Realize there are two distinctly different approaches to the use of blocking and you will get differing opinions. Much depends on the specific situation for which you have provided very little context.
Here are some of my thoughts regarding blocking:
1. You can do Randomized Complete Block Designs (RCBD) or Incomplete Blocks Designs (AKA Balanced Incomplete Block (BIB)).
2. The model depends on how you assign the block.
a. If you have identified the noise and are specifically confounding it with the block (e.g., what is actually changing month-to-month?), you may want to treat the block as a fixed effect. If so, you can "create" the incomplete block by bumping the "number of factors" in your 8 treatment experiment to 5. Choose any column and sort the design. The first 4 runs are coded block -1 and the second are 1. This will be resolution III design. Y = Block+X1+X2+X3+X4+Block*X1+Block*X2
Aliasing:
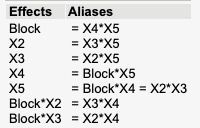
b. If you have not identified the noise (which is what appears to be your case) and therefore cannot assign the the noise to the block, the block should be treated as a random effect and used to increase the inference space and quantify experimental error. Y = X1+X2+X3+X4+Block(error)
Aliasing:
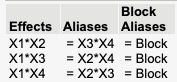
3. For covariates, you would need a continuous value for each lot. Some measure of the lot. There is much to consider for this (e.g., measurement errors, is the measure for the lot consistent within lot, etc.)
"All models are wrong, some are useful" G.E.P. Box