Hi Rick,
Great to hear you're exploring the use of structural equation models with your data! I made up 3 rows of data to gain clarity on the model you're fitting. Based on the description of effects in your post, I have the following path diagram:
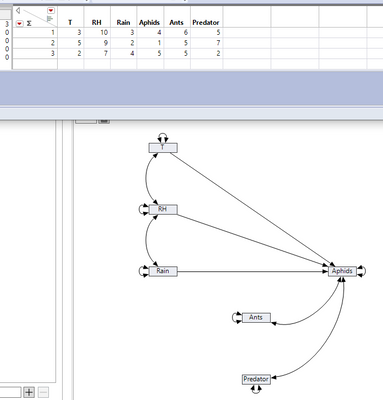
Is this similar to the model you're fitting? If so, I think there are some specification issues that might help. From your description of the problem in the first paragraph, I suspect a more appropriate model might look like this one:
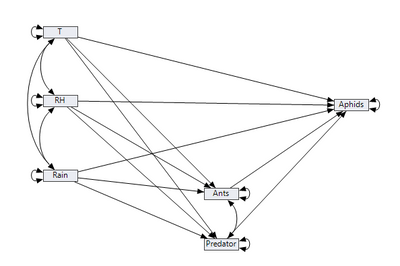
However, I'm not sure I understand how time is represented in your data. Do you have 32 repeated measures for each of these variables (1 measure per month)? If so, are your data in "wide" or "long" format? SEM requires "wide" format. For example, you might have Rain_1, Rain_2, ... , Rain_32 for the repeated measurements of rain. You might consider watching the video on this link, which describes how to model longitudinal data in SEM:
https://community.jmp.com/t5/Discovery-Summit-Americas-2021/Modeling-Trajectories-over-Time-with-Str...
To answer your specific questions:
1. Yes, it's fine, but the research questions change accordingly. If you took averages across all the repeated measures, then you're no longer able to examine time-sequential effects, which is often of interest when you have data taken over time.
2. CFI and RMSEA give you a sense of how well your model fits the data. This is of key importance because you only want to interpret results from models that fit well. Once you know your model fits the data, then you interpret the results, including the R2 of endogenous variables.
3. Reducing the number of parameters in a model should be done based on your desire to test specific hypotheses. For example, based on the second image I pasted, I might decide to fit two models: 1) a model just as I depicted, and 2) a model where I eliminate the unidirectional paths from climate (T, RH, Rain) to Aphids. I could then compare the two models to test the hypothesis of whether climate has a unique direct effect on Aphids above and beyond the indirect effect that goes through Ants and Predators.
HTH,
~Laura
Laura C-S