This June, JMP and Wiley presented a two-part webinar series, Conquering the "Do More with Less" Paradox with JMP. The series discusses ways to overcome the new reality faced by everyone in today's workplace; becoming more efficient, improving processes, and driving growth for their organizations... without growing the budget. The series gives attendees real-world tips, tricks, and best practices to help them see data more clearly, to interpret and share discoveries more easily, and to make insight-driven improvements that help them "do more with less."
The first talk was offered in June and again on October 31, 2023. Decoding Python: Shorten the time from discovery to model deployment using JMP Pro, shows attendees how to perform time series forecasting using Python and JMP Pro, featuring the machine learning method XGBoost. Python is the most popular programming language in data science and machine learning; it is also a great complement to the powerful graphical and analytical capabilities of JMP Pro. During the webinar, Russ Wolfinger, Director of Scientific Discovery and Genomics at JMP, and Kemal Oflus, JMP Principal Systems Engineer, show attendees how to:
In addition, Russ and Kemal also share with attendees the following Jupyter notebook attachment: final_combined_data.csv.zip) and JMP table (attachment: PJME_hourly.jmp) and a link to the XGBoost add-in.
For more advanced discussion of this topic, refer to Russ’ paper with David Lander on their approach to winning the Kaggle M5 Uncertainty competition.
The second talk, Analyzing Curve Data with JMP, shows attendees how to use JMP to work with data represented by a curve, a challenge many scientists and engineers face. It also shows viewers how to use curves in design of experiments and modeling so they can better understand how input variables affect the shape of curves. The talk, led by Senior Systems Engineer Andrea Coombs, shows attendees how to analyze both curves described by a formula (attachment: Fit Curve DOE.jmp) and curves that needed to be described with a flexible fit (attachment: Functional DOE.jmp).
To access the on-demand recording of either talk, register using the links below:
Analyzing Curve Data with JMP
Decoding Python: Shorten the time from discovery to model deployment.
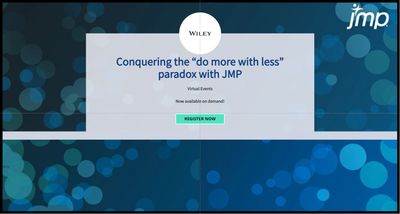
final_combined_data.csv.zip
You must be a registered user to add a comment. If you've already registered, sign in. Otherwise, register and sign in.