I'm using multidimensional scaling on a 91 x 91 distance matrix. When the 2D MDS plot is displayed, the points are plotted using various different symbols and colors assigned by JMP, evidently clustering points togethers based on their proximity in the multidimensional distance space (see attached screenshot). Questions:
- What is the criterion that JMP is using to decide how to group or cluster points?
- Is there any way to adjust the criterion or threshold for how points are clustered?
- Is there any easy way to get a report on how many clusters have been found (other than manually counting them on the plot; not practical for large data sets)?
I have searched JMP documentation and Google and have not been able to find anything on this.
I'm looking (preferably) for answers that are not dependent on scripting.
Thanks in advance for any assistance.
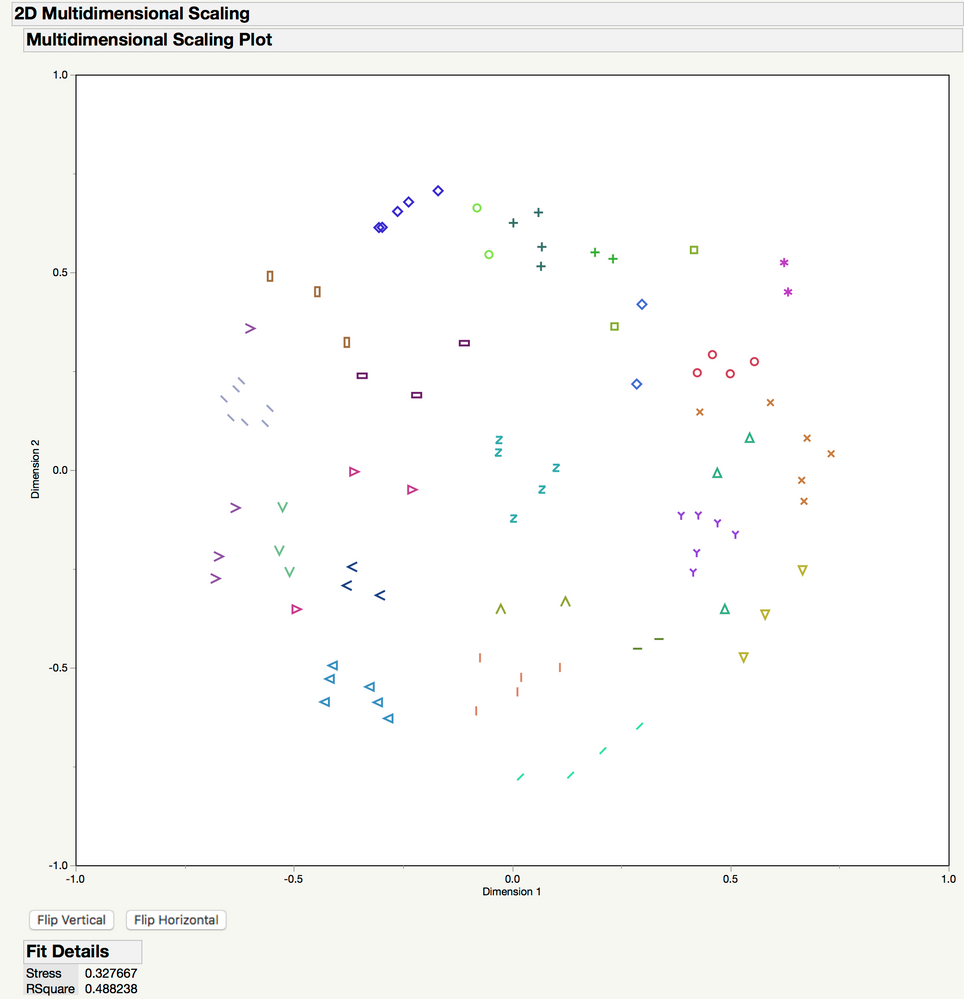