Original session date: 7 October 2021
Topics covered: Graph Builder, clustering, support vector machines
Methodology is inspired by SPE Paper 1961100 - Machine Learning of Spatially Varying Decline Curves for the Duvernay Formation
It is understood that heterogeneity exists at all scales in the natural environment. Whether at the pore scale or basin scale, rarely can a geologist or petroleum engineer replicate their assumptions or their drill plan from well to well. Finding tools that allow one to rapidly group together like wells for the purposes of model building is a key tool used by many in the industry. In this session of JMP in the Upstream, Systems Engineer Nick Shelton, highlights a work flow to use geologic and completion parameters to cluster wells then use those clusters as grouping categories to predict decline curves using the machine learning tool, support vector machines. He presents an elegant and simple point-and-click workflow to achieve a much more robust predictive model to then make analytical-informed decisions about best locations to place wells.
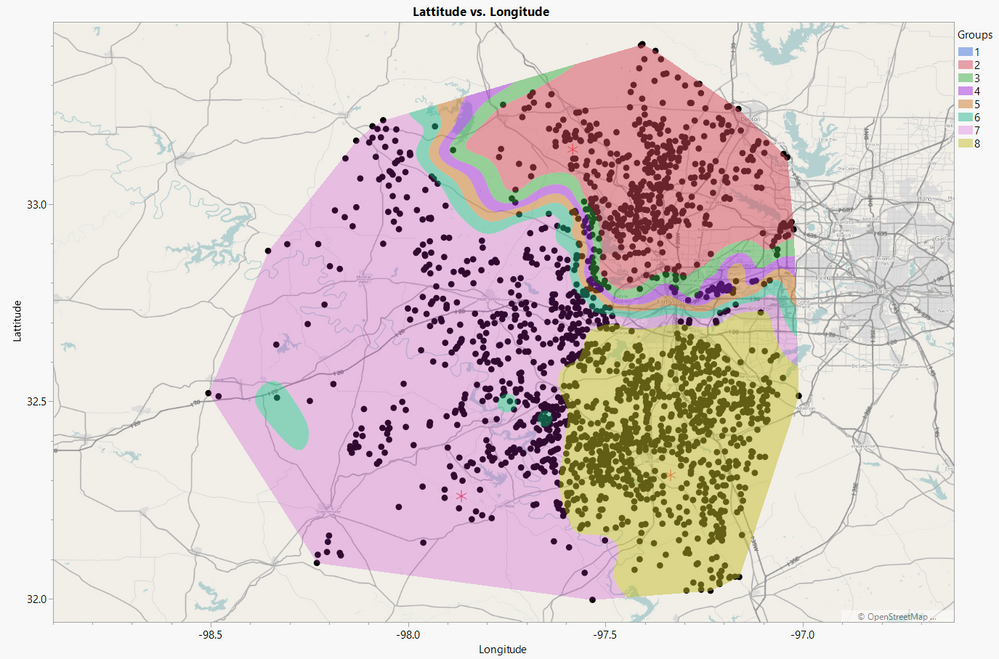
Figure 1. Predicting oil production in the Barnett Shale from decline curves using clustering and support vector machines in north central Texas.
ML_DCA (SVM is Pro only).jmp