Sleeping has always been one of my family’s favorite activities. My wife, Kelly, and I have different philosophies on sleeping. I tend to be in the camp with Benjamin Franklin, who said, “Early to bed and early to rise makes a man healthy, wealthy, and wise.” Kelly prefers Mindy Kaling’s viewpoint: “There is no sunrise so beautiful that it is worth waking me up to see it.” Either way, we both enjoy our sleep.
Our mutual love of sleep inspired me to write this post. I wanted to look at the impacts of some of our behaviors on sleep, and Matt Walker’s TED talk gave me the perfect test focus. In the talk, Matt shares how caffeine and alcohol affect sleep. He mentions that caffeine is a stimulant that stays in the blood for much longer than we think. Caffeine has a half-life of 5-6 hours – meaning if you drink a cup of coffee in the afternoon, half of that caffeine is still in your system at bedtime. Matt also mentions that alcohol is a sedative, so it affects the quality of sleep.
Measuring sleep
To measure my own sleep, I used two devices: a Fitbit Charge HR and an Apple Watch Series 3 with the AutoSleep application. I found that the two different trackers did not have the exact same measures of sleep, but the amount of total sleep, quality sleep, and awake time were in good agreement.

Sleep Study
For the study, I needed to recruit some willing victims, umm, I mean participants. Thank you to Eric Hill (@Eric_Hill), Holly Connors (@holly_connors), Hadley Myers (@HadleyMyers), Arati Mejdal (@arati_mejdal), Brian Watts (@brian_watts) and Juliette Plager (@Juliette_Plager) for your help with this study. All these participants had a Fitbit or Apple Watch to measure their sleep. I asked each of these participants to keep their bedtime routine as consistent as possible for the week of the study, with the only change being if they consumed caffeine or alcohol that evening.
I was interested in different people who had different impacts with the two substances. Kelly and I have different tolerances to caffeine; while I am very susceptible to it, Kelly seems to have a much higher tolerance. Would it be the same for this group, or are different people impacted in different ways?
I made a DOE using the Custom Design platform in JMP, with the person as a blocking factor. Blocking factors let you group homogeneous factors (person in this case) to isolate extraneous noise associated with the factors. This means you expect less variation in sleep from a single person than you would across a group of people. In this setup, I added my block as a fixed effect as I wanted to estimate the difference between each person. To learn more about blocking factors, watch this webcast by my colleague @DonMcCormack on the topic – it is a great resource.
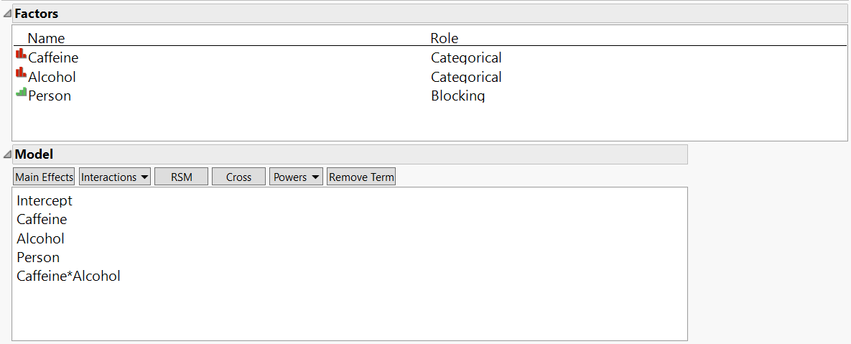
The design gave me good power to predict the impact of caffeine, alcohol, and the impact of different participants.

Results
As you can imagine, different people sleep for different amounts of time on average, and this group was no different. Sleep time for this group ranged from under six hours to more than nine hours a night.
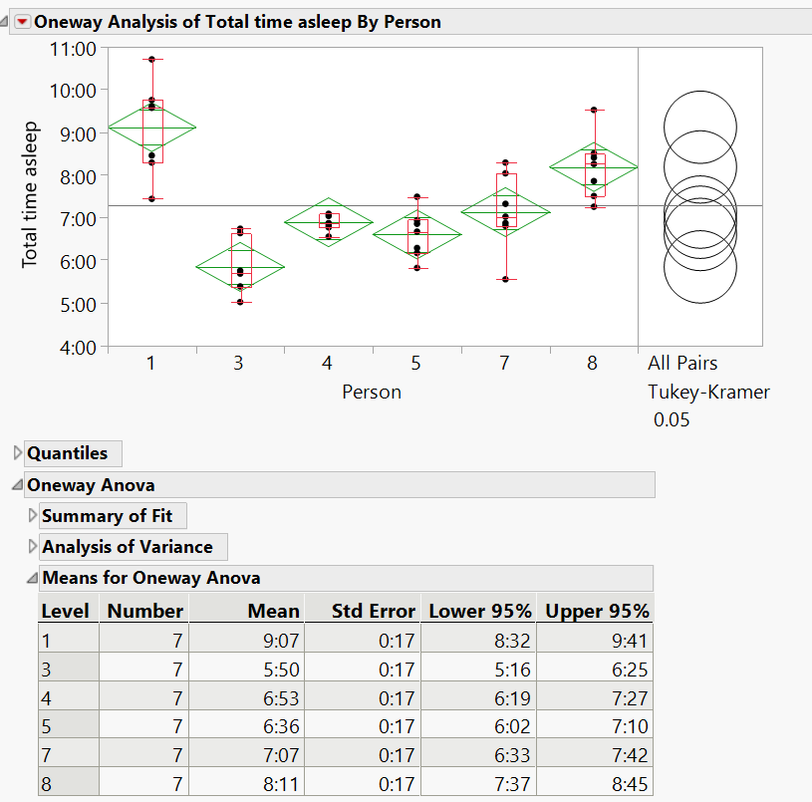
This person to person variation adds a layer of complexity when analyzing the data. To properly analyze this data, we need to take that added variation into account. For example, if you looked at the impact of caffeine on total time asleep without taking a person into account, there is too much variability to see the statistical significance. Luckily when you create a DOE in JMP and have a blocking factor, JMP knows how to analyze the data.
As you can see from the Profiler, drinking caffeine in the afternoon led to a reduction in total time asleep and quality sleep. Drinking alcohol at night had very little impact on the overall amount of sleep, but it did show a drop in quality sleep, leading to lighter sleep.
Only one participant in the study got more sleep when caffeine was consumed in the afternoon. However, this participant did also have a slight drop in quality sleep as a result. As for alcohol, only one of the participants had an increase in quality sleep after consuming alcohol.