While our recent mini-series, Smarter Innovation with Design of Experiments, has concluded, interest in the topics we covered has not waned. In particular, people want to learn more about:
- Reflections on the past, present, and future of DOE
- Easy DOE
- And predictive DOE.
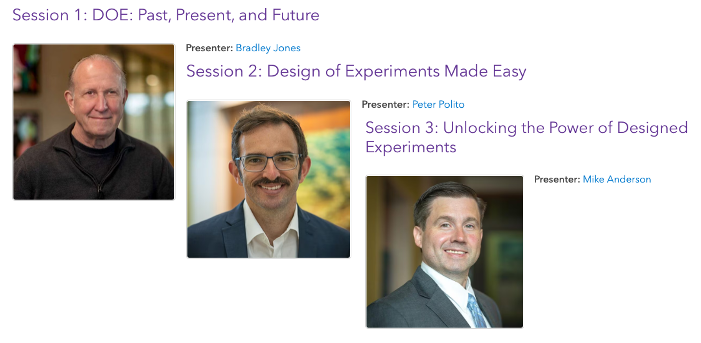
We had so many questions, we didn’t have time to answer them in the livestream events, so our brilliant subject-matter experts have agreed to answer them in this blog post in the order of each session.
Session 1 was unique in that it was a changing-of-the-guard interview with now-retired Senior Research Fellow, Dr. Bradley Jones, followed by Dr. Ryan Lekivetz, whom Brad hired years ago and who now leads JMP R&D innovation efforts for DOE. We had some meaty follow-up questions, so we are pleased that Ryan could share more of his expertise.
Could you please send the list of the books and articles mentioned during this webinar?
Goos, P. and Jones, B., 2011. Optimal design of experiments: a case study approach. John Wiley & Sons.
Jones, B. and Nachtsheim, C.J., 2011. A class of three-level designs for definitive screening in the presence of second-order effects. Journal of Quality Technology, 43(1), pp.1-15.
Fedorov, V.V., 2013. Theory of optimal experiments. Elsevier.
Box, G.E., Hunter, W.H. and Hunter, S., 1978. Statistics for experimenters (Vol. 664). New York: John Wiley and sons.
Jones, B. and Goos, P., 2007. A candidate-set-free algorithm for generating D-optimal split-plot designs. Journal of the Royal Statistical Society Series C: Applied Statistics, 56(3), pp.347-364.
Anderson‐Cook, C.M. and Lu, L., 2023. Is designed data collection still relevant in the big data era? Quality and Reliability Engineering International.
Lekivetz, R. and Jones, B., 2015. Fast flexible space‐filling designs for nonrectangular regions. Quality and Reliability Engineering International, 31(5), pp.829-837.
Could you provide us resources that demonstrate the usage of augment design to further optimizing the design space? Do you have any video link or book that covers this topic with specific examples?
Chapter 3 of Goos & Jones (2011) has an example of design augmentation:
A few presentations that discuss use of the Augment Design platform:
Specialized Custom DOE for Experienced Experimenters – JMP User Community
Using Definitive Screening Designs to Get More Information from Fewer Trials – JMP User Community
Some Discovery talks that take different approaches to design augmentation:
Exploring JMP DOE Design and Modeling Functions to Improve Sputtering Process (2... – JMP User Commu...
Candidate Set Designs: Tailoring DOE Constraints to the Problem (2021-EU-30MP-78... – JMP User Commu...
Big DOE: Sequential and Steady Wins the Race? (2021-EU-45MP-775) – JMP User Community
With the D/I-optimality evaluation with the DOE diagnostics, what does that even mean? How do I really get value or interpret these?
The optimality criteria are comparing to a theoretically optimal design (that may not even exist!).
https://www.jmp.com/support/help/en/17.1/index.shtml#page/jmp/optimality-criteria.shtml
By itself, it can be hard to interpret what it means. I usually like to think of these in comparison to other designs (like we do with relative efficiencies in Compare Design). https://www.jmp.com/support/help/en/17.1/index.shtml#page/jmp/design-diagnostics-2.shtml#)
Broadly speaking, D/A-optimality is for when you’re looking at estimating the terms in your model, while I-optimality is for when you are trying to do a better job estimating the response. I like Christine Anderson-Cook’s white paper on discussing some ideas in choosing designs:
Choosing the Right Design - with an Assist from JMP's Design Explorer | JMP
Session 2 featured scientist and JMP Systems Engineer, Peter Polito, who shared some fun and very relatable experiments he’s done with his family to show the equivalent of an “easy button” for DOE in JMP.
Is there a way to have JMP automatically generate a design that meets certain requirements, for instance, correlation coefficients no greater than 0.5, FPDS less than 0.25 for 80% of design space, etc.?
You can use both the Design Evaluation tools and Compare Designs platforms to home in on the best DOE for your situation.
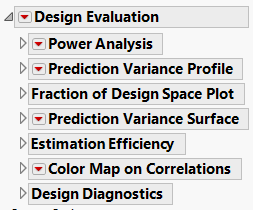
If you don't make good guesses about the coefficients, it doesn't seem like these latter tabs are helpful a priori. Am I understanding this correctly?
Yes and no. If you don’t have a good sense of what the coefficients will be ahead of time, the simulation panel becomes a great tool to explore how your model may look. While it may not be as informative to the ultimate outcome, you will have a good sense of the art of the possible, which you can use to explore the experimental space.
How do you identify that your model is robust?
“Beauty is in the eye of the beholder,” as they say. It depends, which is an ungratifying answer. Do you have a high R2? Does actual versus predicted match up? These are some methods to identify robustness. But at the end of the day, we build DOEs.
Did you really have a paper plane with a plane length of exactly 6.985 inches?
6.985 inches is ~17.74 cm. My ruler has gradations of 1 mm, so I was able to get pretty close but probably not exact.
Most of the time, it makes logistic sense to order the factors when running the experiments. How much am I losing if I don’t follow the random order that JMP suggested?
In part, you are losing the ability for JMP to accurately quantify and account for experimental noise and bias. At the end of the day, JMP can accommodate most of what you’d desire, it just needs to know what you are doing. So, if there is a particular order you’d like to go in, tell it. You can do this either by generating a split plot design (for hard to change factors) or setting a particular run order.
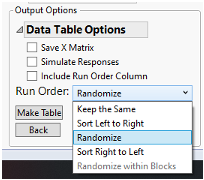
What were the results of the egg experiment?
What’s funny is I don’t actually remember the outcome I just knew as soon as I saw it I needed to do it myself—but I want one of the factors to be store bought vs. eggs laid by my chickens.
Can you show how we define and input our factors?
Enter responses and factors on the first page of the Easy DOE platform.
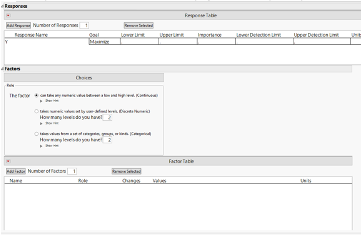
How does existing DOE tells if there is another important factor that may not be included?
There are several ways but the most telling is when our DOE results in no factors being significant. If no factor is significant, something else must be.
The side comment, we're curious on how you used DOE to optimize running?
Read about it here: https://community.jmp.com/t5/JMP-Blog/DOE-ing-Myself-Using-design-of-experiments-to-run-more/ba-p/25....
If there is a variable that is expensive to change, how is this addressed?
When adding factors, use the drop down menu to identify your variable as “Hard” to change, this will create a Split Plot design, which will order your trials such that the hard to change factor, changes as little as possible (among other things).
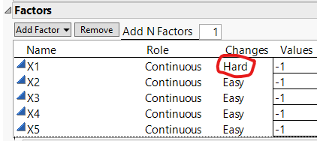
For RSM, how can you know the design is "good" before beginning the experiment. i.e., whether factors are correlated or prediction variance won't be too high?
I often refer to the Color Map of Correlations, in the Design Diagnostics section of Custom Design. This will tell you which variables and/or interactions you should be able to disentangle from the other variables.
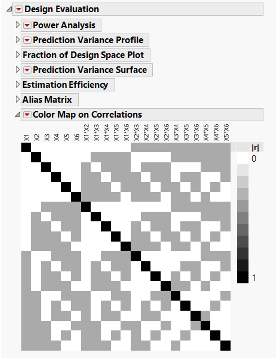
If you want more analysis tools, is there is an easy way to export the data from Easy DOE to a standard data table?
Yes, there is an “Export Data” button on the “Data Entry” tab.
When you use JMP to build the DOE table, let’s say you decided to add several factor changes on levels. I know when you go back to model and re-built DOE backward, sometimes it functions weird (specifically number of run goes up and down). Is that normal to see?
If you add additional factors or additional levels to nominal factors, JMP will likely increase the number of required runs to build a robust, interpretable model.
Can JMP handle, "Order of Addition" DOE's, i.e., where you're dealing with permuations effects?
Balanced Incomplete Block Designs (BIDB) may be a way to build a DOE in this way
If we already have some data, can we use that to improve the model prior to starting the DOE and doing more experiments?
Mike Anderson’s session (the third in the series) covers this very well (see link to the series below).
Can you talk more about the value of removing non-significant factors from the model and then reanalyzing?
In short, non-predictive models add noise. Removing them reduces noise, which increases the signal.
Would it be possible to briefly explain the purpose of simulation again?
The simulator allows you to “see” what your model may ultimately look like, based on the coefficients you think are likely. It is a preview of what may happen. If, for example, you have a good handle on the coefficients and you want to see how a particular interaction plays out, you can do so. If you cannot gain enough resolution on that interaction, you can add more runs, then re-simulate and see if there is improvement.
Session 3 covered more ways to derive value from your experiment and observational data, featuring chemist and JMP Systems Engineer, Dr. Mike Anderson.
A significant intercept indicates the factors do not adequately explain the results. When this occurs in my analyses, I work with the factors before coming to conclusions. So, yes, the intercept is always there, but when it's significant, I work to eliminate factors or look at interactions until the intercept is not significant. Are you saying this is not important within JMP's algorithms?
In the context of the SVEM report, the percent nonzero data says how often the intercept is different from zero. This is different than assessing the significance of the intercept based on a statistical test.
Lasso and Elastic net are regularization techniques used in regression to prevent overfitting. Elastic Net combines L1 and L2 penalties, while Lasso uses only the L1 penalty? Is this correct? And how to choose between these two methods?
L1 is the penalty for a Lasso regression. L2 is for a Ridge regression. The Elastic net is a linear combination of a Lasso and Ridge penalty with an extra parameter (alpha) to control how much each contributes to the total penalty. In general, Lasso is used for variable selection or cases where the covariance between factors is low. Ridge is generally used for situations where we suspect factors are correlated. Elastic Net is useful for situations where you need to do variable selection but are concerned about some potential factor-factor correlation.
Some useful details on these penalties can be found in the statistical details for the documentation: https://www.jmp.com/support/help/en/17.1/index.shtml#page/jmp/statistical-details-for-estimation-met...
There is also a great class offered by JMP Education on how these three penalties work. The course is called Finding Important Predictors: https://www.jmp.com/en_us/training/courses/jmp-and-pro-finding-important-predictors.html
We greatly appreciate our specialists taking time to provide more of their expertise and to recommend resources. We hope you find them useful. If you missed any of the Smarter Innovation with Designed Experiments series, you can watch them on demand. Additional resources are listed on the watch pages for each session. If you’d like to share resources you think are particularly useful, we invite you to put them in the comments. Thanks!