Three years into the COVID-19 pandemic, we have learned a few things (and we have learned there’s a lot more we still don’t know). Data challenges to effectively track and forecast infectious disease are considerable—but not insurmountable. We have more data to consider in making other decisions affecting health—but what useful information may be derived from -omics data (e.g., genomics, proteomics)?
We are grateful to have award-winning, world-renowned statisticians, father and son, Drs. Robert and Ryan Tibshirani, bringing their innovative problem-solving skills to bear to realize considerable progress in these areas. And we appreciate them sharing more about their current research interests on the last 2022 episode of Statistically Speaking.
Both have many accolades for contributing powerful new statistical methods, and for their work in so many areas, most notably biology, medicine, and public health. Ryan Tibshirani, Professor of Statistics at the University of California, Berkeley, is the recent recipient of the 2022 Mortimer Spiegelman Award, which is presented to a health statistician who’s made outstanding contributions to statistical methodology and its applications in public health. Rob Tibshirani, Professor of Biomedical Data Science and Statistics at Stanford University has many awards, the latest of which is the International Statistical Institute’s Founders of Statistics Prize for Contemporary Research Contributions in recognition of his 1996 paper on the LASSO, considered a cornerstone of statistics and data science.
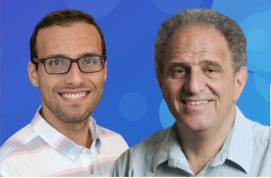
Their talks inspired several questions, a few of which went unanswered during the Q&A, but Rob has kindly provided answers here.
Was the Lasso something that was inspired by another discipline like applied math or was it more of a next step after ridge regression? Was its variable selection property a surprise or did you see the geometry first and then made the connection that it could be used for variable selection?
Rob: The lasso idea came directly from Leo Breiman's 1995 paper on the "Garote". (Leo was the inventor of bagging and Random forests; one of my heroes.) There he proposed computing the least squares estimates and then shrinking them via non-negative constants with a bound. This didn’t work for p>n (the case with more predictors than observations), so I just eliminated the middle man (LS).
What about causality in the analysis of observational data? People often attribute a causal explanation to the results of a variable selection exercise. Are there principled ways the Lasso can be used to make causal statements?
Rob: Re causal inference and the Lasso. Difficult to do in general. But with observational (non-randomized) data—you can use propensity scores to estimate a causal treatment effect, with confounding vars modelled via the lasso: see Quasi-oracle estimation of heterogeneous treatment effects- R estimation—X. Nie, S. Wager, Biometrika 2021. Full disclosure—the second author is my son-in-law!! But it really is a nice idea, and one that I have used.
Rob’s talk also included a brilliant animation he made with one of his graduate students, Daisy Ding.
We thank Rob and Ryan for taking the time to share some of their vast knowledge, wisdom, and innovations in statistical problem-solving. We hope you will watch the on-demand version of this episode of Statistically Speaking to see some of Tibshiranis’ fascinating current research interests and applications benefitting us all.
You must be a registered user to add a comment. If you've already registered, sign in. Otherwise, register and sign in.