Hi @RaviK,
There are a few ways you could approach this, but perhaps the most straightforward is a Chi-Square Test of Independence, a type of contingency analysis. This test is appropriate because what you have are two categorical variables, a grouping variable, and a categorical outcome (success or fail), and you are interested in whether the observed proportions of the categorical outcome for your two groups provide evidence that the process generating the outcomes differs between the groups. You can obtain this test using Analyze > Fit Y by X. But first, you'll first need your data entered in a particular way (also attached): 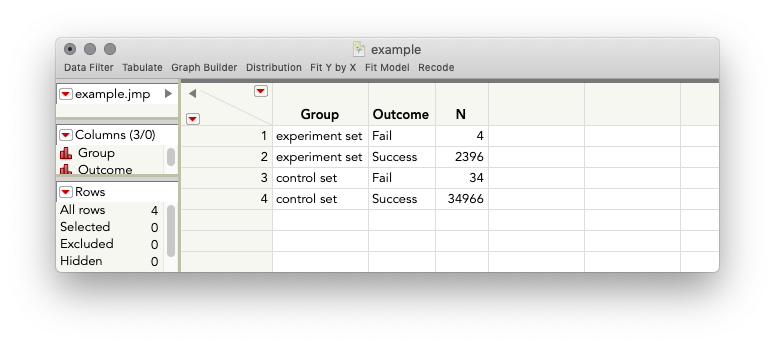
What I've done is taken the numbers you provided me and made columns for Group, Outcome, and N, the number of observations in each. For the number of successes, I simply took the total you gave minus the failures. Next, we run Analyze > Fit Y by X: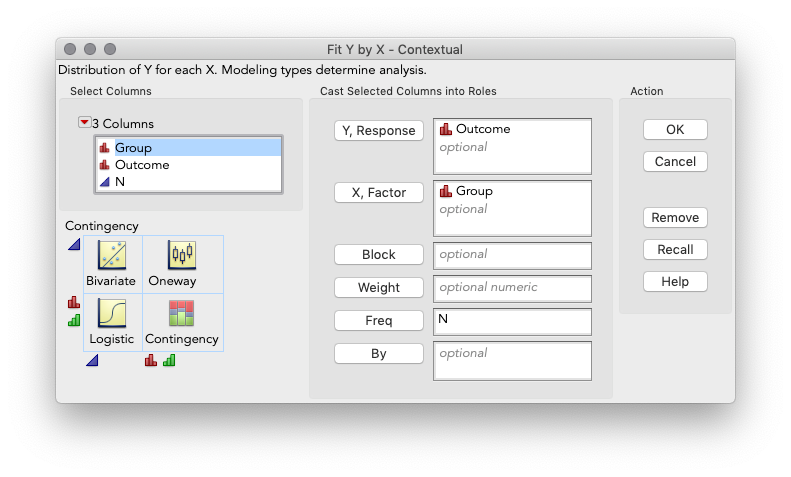
Here I've cast Outcome in the Y role, Group to the X, and N as the Freq, or frequency of occurrence. When we hit OK, we get the output below (I've hidden the mosaic plot since your observed frequencies of fail are so low that the plot is not helpful). 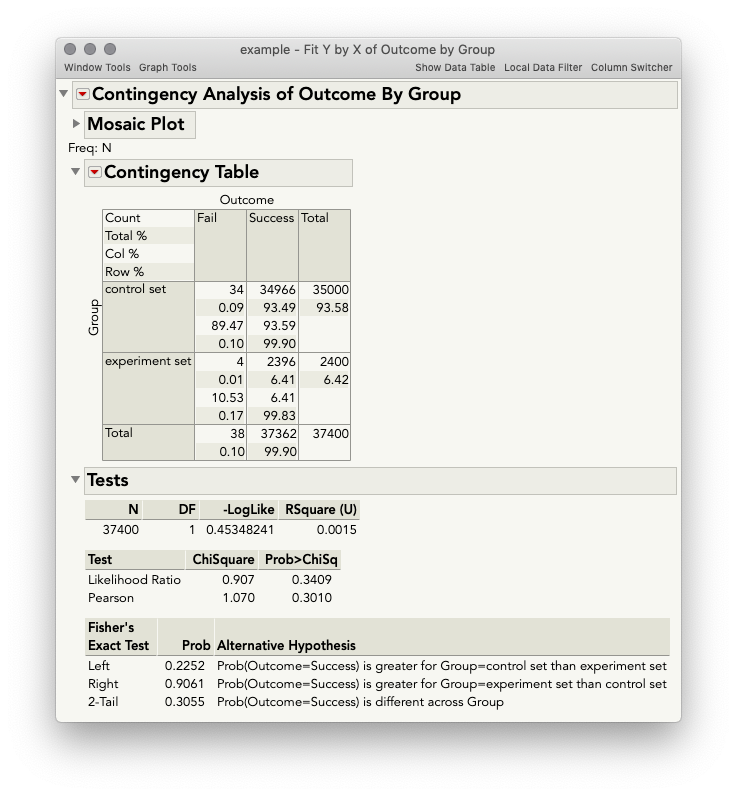
Our p-value of interest is the Likelihood ratio, or Pearson (classical Chi-Square test of independence), both of which are around p = ~0.30, indicating that if there isn't any true difference in the experimental and control group processes, a difference in the proportion of failures you observed in these sample data (or a difference more extreme) would occur about 30% of the time when taking samples of the sizes you had. In other words, not very convincing evidence that there is a true difference in these sets. Given what appears to be a large difference in the proportion of failures this may be surprising; but, given the low failure count overall, it's relatively easy to observe differences in the proportions of this magnitude or greater simply by chance (which is what this statistical significance test is telling us directly).
I hope this helps!
@julian