The 2024 NFL draft begins Thursday, April 25, and will be full of intrigue for NFL fans. For teams near the bottom of the standing, the draft represents hope for the future. The right player can turn around the course of a franchise, while the wrong pick can set a team back for years. As a result, many teams select quarterbacks (QBs), which is the most important position in football, in the first round of the draft. There has been a total of 48 QBs selected in the first round of the draft since 2008. That means, on average, every team in the NFL has drafted 1.5 QBs in the first round in that 16-year period. Each year, at least one – and as many as five – quarterbacks have been picked in the first round.
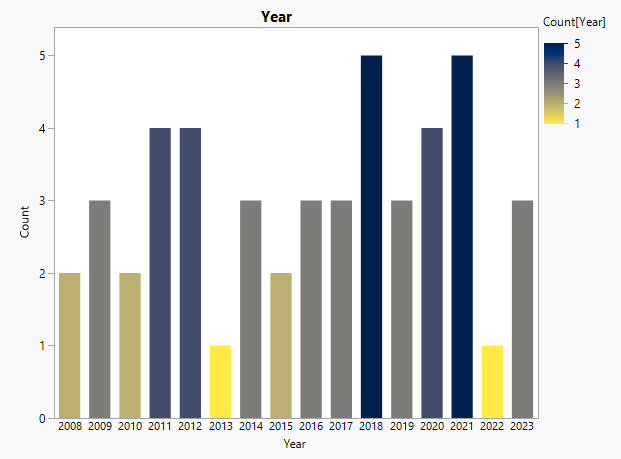
Twenty-eight of the 32 NFL teams have selected at least one QB in the first round since 2008. The four teams with no first-round QB picks are the Raiders, Seahawks, Cowboys, and Saints. As seen in this graph, the Browns, Jaguars, and Jets lead the way with three QBs picked in the first round since 2008.
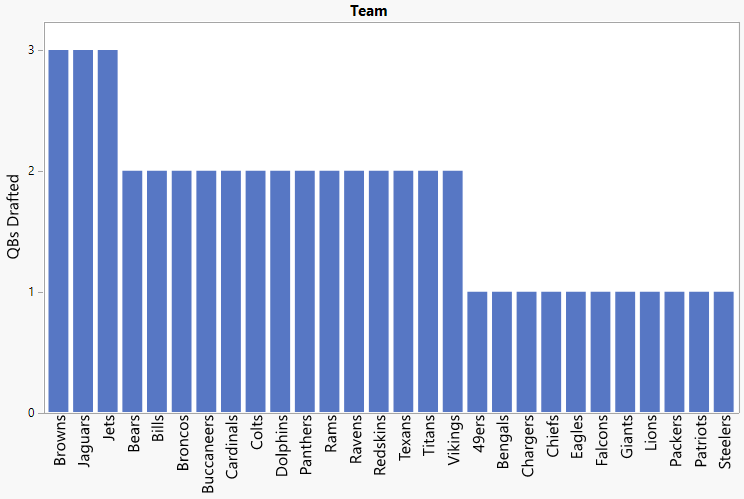
Not every QB drafted in the first round turns into a star. (The NFL stats for all 48 first-round drafted QBs were imported from sports-reference.com.) The stats per year for each QBs were calculated, and a hierarchical clustering analysis was run to group the QBs. (From the cluster analysis, Bryce Young, the first pick of the 2023 NFL draft, had a unique statistical year when compared to the other QBs. Young was sacked a staggering 62 times in his 16 games and threw only 11 TDs in his 527 pass attempts. The stats from Young last year were so unique that he ended up in a cluster by himself. For the analysis, it was determined the best approach was to remove Young from the cluster analysis.) The remaining 47 QBs were used in the cluster analysis and grouped into five clusters, as shown below. The QBs in Clusters 1 and 2 are playing a high percentage of the games with fairly good results. The QBs in Custers 3, 4, and 5 are not playing as often and not having as much success when they do play.

If we examine each cluster with the actual QBs, this looks about right – with a few caveats. Looking at Clusters 1 and 2, these are mostly QBs that you would be happy to get in the first round of the draft, so I label them as good picks. The only two QBs out of the list that didn’t have great NFL careers are Blake Bortles and Mac Jones, but from their stats, they cluster mostly with good picks.
Clusters 3-5 are mainly what you would consider bad picks. You might make an argument that Joe Flacco doesn’t deserve to be called a bad pick because he has won a Super Bowl, but he is statistically aligned with the bad picks. Anthony Richardson and Jordan Love fall into Clusters 4 and 5 respectively, which would mean they would be considered bad picks, but they have both had limited opportunity to play. So it was determined that it would be best to remove both of those QBs from the analysis. With Young being excluded from the cluster analysis and then removing Love and Richardson, we now have 45 total QBs to be used for a model. Of these 45 QBs, I have labeled 20 as good picks and 25 as bad picks.

To make a predictive model, we need to look at data that is available before the NFL draft. We can find that data from college football stats. (The college stats for these QBs were pulled from sports-reference.com.) The model used was a neural network, and it correctly predicted 10 out of 12 picks on whether they were a good pick or not from a set of the data left out of the model building process. The two misses were Blake Bortles being called a bad pick and Marcus Mariota being called a good pick. This is a pretty good result for the model and might be labeled more accurate than my classification for good or bad picks.
For the 2024 NFL draft, the projections are that between four and six QBs will be selected in the first round. In this table, I have included the six QBs who are the mostly likely candidates to be drafted in the first round of the NFL draft.

The college stats for these six QBs were run through the neural network model. What does the model think is the likelihood of each of these six QBs becoming a good pick? The model gives Caleb Williams the best chance of being a good pick, with the probability being about 90%. The model gives national champion J.J. McCarthy the lowest probability of being a good pick, with less than ~ 0.1% probability. The probability of each QB being a good pick is shown in the graph below.

In summary, the NFL analysts and front offices have done slightly worse than a coin flip when it comes to successfully picking NFL QBs in the first round (20 out of 45). And that's with years of combined experience studying hours of film on each potential draft pick.
In one afternoon of doing some web scraping and using the built-in modeling tools inside JMP Pro, this is the prediction I came up with. The model says Caleb Williams and Michael Penix Jr. are more likely to be good picks if drafted in the first round, while and the other four QBs are more likely to be bad picks. If the model gets three of these right, then it is more accurate than the NFL has been in the past 16 years. I am happy to show any QB-needy NFL team how to use JMP Pro and how I built this model.