Megan Tengan is a senior at the University of Hawaiʻi at Mānoa in Honolulu where she is pursuing a double major in political science and philosophy. As Treasurer and Secretary of the Mānoa Pre-Law Association, Megan plans to attend law school following her graduation in December 2022.
She is currently working as a congressional intern at the office of US Senator Mazie K. Hirono in Washington, D.C. During the fall semester of 2021, Megan served as Teaching Assistant for Dr. Lawrence Nitz’s 300-level political science course, Political Inquiry and Analysis. Together, they developed a series of lesson plans and assignments based on research questions that could be explored using open data sets.
Megan also supports independent research undertaken by Nitz, investigating the extent to which tweets related to Covid in Hawai’i are driven by fluctuations in the rate of new infections and local news and opinion reporting. I spoke with Megan to ask her about her experience learning and working with JMP in a social science setting.
Megan Tengan, undergraduate student at the University of Hawaiʻi at Mānoa
Meg: So tell me, Megan, how did you become interested in statistics? Quantitative approaches have always been an important branch of research methods in political science, but many poli sci majors – myself included! – don’t get much undergraduate exposure to statistics.
Megan: I had never worked with numbers in political science [prior to TA-ing for Professor Nitz], so statistics was new to me! I learned alongside my students. That said, it was a really cool experience to play around with the numbers and text analysis, because we did look at a lot of social statistics like race and health data – and we were, of course, also looking at Covid. [Having experience with statistics] is a great resume builder because I think the future is data analysis. There will always be that need for quantitative inquiry.
Meg: Was there a turning point for you, where you realized how important data analysis skills would be? Or when you saw the importance of data as it played out in a social science use case?
Megan: In class, we looked at tweets about critical race theory and used text analysis to look at popular opinion. Obviously, modern civil rights movements have been gaining momentum recently, and as a Gen Z-er, that's something on my social media timeline a lot. I didn't know that you could analyze text in the way that we did in class, and JMP gave us the means to analyze not just common words and phrases, but the tone of the text. I guess I just didn't realize that there was technology that could do that – that could search through social media data.
Meg: Now that people are living their lives online, there’s an incredible amount of open data that anyone can freely access. And I’d imagine social scientists are just at the tip of the iceberg when it comes to using that data for research. How did you go about building a library of course assignments around data from Twitter, and what kinds of skills did you want students to learn?
Megan: Professor Nitz compiled tweets related to the state of Hawai’i and current topics like Covid or critical race theory. Then we would set the students up with a few articles about the subject: what it is and what [the controversy is]. Then we would give them the data set. The assignment was to use charts and graphs in JMP to analyze and understand [data trends], and then apply what you see to the real world. We did text analysis and changed some of the settings to get rid of [the noise]. For example on Twitter, you see a lot of “http,” which is just a hyperlink, and you don't need that. We learned a lot about throwing out data that is just irrelevant and clouds your analysis. Once we’d taken out all the text we didn’t need, we would ask questions like, “Are the cluster of top words similar?” or “Do the two bodies of text fall together the same way?” Students would look at two separate sets of tweets and compare. [When preparing the data sets] we tried to make it so that one was a little bit more passionate – for example, clearly outraged about critical race theory – while the other used positive words but in an ironic or sarcastic tone.
We wanted to throw the students off a little bit because JMP could take irony as a positive sentiment when in reality, it's not. They had to learn to read the chart a different way…and run a sentiment analysis: Are the [two data sets] similar in positive and negative sentiment content? We emphasized the importance of cleaning up terms designated as stop words and asked students to explain the differences between the clusters they were observing. JMP would cluster different themes – one would be about education, another one would be about politicians, another about racism, another about discrimination, and so on. JMP categorized the data like this, and my students were able to tell me what they saw in each cluster.
Meg: Twitter is a real minefield for sarcasm! How does a statistical tool figure out intention?
Megan: Right. So someone might tweet something like, “The fact that racism is still prevalent in America is just amazing,” even though they don't mean that it's fantastic; they mean that it's astonishing or shocking that racism is still a problem. And you have to consider the context around a word, though obviously you're not going to read every single word of the thousands of tweets we pulled. For example, you might think that people are saying critical race theory is amazing or astonishing, even though that's not what people who are tweeting actually mean. So we asked students to use JMP to look at how sentiment was dispersed and separate the ironic tone from the literal.
Meg: No one could argue this isn’t interesting…but what’s the application? What are you learning?
Megan: Well, two things. For the students, Political Inquiry and Analysis is a required class, and I think ultimately, data analysis is a good skill to learn. In fact, one of our guest speakers offered a few people jobs as statistical analysis interns at the end of the semester! The more well-versed you were in JMP – and the more time you put into it – the higher the pay was. So we had a couple students try it out, and I'm hoping they've made something of the experience. And second, my professor and I are considering presenting our Covid tweet research at this year's JMP conference.
Meg: I hope you do! This topic would make for a great Discovery Summit talk! So tell me, what are some of the biggest data challenges you face when it comes to using open data to explore public opinion?
Megan: For one thing, JMP itself is very easy to use. I like the user interface. But with that comes the responsibility of reading the data correctly. I think it's trial and error to find what you want, and you need to have a good grasp of what you're learning so that you can see patterns when you think there aren't any. Even if the pattern is that there isn't a pattern, that's a pattern! That can be difficult to understand at first. JMP gives you so much information, which can be like a double-edged sword because it's great to [be able to access so much data], but you also have to distinguish what you're looking for. For example, I can apply that conundrum to our current project because so far, we haven’t found the causal relationship that we were expecting between rising Covid infections and related tweets – that is, tweets pertaining to the topics of Hawai’i, Covid and our governor, David Ige.
Meg: What were you expecting to find?
Megan: Our main questions were: Are these tweets about Covid in Hawai’i driven by recent infection rates? In other words, once the state reports that we have 700 new cases, does that cause more people to tweet about Covid? And second, what is the content of their tweets? Is the content of a tweet related to the news being broadcast about Covid in Hawai’i? At first, it was like “this deadly disease,” and then it turned into “Should we get the vaccine?” And then “Should we get the booster?” Now it's mandates, no mandates, lifting mandates, etc. The news can decide what they report on, and we want to know whether that affects what average citizens choose to tweet about. Again, we have to highlight the sarcasm. People might say something like, “Brilliant, this tourist got Covid,” or “It’s amazing when those who enforce rules refuse to follow them.” Of course, they don't actually mean those statements literally.
Meg: It may sound like a simple question, but what's the value in establishing these correlations and relationships? To gauge public sentiment? To inform policy?
Megan: I think you said it perfectly – evaluating public sentiment – that's really what we are doing with these assignments. We want to understand how public opinion changes. The novelty of a pandemic within this modern century is, I think, what made it so interesting to research because this hasn't happened in a hundred years. We didn’t have JMP or anything like it back then, right? And especially now with social media – and Twitter in particular – we have an unprecedented platform where the public can express their concerns and opinions. We have more material than ever to analyze in JMP!
Meg: As a teaching assistant, what pedagogical approaches have you observed work best when it comes to teaching statistics to undergraduate, non-statistics majors?
Megan: I think the important thing is to be able to draw relationships within the data sets that we choose to give students. We spent a couple of weeks covering the likelihood of the relationships – the gamma or whatnot – and that was a little bit hard, maybe, for everybody to grasp, especially had we chosen different factors than we did in the demos. But I think the real mathematical part of it was the kicker, because you're right – these students aren't studying math. My highest math was like pre-calc, and I hated it!
Once we realize that obviously not everybody is interested or particularly gifted at the math side of things, we can focus more on pulling out relationships. For example, we did a survey of the extent to which citizens of a state trust their home state politicians versus the President, and explored whether the local drives the national, or vice versa. Or we might ask: Does having a higher degree – or your parents having a higher degree – have anything to do with how much debt you have?
Meg: By understanding the application and the relationships between factors, that helped people stay engaged in learning statistical methods.
Megan: Definitely. And especially as social science majors! We always want to find out the why – even in everyday politics. Why is this policy not making it through? Why are these people against it? It's always about what’s hidden behind the argument, so it was almost intuitive for my students to find out if there was a why. And JMP really helped visualize that for them, because they could see trends and then even look at the numbers – even if they didn't want to – and then see if there was a relationship between whatever factors we were looking at.
Meg: So you’ve got law school in your future, Megan? Do you think you’ll be able to put these quantitative skills to use?
Megan: I think that, depending on what field of law I go into, I could use and apply these quantitative skills that I've learned through JMP. For example, maybe if I become an environmental lawyer, and I’m looking at GHG emissions driving global warming or whatnot. But even if data science isn’t at the center of my career, it was, and still is, an interesting project to have on the side. And like I said before, data drives the conversation. I obviously like to have an evidence-based opinion, and that's what JMP is able to provide me with.
Meg: So my last question is: What advice would you give to either students or novice JMP users in general?
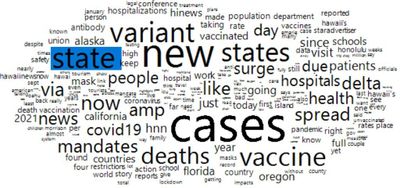
Megan: Definitely use the resources that JMP has to offer for beginners. Even if you’re coming in with advanced skills, you can still learn through the videos, too. I learned a lot of what I knew through those beginner guides. If you want to do something specific, JMP most likely has a way for you to do it. And I recommend using the sample sets that JMP has. Those were really helpful because you can't always find a perfectly formatted spreadsheet to use in JMP. That offering was really great. And lastly, explore. Click all the buttons and figure out what everything does. Click all the dropdown menus. That was definitely how I got myself a little bit better versed in what I was doing!
You must be a registered user to add a comment. If you've already registered, sign in. Otherwise, register and sign in.