I have a dataset with 4 treatments (Locations) that were repeated for 3 Years. When I run a full factorial (Year, Location, Year*Location) I get the nonEstimable error message, as seen below. When I analyze just Year and Location with no interaction, I do get results as seen below. Now when I don't select a By element in the Fit Model control panel, specifically don't select Cultivar as a By element, I do get a result for the full factorial, as seen below.
1) Do I not have enough degrees of freedom to test the Full Factorial with a By element? Is why I am getting the NonEstimable message. I know the message has to do with confounding factors but to be honest I don't know what I am supposed to do as a solution.
(I have pasted some of my data to give an idea of what I am working with, please note the sample size being small and unbalanced for example)
2) If my DFs are too low, are there any solutions/alternatives to testing the interaction?
Full factorial- nonestimable:
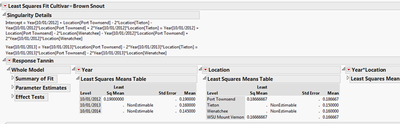
No interaction:
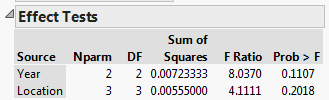
Full factorial without By element:
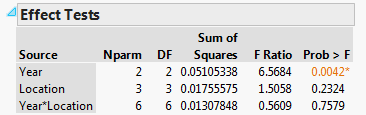
Data snapshot:
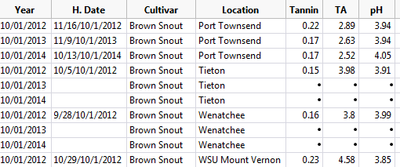