Hi,
Considering your description of the problem and the solution proposed by @statman in point#5, I created this design (table script below). I created a 32-run response surface with 5 continuous factors using Custom Design, and then grouped runs into random blocks of size 4 (corresponding to the number of flasks per incubator per day). In the table, I manually added a "Day" variable and adjusted "incubator" so that 1-4 repeat on both days. It would then be possible to account for the random variation by setting up the model as shown in the figure.
I'd be very interested to hear if @statman or anyone else has an opinion on this approach.
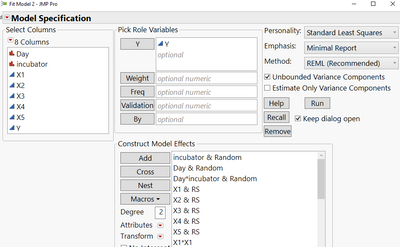
New Table( "day and incubator block design",
Add Rows( 32 ),
New Table Variable( "Design", "Custom Design" ),
New Table Variable( "Criterion", "I Optimal" ),
New Script(
"Model",
Fit Model(
Effects(
:incubator & Random, :X1 & RS, :X2 & RS, :X3 & RS, :X4 & RS,
:X5 & RS, :X1 * :X1, :X1 * :X2, :X2 * :X2, :X1 * :X3, :X2 * :X3,
:X3 * :X3, :X1 * :X4, :X2 * :X4, :X3 * :X4, :X4 * :X4, :X1 * :X5,
:X2 * :X5, :X3 * :X5, :X4 * :X5, :X5 * :X5
),
Y( :Y )
)
),
New Script(
"Evaluate Design",
DOE( Evaluate Design, X( Random Block, :X1, :X2, :X3, :X4, :X5 ) )
),
New Script(
"Fit Mixed",
Fit Model(
Y( :Y ),
Random Effects( :incubator ),
Effects(
:X1 & RS, :X2 & RS, :X3 & RS, :X4 & RS, :X5 & RS, :X1 * :X1,
:X1 * :X2, :X2 * :X2, :X1 * :X3, :X2 * :X3, :X3 * :X3, :X1 * :X4,
:X2 * :X4, :X3 * :X4, :X4 * :X4, :X1 * :X5, :X2 * :X5, :X3 * :X5,
:X4 * :X5, :X5 * :X5
),
NoBounds( 1 ),
Personality( "Mixed Model" ),
Run
)
),
New Script(
"DOE Dialog",
DOE(
Custom Design,
{Add Response( Maximize, "Y", ., ., . ),
Add Factor( Continuous, -1, 1, "X1", 0 ),
Add Factor( Continuous, -1, 1, "X2", 0 ),
Add Factor( Continuous, -1, 1, "X3", 0 ),
Add Factor( Continuous, -1, 1, "X4", 0 ),
Add Factor( Continuous, -1, 1, "X5", 0 ), Set Random Seed( 1910031543 ),
Number of Starts( 105 ), Add Term( {1, 0} ), Add Term( {1, 1} ),
Add Term( {2, 1} ), Add Term( {3, 1} ), Add Term( {4, 1} ),
Add Term( {5, 1} ), Add Term( {1, 2} ), Add Term( {1, 1}, {2, 1} ),
Add Term( {2, 2} ), Add Term( {1, 1}, {3, 1} ),
Add Term( {2, 1}, {3, 1} ), Add Term( {3, 2} ),
Add Term( {1, 1}, {4, 1} ), Add Term( {2, 1}, {4, 1} ),
Add Term( {3, 1}, {4, 1} ), Add Term( {4, 2} ),
Add Term( {1, 1}, {5, 1} ), Add Term( {2, 1}, {5, 1} ),
Add Term( {3, 1}, {5, 1} ), Add Term( {4, 1}, {5, 1} ),
Add Term( {5, 2} ), Set Runs Per Random Block( 4 ),
Set Sample Size( 32 ), Optimality Criterion( 2 ),
Simulate Responses( 0 ), Save X Matrix( 0 ), Make Design}
)
),
New Column( "Day",
Character,
"Nominal",
Set Property( "Design Role", Design Role( "Random Block" ) ),
Set Values(
{"1", "1", "1", "1", "1", "1", "1", "1", "1", "1", "1", "1", "1", "1",
"1", "1", "2", "2", "2", "2", "2", "2", "2", "2", "2", "2", "2", "2",
"2", "2", "2", "2"}
)
),
New Column( "incubator",
Character,
"Nominal",
Set Property( "Design Role", DesignRole( Random Block ) ),
Set Property(
"Value Order",
{Custom Order( {"1", "2", "3", "4", "5", "6", "7", "8"} )}
),
Set Values(
{"1", "1", "1", "1", "2", "2", "2", "2", "3", "3", "3", "3", "4", "4",
"4", "4", "1", "1", "1", "1", "2", "2", "2", "2", "3", "3", "3", "3",
"4", "4", "4", "4"}
)
),
New Column( "X1",
Numeric,
"Continuous",
Format( "Best", 12 ),
Set Property( "Coding", {-1, 1} ),
Set Property( "Design Role", DesignRole( Continuous ) ),
Set Property( "Factor Changes", Easy ),
Set Values(
[-1, -1, 1, 0, 1, 0, -1, 0, 1, 0, -1, 1, -1, 0, 0, 1, 1, 0, -1, 1, -1, 1,
-1, 1, -1, 1, 0, -1, 0, 1, 0, -1]
)
),
New Column( "X2",
Numeric,
"Continuous",
Format( "Best", 12 ),
Set Property( "Coding", {-1, 1} ),
Set Property( "Design Role", DesignRole( Continuous ) ),
Set Property( "Factor Changes", Easy ),
Set Values(
[-1, 0, 1, 0, -1, 1, 1, 0, -1, 0, -1, 1, 1, -1, 0, 1, -1, 1, 0, -1, 0, 1,
1, -1, -1, 0, 1, -1, 0, 1, -1, 1]
)
),
New Column( "X3",
Numeric,
"Continuous",
Format( "Best", 12 ),
Set Property( "Coding", {-1, 1} ),
Set Property( "Design Role", DesignRole( Continuous ) ),
Set Property( "Factor Changes", Easy ),
Set Values(
[-1, 0, 1, 0, -1, -1, 1, 0, 1, -1, 1, 0, -1, 0, 1, -1, 1, 1, -1, 0, 0, 1,
-1, -1, 1, 1, 0, -1, 0, -1, 1, 1]
)
),
New Column( "X4",
Numeric,
"Continuous",
Format( "Best", 12 ),
Set Property( "Coding", {-1, 1} ),
Set Property( "Design Role", DesignRole( Continuous ) ),
Set Property( "Factor Changes", Easy ),
Set Values(
[1, -1, 1, 0, 1, 1, 0, 0, 1, -1, -1, 0, 1, 0, 1, 1, -1, -1, 0, -1, 1,
-1, -1, 0, 1, 0, 0, -1, 1, -1, 0, 1]
)
),
New Column( "X5",
Numeric,
"Continuous",
Format( "Best", 12 ),
Set Property( "Coding", {-1, 1} ),
Set Property( "Design Role", DesignRole( Continuous ) ),
Set Property( "Factor Changes", Easy ),
Set Values(
[1, 0, -1, -1, -1, 0, -1, -1, 1, 1, 1, 0, -1, 0, 0, 1, -1, -1, 0, 1, 1,
1, 1, 0, -1, 0, 1, -1, 0, -1, 1, 1]
)
),
New Column( "Y",
Numeric,
"Continuous",
Format( "Best", 12 ),
Set Property(
"Response Limits",
{Goal( Maximize ), Lower( . ), Upper( . ), Importance( . )}
),
Set Values(
[., ., ., ., ., ., ., ., ., ., ., ., ., ., ., ., ., ., ., ., ., ., ., .,
., ., ., ., ., ., ., .]
)
)
)