Original session date: 2 September 2021
Topics covered: predictive modeling, machine learning, neural networks, XGBoost
Data source: https://www.netl.doe.gov/node/6865
In this episode of JMP in the Upstream, Peter Polito (Sr. Systems Engineer/Geologist) walks us through a methodology for predicting downhole hydrocarbon saturation using four modeling methods: standard least squares, generalized regression, neural networks, and XGBoost. He uses the hydrocarbon saturations in the upper ~1/3 of the well to construct each of the four models in order to predict saturations in the lower ~2/3. Finding that the two machine learning methods--neural networks and XG Boost are better predictors.
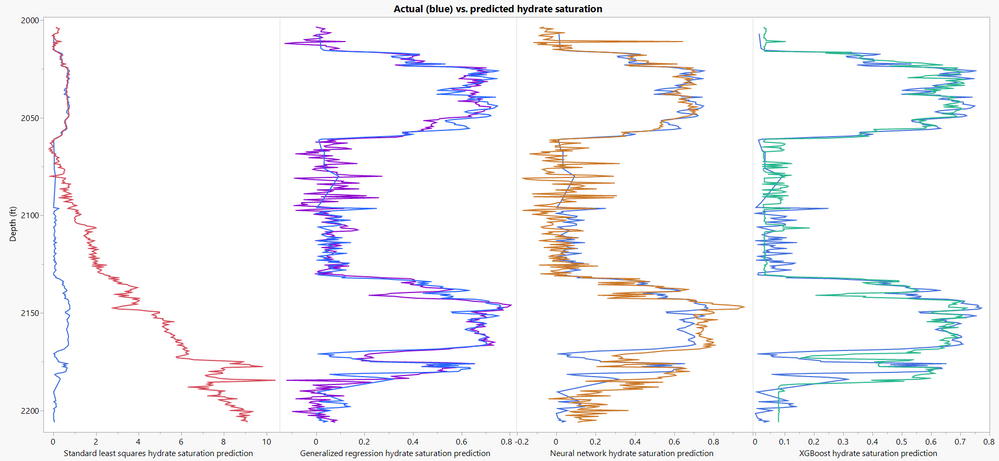
Success (or lack thereof) in predicting downhole hydrocarbon saturation using standard least squares, generalized regression, neural networks, and XGBoost. Models were constructed using hydrocarbon saturations shallower than 2050 ft to predict saturations deeper than 2050 feet.