Quick response is that we don't have the tool to address the situation yet: repeated measure with censoring.
If we can ignore the fact that the measurements are repeated on individual computers, we can still get something out of the data.
The following are what I did: a Graph Builder report to look at the data; a Parametric Survival report to fit some models; a Life Distribution report to inspect residuals; and finally a Graph Builder report to look at the data and predicted values side by side.
First is a plot of the data: Duration vs. Atempt, grouped by OS, overlaid by Computer ID. So by OS, they all present a downward trend. Windows10 machines do stay tightly. Other machines stay more or less stay together by OS. IOS and Linox have relatively more right censored observations. So a preliminary answer to your interest is that Atempt and OS matter.
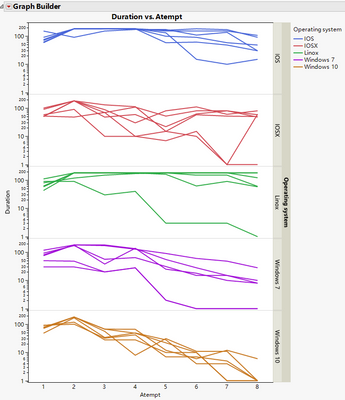
Now I attempt to fit a model. Based on what I see in the plot, a Parametric Survival analysis should be appropriate. So the setup is to see whether I can fit a distribution to Duration, while the location parameter of the distribution is a linear function of the effects. The Scale Effect tab is empty, so I am not considering the scale parameter of the distribution is a linear function of some effects, but it can be tried later to see whether it matters.
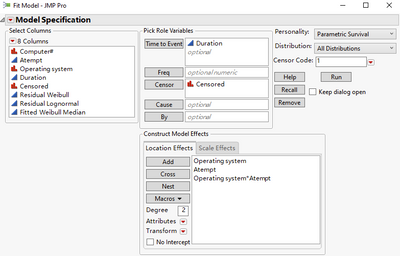
The report says Weibull is the best fit, followed by Lognormal. Also individual distribution reports show effects are significant.
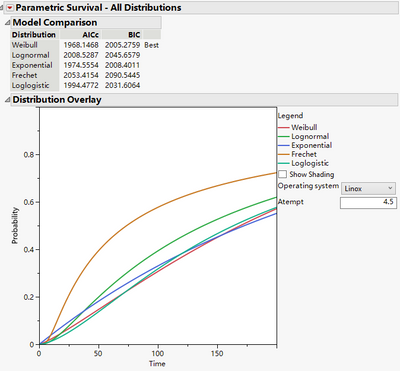
I then save the residuals from Weibull and Lognormal results back into the table. The use Life Distribution to look at them. Notice when put Residual into the Y role in Life Distribution, the Censor column needs to go to the Censor role, like what's in the following screenshot.
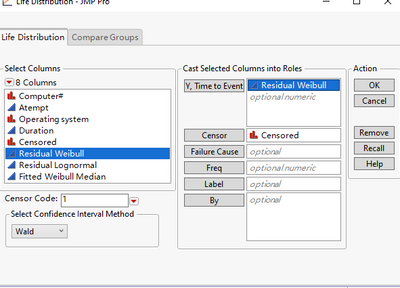
Analyze the Lognormal residuals similarly and compare the two reports. Weibull does seems to be a better fit, because linearization seems to better fit Weibull. Lognormal has a more significant kink.
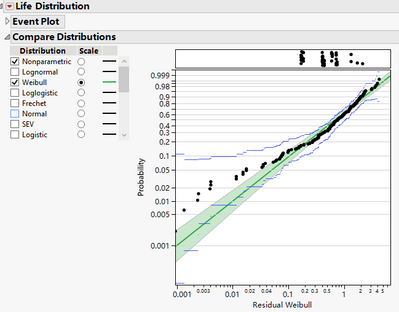
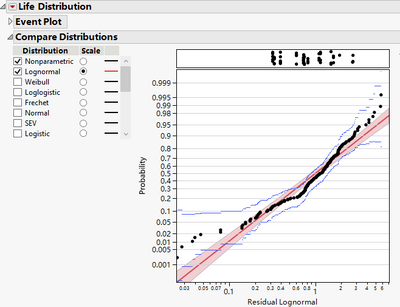
Then from the Parametric Survival report, I save "Quantile Formula" from the Weibull result. When it prompts for "probability", enter 0.5, for the median. Now the data table has an extra column, and I name it "Fitted Weibull Median". Then I stack "Duration" and "Fitted Weibull Median", and get a new data table, so for every observation in the original table, I have a corresponding predicted median.
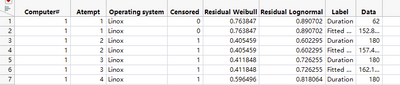
Use Graph Builder to put data and predicted values side by side, the model looks pretty reasonable.
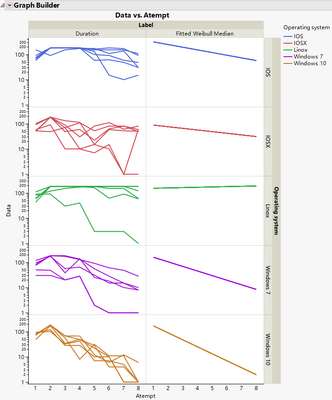
The above may not be the only plausible way to fit the data, but I am not going to exhaust them here. I attach the updated data table and the stacked data table as well.