Original session date: 7 April 2022
Topics covered: XGBoost, Hyperparameters, machine learning
Speaker: Russ Wolfinger (@russ_wolfinger), Distinguished Research Fellow
XGBoost is a (relatively) new machine learning protocol that is only beginning to be deployed in the Upstream Energy and Production industry. This decision tree ensemble model is taking the world by storm (well, ok, that might be a bit of hyperbole, but come on!), winning Kaggle competitions and every so slowly, making its way into oil and gas. Russ Wolfinger, a SAS Distinguished Research Fellow, Kaggle Grandmaster, and longtime lead in the JMP Life Sciences division has created and refined a XGBoost add-in, which he demonstrates in the video below.
Why XGBoost and how is it different? XGBoost deploys a a gradient boosted decision tree algorithm. Through gradient boosting, one is able to use layers of models to correct the error made by the previous model until there is no further improvement can be done or a stopping criteria such as model performance metrics is used as threshold" (Medium). Deployed in JMP, this provides the user the opportunity to a) apply up to three orthogonal folds for model validation, b) b) autotuning, which deploys JMP-patented DOE technology to rapidly iterate to optimal hyperparameters, and c) JMP's prediction profiler to observe and interact with the model to better understand how individual factors can be altered to optimize the response of interest.
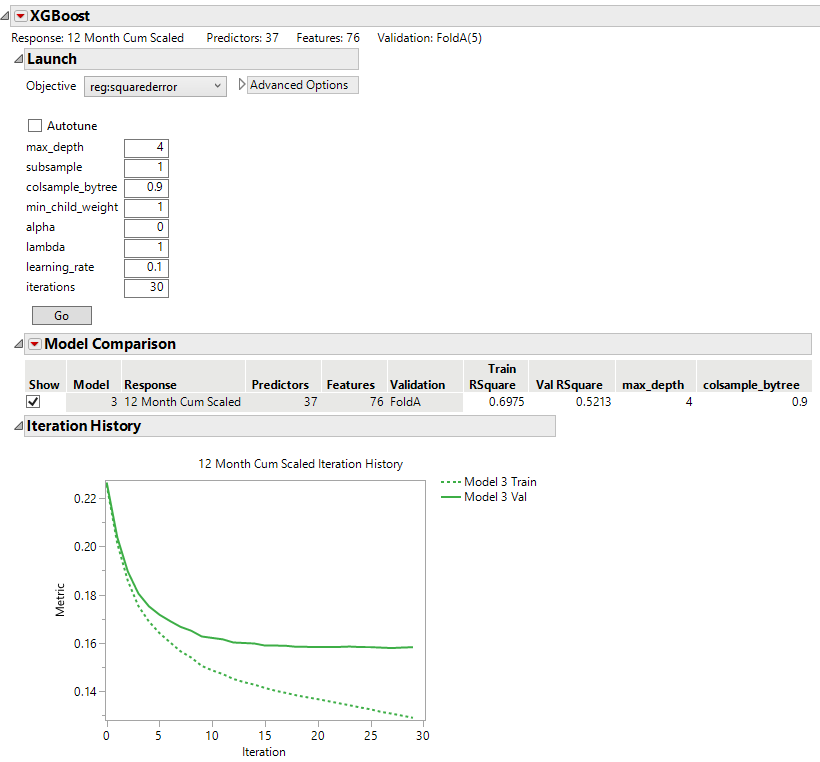
Figure 1. Model hyperparameters and fit statistics evaluating a series of location, geologic and completion factors on 12 month cumulative production in the Barnett Shale, Texas, USA.
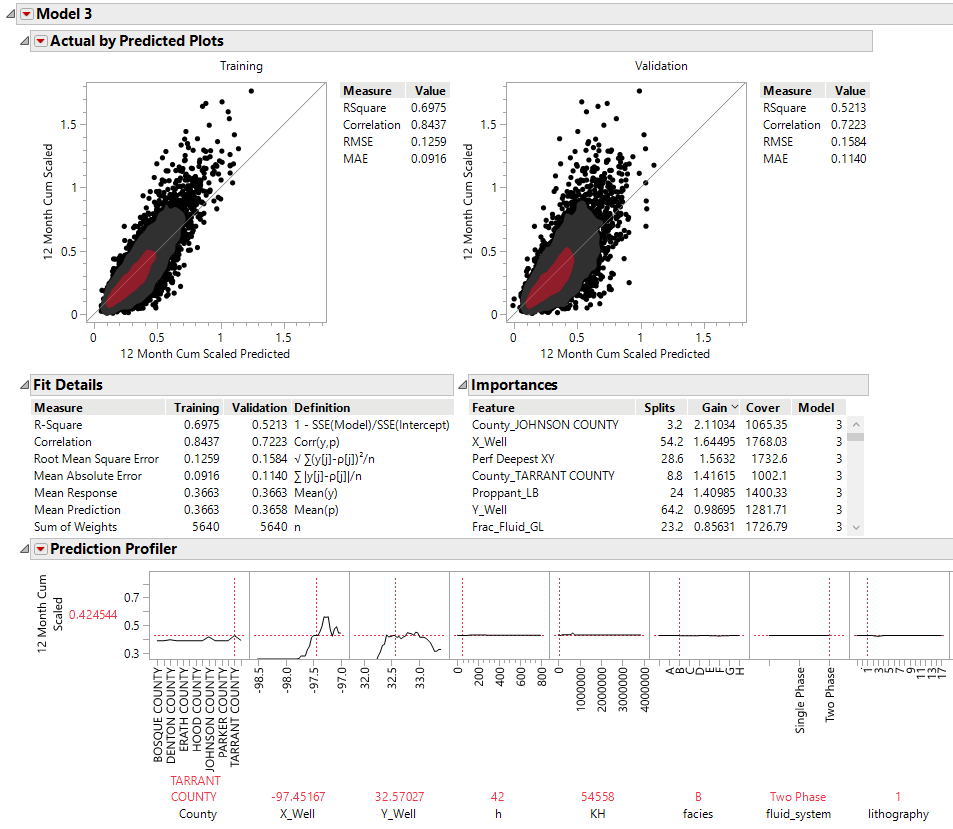
Figure 2. Predicted vs. actual and prediction profiler of an XGBoost model evaluating a series of location, geologic and completion factors on 12 month cumulative production in the Barnett Shale, Texas, USA.