We were very happy to have had so much interest in the recent Technically Speaking featuring Model Screening, a powerful new feature in JMP Pro 16. When you’re building models, you never know which method will work the best on any given set of data.
With Model Screening, you can try multiple approaches at once, assess the model performance and choose the best-performing model, including customizing the decision thresholds according to your needs. This powerful new feature generated so many questions, we couldn’t answer them all. So, we are addressing them in this blog post. You can see the speed and power of Model Screening in this episode of Technically Speaking.
Kemal Oflus setting the stage to show the Model Screening platform.
General Questions
When we try to fit nonparametric models, we might achieve high predictability, but we lose interpretability of the model. How do we achieve that balance between predictability and interpretability in real-world manufacturing problems?
In this episode of Analytically Speaking, Galit Shmueli dives into the details of this topic with her much-cited paper, To Explain or to Predict. We have metrics like RSquare that summarizes model performance in terms of how much of the variance can be explained by the underlying model. A model with higher RSquare is technically better than a lower one. Model interpretability provides insight into the relationship between the inputs and the output. An interpreted model can answer questions as to why the independent features predict the dependent attribute. The issue arises because as model accuracy increases so does model complexity, at the cost of interpretability. For this case, the JMP Profiler helps us visualize the underlying model so we can use the subject matter expertise to verify the sanity and the feasibility of the model. A model with fewer parameters is easier to interpret. This is intuitive. A linear regression model has a coefficient per input feature and an intercept term. For example, you can look at each term and understand how they contribute to the output. Moving to logistic regression gives more power in terms of the underlying relationships that can be modeled at the expense of a function transform to the output that now too must be understood along with the coefficients. A decision tree (of modest size) may be understandable, a bagged decision tree requires a different perspective to interpret why an event is predicted to occur. Pushing further, the optimized blend of multiple models into a single prediction may be beyond meaningful or timely interpretation. Again, JMP Profiler is the perfect tool to visualize and interpret the models we build.
When assessing regression models, is there a standard range for a good RSquare metric that signifies a good model, or does it depend on the context?
This will depend on the context; if trying to predict the stock market, 55% RSquare will be more than adequate versus when you are trying to predict if a heart valve will fail.
What is the difference between the training set and the validation set? Can you go into more detail on what type of data scenarios would be in those sets?
The training set is what we use to learn from data (and build the model); the validation set is to validate what we learned from the training set. If there are enough data, it is a better practice to also have a test data set to further tune the model, then validate the results with the validation set.
Is there a reason to not use PLS?
No particular reason, just that it is harder to interpret and not really appropriate for the data sets that we looked at during the webinar.
Some assays have a larger error. Is there a way to enter expected error in the models?
If this is a question about using a classifier, by default the classification threshold is set at 50%, but depending on your circumstances, you can modify this threshold.
Regarding the sample on coffee, why did the neural boosted method have a lower sample size versus all the other models (which were identical to each other)? Did the RSquare value for neural boosted receive an advantage by selecting more appropriate data?
That is because a few of the selected input factors had missing values; some algorithms can handle the missing values and hence, use the rest of the information from the given observation. However, since neural networks cannot, a smaller number of observations are included in the sample.
How would one adjust and compare parameter settings of several models?
The model screening and comparison platforms provide a way to average/combine/aggregate multiple models so that you can create an ensemble model, which you can then use to optimize parameter settings.
It seems like this process might be prone to overfitting. How would you compare this with AIC?
AIC values are presented for the appropriate models in the individual model summary section of the model screening platform. The details in the online help cover this and more in the Model Comparison Report.
Doesn't the data scientist fill a critical role that JMP cannot? For example, the data scientist can balance between getting more true positives (TP) at the risk of increasing FP in a Classification problem. How does JMP deal with this tradeoff and communicate it to the modeler?
Software cannot replace the role of a subject matter expert (in this case, the data scientist), who is the bridge between the data and the applicable use cases. However, the tools and reports JMP provides with the model evaluation metrics (RSquare, adjusted RSquare, misclassification rate, confusion matrix and most importantly, the profiler) make communicating the findings to different levels of the organization extremely easy.
JMP Capabilities Questions
Can we do dose response logistic modeling in JMP?
Yes, this post in the Community and this one from JMP partner, Pega Analytics, cover more about how to do this in JMP.
Can we run agent-based modeling in JMP?
Not at this time.
Can the user specify values of model parameters or are default values assumed?
The Model Screening platform is designed to find the optimal parameter settings (for example, the number of trees, boosted layers, etc.). You can include interaction terms for linear models. Once the best model (or a set of models) is found, each model can be further customized.
Is there a validation column in your worksheet? Does this populate automatically?
If you have a validation column, you can utilize it. If not, JMP provides multiple ways to create a validation column. See options of how to do this.
Is there any way to get model analysis (such as Prediction Profiler) for a model created in Python if I put the data and predictions in JMP?
There is an Excel profiler in the Excel JMP add-in that can only be used for models created in Excel. However, JMP can execute your Python, R, or MATLAB scripts and/or exchange data back and forth, provided you have access to the software.
What about creating custom variables that might work best in a model created from raw data? Does this platform handle this or is it necessary to create these first to have them as inputs?
The Model Screening Platform uses the data as is; if you believe there should be some compound variables like Principal Components, they will have to be created separately. Simple interaction variables like a*b or quadratics can be selected via checkbox in the dialog box. Also, each node in the neural network also corresponds to a compound variable that might have a physical meaning (but again we do not have any control over those).
Can JMP incorporate a model created in R or Python?
If you have R or Python script that uses a model to predict results, you can have JMP run the R/Python script and use the results as a new column variable, if need be. This white paper, “JMP Synergies: Using JMP and JMP Pro With Python and R,” is a great resource.
Have you used JMP against textual data at all?
JMP has a built-in Text Explorer platform. This JMP Discovery Summit talk has some nice examples and the online help gives a good overview.
In Prediction Profiler, when we see behavior of y versus one of our x’s, is it established, considering that it is the only x or does it assume the behavior knowing there are other x's in the model as well?
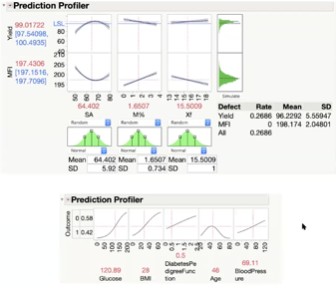
The Profiler provides a way to investigate and optimize the relationship between multiple input variables to desired output variable. The input variables can be setup to be independent of each other or may be interacting with one another. This video shows how to use the Profiler. Our own Bradley Jones, Distinguished Research Fellow in R&D, wrote this JMP Foreword article in celebration of the 30-year anniversary of the Profiler, one of our most popular interactive visualizations.
You must be a registered user to add a comment. If you've already registered, sign in. Otherwise, register and sign in.