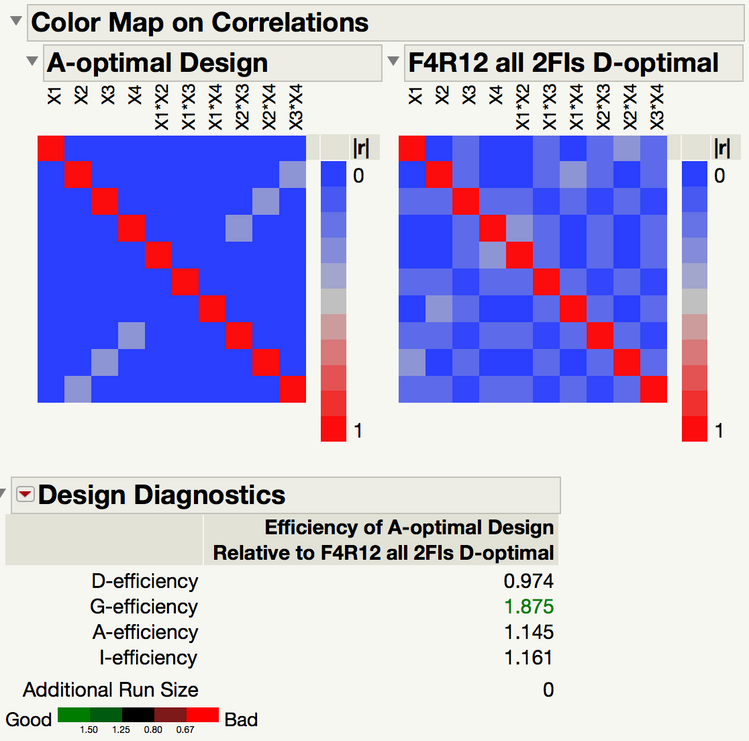
This is a simple example, but it conveys the potential for A-optimal designs to give you flexibility to emphasize some effects over others and create a design that can better meet your needs.
A-optimality criteria is a relatively new research area in design of experiments, and we are pleased to include it in JMP 14. You can expect more detailed blog posts on new DOE features later in the year.
You must be a registered user to add a comment. If you've already registered, sign in. Otherwise, register and sign in.