Hi community,
I'm seeking to gain knowledge on what factors influence the force required to push on an inhalator mouthpiece to an inhalator.
I have 8 boxes (A through H), each containing 1 inhalator and 12 mouth pieces (A1 to A12, B1 to B12, and so on). There are 3 types of inhalators across these 8 boxes (3 brown + 3 green + 2 red), but all the 96 mouthpieces are supposed to be identical.
It is unknown if we can expect variation in push-on force from "inhalator type", "inhalator box" or even "mouthpiece box" or a combination of these.
Moreover, the mouthpiece can be put on at various angles and the requirement for the test sequence is to try out 8 different angles.
My question is how best to set up a Custom Design in JMP to test the possible influence of these factors. We are commissioned to do 96 runs, which is a lot, but can be executed in a day. I think the original idea was to test inhalator A with all its mouthpieces (A1 to A12), B with all its mouthpieces, etc., which would amount to 96 runs, but I believe we can do better with DOE.
What I've done so far is to create a data table of all possible combinations of inhalator and mouthpiece, 768 in total (example of a row: inhalator from box C, which is a brown type, with mouthpiece G5, see screenshot). From this table I've tried to create a custom design with Inhalator (A, B, ..., H), Mouthpiece Box (A, B, ..., H), and Inhalator Type (Brown, Green, Yellow) as Covariate factors and Angle as a Discrete Numeric factor with 8 levels (0, 45, 90, ..., 315).
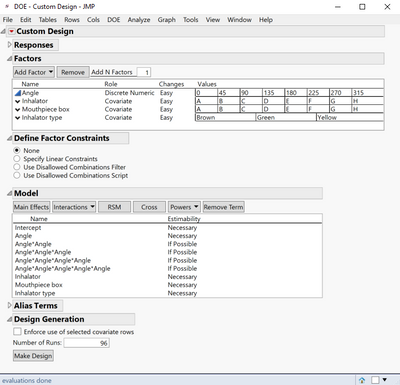
When trying to make the above design with 96 runs, JMP thinks for a little and then states that "The selected terms in the Model outline are linearly dependent on the previous terms. Please press the Remove Term button and try again."
It helps if I remove Inhalator as a factor, and I suspect this is were I'm going wrong? Can't I have a design that models both box-to-box variation and type-to-type variation for inhalators? Am I down the wrong path and have to rethink the DOE setup altogether?
All help is appreciated, thanks in advance!
I'm running JMP 14.2.
/Morten