Here are the options that I see.
First, the Distribution platform. Choose "Tolerance Interval" item, then choose "Nonparametric" method. See next two screenshots.
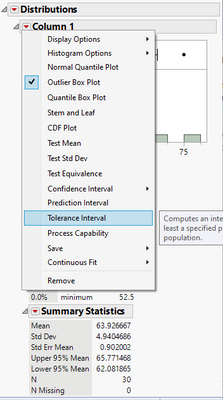
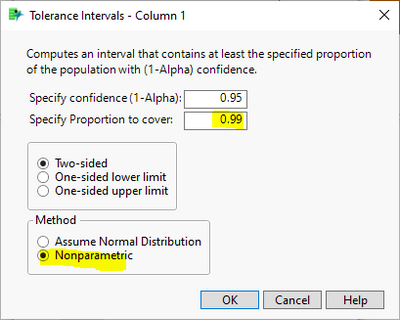
Second, the Life Distribution platform. This platform was not designed to answer tolerance interval questions specifically. But I have explain how it can be repurposed via confidence intervals.
1.First launch the platform, the item is under Analyze > Reliability and Survival > Life Distribution. Configure dialog, and your data column goes into Y.
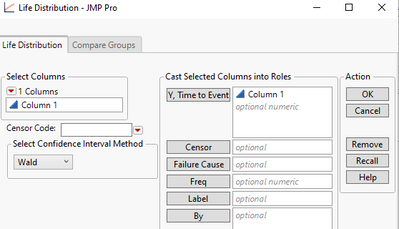
2. Change Confidence Level to 0.975. (Ignore the title shows 98% due to rounding in display, which is a bug I just noticed now. Ignore if column title organization may look different in your version, there is a change in the layout in JMP17.)
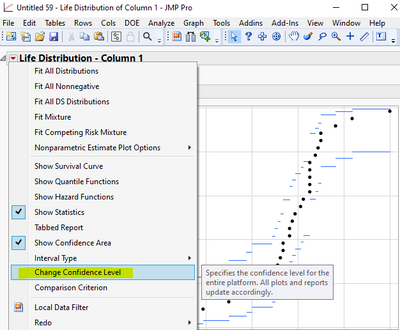
3. If Weibull is desired, first fit Weibull, and seems that your data fits the distribution well.
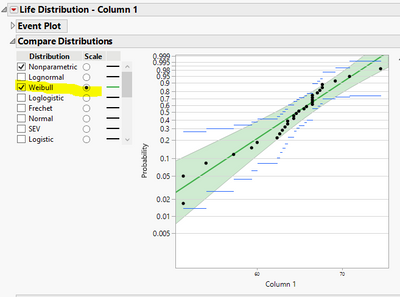
4. Choose "Custom Estimation" from the distribution result's menu.
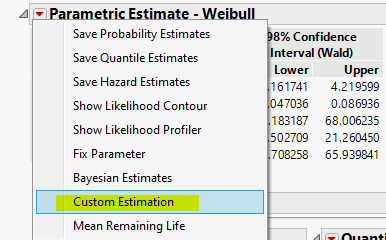
5. Choose "Lower" in the following menu.

6. Enter 0.005 in the Probability. Record the lower bound.
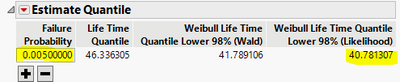
7. Now change to "Upper"

8. Enter 0.995 in the Probability. Record the upper bound. You may want to consider using Likelihood type bound for your sample size.

The two bounds are the ones that I explained in item 4 of my previous response.