Hi, community,
I am new to definitive screening design and I am trying to get my head around the data I got recently. I had 6 factors, one of which was a categorical variable, and two responses.
I did an 18 run DSD which only identified 3 factors to be active. The second stage for determining the even-order effects identified all possible 2-factor interactions to be included in the model for one of the responses, even though only the intercept was significant.
The even-order effect estimates for the other response only included statistically significant 2-factor interactions and quadratic factors.
I have been reading through the heredity and hierarchical principles but still, I am not able to identify the reason why some of the statistically insignificant interactions and quadratic effects are still included in the model for one response and not for the other.
Any help will be greatly appreciated.
I have tried to include a screenshot of the fit definitive screening design I am referring to.
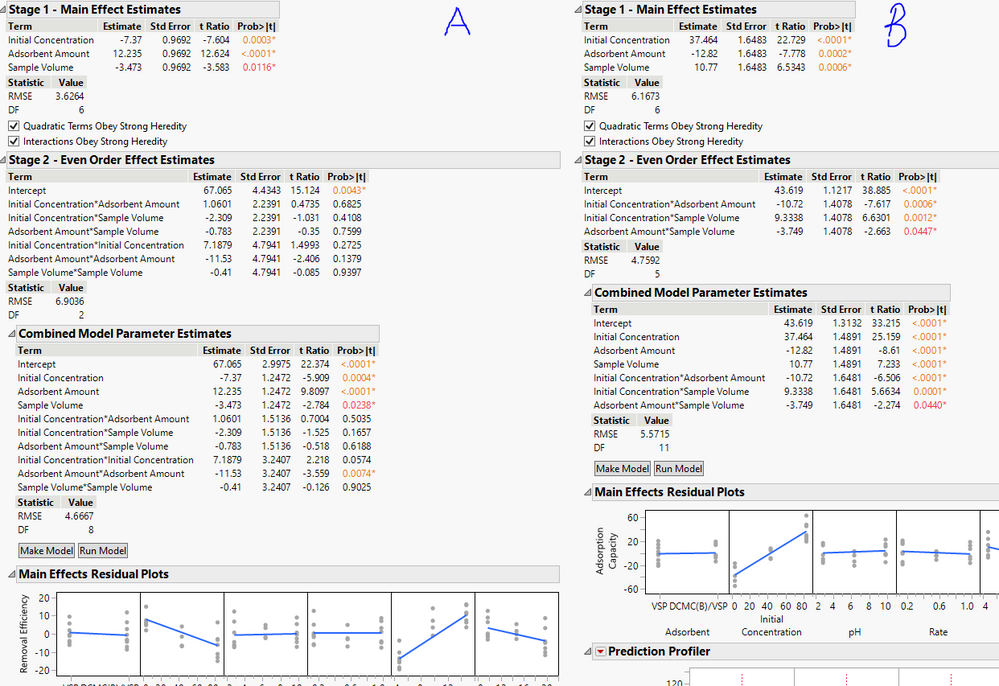