Hi @LatinLinkages95,
Ok thanks for the clarification, I understand better your objectives and needs.
An Extreme Vertices design might be helpful for the first part (assessing the influence and relative importance/benefit of each mixture factors on the responses), for the second part you can use the Augment Design platform, select the important mixture factors detected in the first part and the responses, and either use a model-based approach (add runs to the design in order to fit a specific model, like Mixture Response Surface model) or a model-agnostic approach (add space filling points to a design in order to homogeneously improve the coverage of your experimental space) that will let you some flexibility in the modeling part (regression models, Machine Learning models, ... to fit a Response Surface).
About your questions :
- You can add the constraints the way you intended to do it in your first post (with positive values for factors and inequality constraints >= and =<), it should work, no need to add the constraints with negative values. The negative values writing is used by JMP to handle any inferior linear contraints. In a simple example with 3 mixture factors, I specified that X1 + X3 should be between 0,5 and 0,8 and here is how this constraint is translated by JMP in the table :
{1 * :X1 + 1 * :X2 - 0.8, -1 * :X1 + -1 * :X2 + 0.5}
You can see that for the inferior linear constraint, JMP did rewrite it like - X1 - X2 =< -0,5 (or -X1 -X2 + 0,5 =< 0), it's just a way to have the same JMP scripting language writing convention as for the superior linear constraint with the same inequality sign, but you don't need to do it yourself.
Adding the same constraints with positive and negative values is helpful if you're in a situation of mixture of mixtures, where some components should sum up to a certain percentage (like A+B = 0,8), and adding the rest of the mixture components sum up to 1 (or 100%, like A+B+C+D).
- Based on your requirements, I would set up the linear constraints like these :

- X3 has a very narrow range (0 - 0,08) so that may explain why other levels are not proposed. Are you sure about specifying an individual (narrow) range for X3 in addition to the linear constraint for X3 to X12 ? Same question for X1 and X2 with their individual constraints and their linear constraint.
Also you're right, the degree of the design is an indication about the maximum number of mixture factors that can be involved in the experiments (from 1 to the degree). But since your primary focus is evaluating the influence and importance of each mixture factors and the subset selection had a D-Optimality criterion (and you have a limited budget compared to the number of possible combinations), even with a higher degree the subset proposed with a 25-runs budget will put high emphasis on experiments with few components :
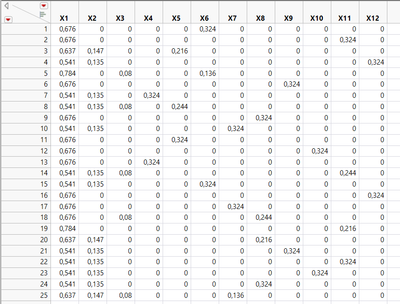
Here is the script to generate this design :
DOE(
Mixture Design,
{Add Response( Maximize, "Y", ., ., . ),
Change Factor Settings( 1, 0.541, 0.784, "X1" ),
Change Factor Settings( 2, 0, 0.147, "X2" ),
Change Factor Settings( 3, 0, 0.08, "X3" ), Add Factor( Mixture, 0, 1, "X4", 0 ),
Add Factor( Mixture, 0, 1, "X5", 0 ), Add Factor( Mixture, 0, 1, "X6", 0 ),
Add Factor( Mixture, 0, 1, "X7", 0 ), Add Factor( Mixture, 0, 1, "X8", 0 ),
Add Factor( Mixture, 0, 1, "X9", 0 ), Add Factor( Mixture, 0, 1, "X10", 0 ),
Add Factor( Mixture, 0, 1, "X11", 0 ), Add Factor( Mixture, 0, 1, "X12", 0 ),
Set Random Seed( 119094230 ), Add Constraint(
[-1 -1 0 0 0 0 0 0 0 0 0 0 -0.676,
1 1 0 0 0 0 0 0 0 0 0 0 0.784,
0 0 -1 -1 -1 -1 -1 -1 -1 -1 -1 -1 -0.216,
0 0 1 1 1 1 1 1 1 1 1 1 0.324]
), Mixture Design Type( Extreme Vertices, 8 ), Find Subset( 25 ),
Simulate Responses( 0 ), Set Run Order( Randomize ), Make Table}
)
And attached you'll find the datatable.
Hope these answers may help you better understand,
Victor GUILLER
"It is not unusual for a well-designed experiment to analyze itself" (Box, Hunter and Hunter)