This is an interesting question. To compare the prediction variance profiles for the two designs, I prefer to look at the Fraction of Design Space Plot. I have a D-optimal, I-optimal, and an A-optimal design shown for your situation:
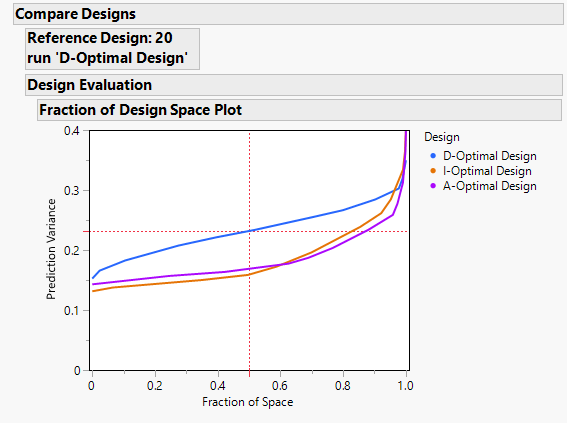
This shows that although the D-Optimal design does slightly better at the edge of the design space, the I-Optimal design is far superior in terms of the prediction variance. The A-optimal is interesting, but is also worse than D-optimal at the VERY edge of the design space. So how much of a gambler are you that the optimal actually occurs at the edge? And if you are very confident of that, why would you not center your design at that location instead? Further, why go to +/- 20% if you "know" the optimal is at an edge in the first place?
I-optimal designs create a design with minimum integrated variance. Think of the FPS plot shown above. I-optimal will keep the area below the curve at a minimum. A-optimal designs have the effect of minimizing the variance of the parameter estimates which leads to good prediction variance properties, just not optimal. I don't know of any design criteria that tries to minimize the prediction variance right at the boundary of the design space. There probably is something out there that will do that (or can be shown to do that -- I would not be surprised if D-optimal designs actually do that). It just seems to me that a design that does that would have limited practical use.
Dan Obermiller