Editor's note: This post is by @ronkenett, Anat Reiner-Benaim and @david_s of the KPA Group. It is part of a series of blog posts called The QbD Column. For more information on the authors, click the authors' community names above.
Ron Kenett
David Steinberg
Anat Reiner-Benaim
The first two posts in this series described the principles and methods of Quality by Design (QbD) in the pharmaceutical industry. The focus now shifts to the role of experimental design in QbD.
Quality by Design in the pharmaceutical industry is a systematic approach to development of drug products and drug manufacturing processes. Under QbD, statistically designed experiments are used to efficiently and effectively investigate how process and product factors affect critical quality attributes. They lead to determination of a “design space,” a collection of production conditions that are demonstrated to provide a quality product. A company making a QbD filing gets regulatory relief, meaning that changes in set-up conditions within the design space are allowed without needing pre-approval. The statement often heard is that the QbD design space is about moving from “tell and do” to “do and tell.” As long as changes are within the design space, regulatory agencies need only be informed about them.
Study of nanosuspensions: An example
In this post, we look at considerations in planning a statistically designed experiment, collecting the data, carrying out the statistical analysis, and drawing practical conclusions in the QbD context. The example is based on Verma et al. (2013).
The goal of the experiment is to explore the process of preparing nanosuspensions, a popular formulation for water-insoluble drugs. Nanosuspensions involve colloidal dispersions of discrete drug particles, which are stabilized with polymers and/or surfactants, such as DOWFAX. Nanosuspensions achieve improved bioavailability by using small particles, which increases the dissolution rate for drugs with poor solubility. After beginning with larger particles, the process then uses milling to reduce their size. The current study examines the use of microfluidization at the milling stage.
What were the CQAs?
Verma et al. studied several critical quality attributes (CQAs) in the experiment: the mean size of the particles at the end of the milling stage and after four weeks of storage; and the zeta potential, which serves as an indicator of stability by measuring the electrostatic or charge repulsion between particles. Verma et al. provided additional data that track the particle size distribution throughout the milling process, as well as both particle size and zeta potential during storage. We will focus here on the outcomes obtained at the end of milling and storage. In a subsequent post, we will describe an analysis that takes into account the trajectories of these outcomes over time.
Although Verma et al. did not state specific target values for these CQAs, they wrote that mean particle size should be as small as possible and zeta potentials should be as far from 0 as possible (indicating greater stability).
What were the process factors?
The team chose to include five different factors in the study. The factors are listed in the table below, along with their experimental settings. The first factor, indomethacin, is the drug that was prepared by nanosuspension. As noted, there were three different concentrations of the drug.
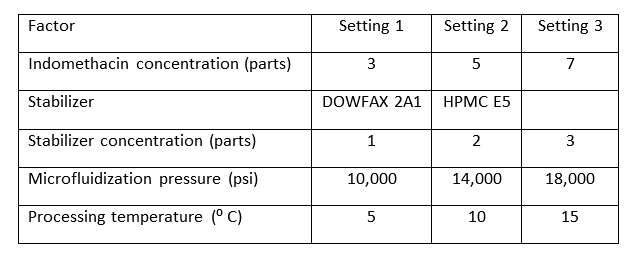
What was the experimental design?
The experiment was planned as a two-level fractional factorial with center points. The fractional factorial was a 25-1 design that used the extreme levels of each of the quantitative factors. Six center points were added. The 25-1 design permits estimation of all the main effects and all the two-factor interactions.
The center points make several useful contributions to the design. First, they can be used to compute estimates of experimental variation that do not depend on choosing some model to fit to the data. Second, they provide some check as to whether there is a need for pure quadratic terms in relating the CQAs to the factors. If so, then they help us to remove bias from our estimates near the middle of the design region. And if not, then they lead to better variance properties for our predictions in the center of the region. Figure 1 shows the JMP Variance Profiler for the design, assuming only main effects are important, and taking the center point for the numerical factors as the point of reference. If the six center points are deleted, the variance at the reference location increases by almost 40%, from 0.091σ2 to 0.125σ2. The design evaluation tool is very helpful here in deciding how many center points to include.
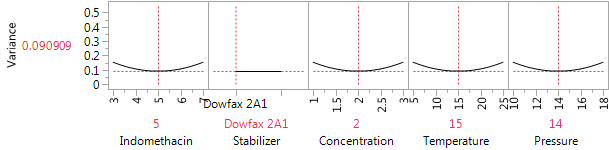
Figure 1: The Variance Profiler for the 22-run design with six center points.

Figure 2: Choosing a design with the Screening Design platform.
How can we generate the design?
Textbook examples typically show center points when all factors are numerical and can be set to an intermediate level. In this experiment, four factors are numerical, but one, the choice of stabilizer, is categorical. The natural choice in this setting is to use each stabilizer for half of the center points, which was the design we used here.
It is easy to construct the design in JMP with the Screening Design platform in DOE. Enter the four numerical factors with their extreme settings, and enter the stabilizer as a two-level qualitative factor. Choose the 16-run fractional factorial without blocking (which was not needed in this study). Then indicate that you want six center points. Figure 2 shows the final screen in this process. Clicking on Make Table produces the design that we used in this study.
What did the analysis find?
Our analysis includes all the main effects and two-factor interactions. How should we handle the center points? There are two possibilities. If we ignore the center points in specifying the model, JMP will recognize them and will include a Lack of Fit summary in the output. The summary compares the average at the center points to the average of the factorial points and also computes the “pure error” variance estimate from replicates at the center. For the Verma et al. experiment, there are really two center points, one for each stabilizer, and hence two center point averages. Consequently, the six center points provide 2 df for lack-of-fit (one for each stabilizer) and 4 df for pure error.
In the second approach, we begin by generating a new “center point” column in the data sheet that is equal to 1 for the center points and 0 for the factorial points. Including a main effect for the center point column and its interaction with stabilizer picks up the 2 df for lack of fit. The model now has exactly 4 df for error, corresponding to the pure error. The advantage of this method is that the p-values for the factorial effects will now be computed using the pure error variance estimate.
Figures 3, 4 and 5 show the sorted effects for the factors and their interactions. The effects are given in terms of regression coefficients. Because the factors were entered here with real levels, not generically coded to -1 and +1, the size of the coefficients depends not just on the strength of the factor, but also on the spread of the levels. The spread also affects the standard error of the coefficients. Consequently, the JMP Factor summary sorts and graphically displays the strength of the effects in terms of their t-statistics.

Figure 3: Sorted parameter estimates for the mean particle size at the end of the milling phase.
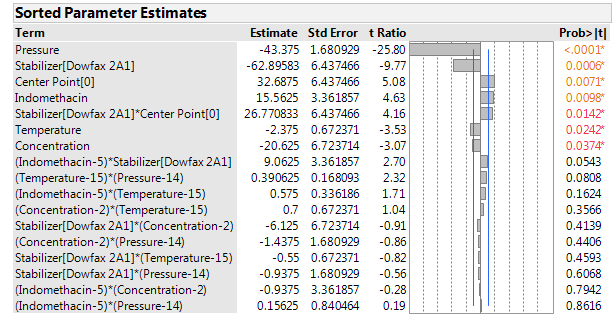
Figure 4: Sorted parameter estimates for the mean particle size at the end of the storage phase.

Figure 5: Sorted parameter estimates for the zeta potential.
What can we conclude about particle size?
The mean particle size ranged from below 500 to almost 1,000 nanometers after milling. Typically, there was a modest increase in mean size after storage. Figure 6 shows the high correlation between the mean particle sizes before and after storage. Not surprisingly, the same factors show up as dominant in analyzing both of these responses. The strongest factor is processing pressure, with substantially lower mean particle size at high pressure.
The stabilizer is also important, with DOWFAX resulting in smaller particle sizes. Increasing the concentration of the stabilizer has a modest reduction effect, which is statistically significant after milling but at the border of significance (p=0.035) after storage. The concentration of indomethacin and the processing temperature have relatively small effects. Both reach statistical significance after milling, but they are very small compared to the effects of pressure and stabilizer.
The analysis clearly shows that increasing pressure and using the DOWFAX stabilizer are the keys to achieving small particle size. Increasing the concentration of the stabilizer reduced particle size after milling, but that effect was small after storage. Lower concentrations of indomethacin and higher temperatures also led to smaller particle sizes, but these effects were small by comparison to those of pressure and stabilizer.
The center point average is significantly higher than that at the factorial points, suggesting some nonlinearity. A first guess would be that pressure is the factor with the nonlinear effect, as it is clearly the strongest numerical factor. There are also significant center point by stabilizer interactions. Figure 7 shows a plot of mean particle size after milling against pressure, with points coded by stabilizer. The results with HPMC appear quite linear, but those for DOWFAX show clear curvature. This suggests that, with the DOWFAX stabilizer, the benefits from increasing pressure are not as pronounced once the pressure exceeds 14,000 psi.

Figure 6: Plot of mean particle size after 28 days of storage vs. mean particle size after 90 minutes of milling, before storage.

Figure 7: Plot of mean particle size after 90 minutes of milling vs. pressure, coded for stabilizer. Pressure is given in units of 1,000 psi.
What can we conclude about the zeta potential?
The zeta potentials ranged from -8 to -76. As all are negative, factor settings that lead to “more strongly negative” potentials are desired. The dominant factor affecting the zeta potential was the stabilizer, with DOWFAX leading to results lower, on average, by about 53 units than HPMC. There was also a modest effect of the stabilizer concentration. The effect of the concentration was limited to the formulations with DOWFAX, with only a small effect using HPMC. It is easy to see this change in the effect of the concentration using the Prediction profiler. Figure 8 shows the profiler for the “preferred conditions”: DOWFAX, high stabilizer concentration and high pressure, leaving indomethacin and temperature at their center levels. Sliding the vertical bar for stabilizer to the right end of the plot (for HPMC) shows that the effect of concentration on the zeta potential becomes much weaker.

Figure 8: Prediction Profiler at the preferred conditions for achieving small particle size and large absolute zeta potentials.
Recommendations for design space
The experiment showed that small mean particle size can be achieved by using high pressure and the DOWFAX stabilizer. There is a modest effect of stabilizer concentration, with higher concentrations giving smaller particle size. The primary effect on the zeta potential is the stabilizer; again, DOWFAX produced better results. With specification limits for these outcomes, the design space can then be determined much as we did in the second post of this series. The small effect of temperature implies that it can be set largely by other criteria, such as ease of operation or reduced cost, without harmful effects on the CQAs. The small effect of the indomethacin concentration implies that the findings are broadly applicable to the production of this drug.
Coming attractions
In the next post, we will show how to achieve a robust design set up, within a design space, using a stochastic emulator.
Reference
Verma, S., Lan, Y., Gokhale, R. and Burgess, D.J. (2009). Quality by design approach to understand the process of nanosuspension preparation. International Journal of Pharmaceutics, 377, 185-198.
You must be a registered user to add a comment. If you've already registered, sign in. Otherwise, register and sign in.