Hi @VarunK,
There are several questions in your post, so I will try my best to answer step-by-step.
About the use of full factorial design and what to expect from it :
If you're planning to do a full factorial design on 4 continous factors with 2 levels with 16 runs, this means you assume your apriori full model will contain the intercept, the 4 main effects (for each factors) and the 6 interactions between 2 factors. In total, you have 11 terms to estimate, so you still have 5 degree of freedom left to assess the error : Analysis of Variance
I would recommend to start with the full model first, as it is your apriori assumed model so it may provide you a better overview of the relevance and influence of the different terms, instead of repeating the model fitting for a lot of different combinations without any prior knowledge (this would require a longer time, and you might get confused by different or conflicting models).
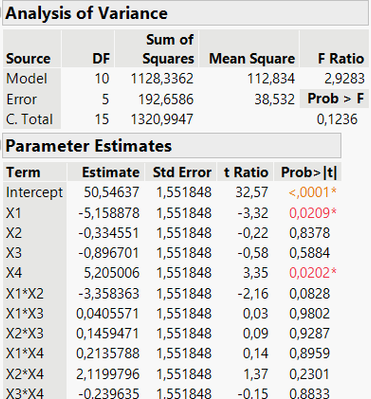
Of course, depending on your results, this full model may not be the most appropriate or relevant one, and there might not be one single model that may answer your needs.
Also if one (or several) factor(s) and its associated higher order terms (interaction effect) have no influence on the model, the runs corresponding to the estimation of these effects can be projected into the lower dimensional space, making them look like replicates. This is called the projection property of factorial designs, and the reason (with the sparsity of effects principle) why screening designs/fractional factorial designs can be very powerful and flexible. Several links are available on internet if you want to know more : Classical Designs-Fractional Fractorial Designs Rev1.pdf (afit.edu)
Projection Properties of Factorial Designs for Factor Screening | SpringerLink
And I also did a post on LinkedIn to explain intuitively how it works through a comparison with shadowgraphy : https://www.linkedin.com/posts/victorguiller_designofexperiments-statistics-dataanalytics-activity-7...
Adding replicates is a good technique to improve the estimation of the parameters/coefficients, but they can greatly increase the experimental budget. To provide a greater flexibility, and depending on the platform used, you can either use replicate (full replication of the design) or replicate runs (replication of a certain number of runs). For more info about this replication difference and the benefits of replicates, see https://community.jmp.com/t5/Discussions/Doe-and-replications/m-p/565296/highlight/true#M77731
About the way to analyze your DoE :
Depending on your objective(s), you may have different paths to models evaluation and selection :
- Explainative model : In an explainative mode, you're more focussed on the terms that do have some influence on the response(s), so you might evaluate the need to include the different terms based on statistical significance (with the help of p-values and a predefined threshold for it like 0.05) and practical significance (size of the estimates, selection based on domain expertise). R², R² adjusted (and the difference between the two, which needs to be minimized) might be good metrics to understand how much variation is explained by the identified terms, and select relevant model(s) to explain your system under study.
- Predictive model : In a predictive mode, you're more focussed on the terms that help you minimize prediction errors, so you might evaluate the need to include the different terms based on how this improve the predictive performances, through the visualizations of actual vs. predictive plot, and size of the errors (residuals plot). RMSE might be a good metric to assess which model(s) have the best predictive performances (goal is to minimize RMSE).
You might also be interested by a combination of the two parts, so different metrics could be used to help you evaluate and select model's, like information criteria (AICc, BIC) that help find a compromise between predictive performances of the model and its complexity. To evaluate and select a model based on these criteria, the lower the better. You might also use maximum likelihood which is similar but does not include a penalty for the complexity of the model.
As you can see, there might be several ways to evaluate and select the most relevant model(s).
I hope this answer will help you have an overview about what to do try next for your use case.
Victor GUILLER
Scientific Expertise Engineer
L'Oréal - Data & Analytics