Video was updated May 2024.
When product reliability at use conditions is high, accelerated life tests (ALT) are necessary to reduce test time. By testing parts under more severe conditions, the failure time is much lower, so testing can be completed in a reasonable time. Then, the accelerated failure data can be used to predict product reliability at low stress.
See how to:
- Understand the principles of ALT
- Use constant Arrhenius and Power Law constant stress models to predict lifetimes at use conditions
- Use a step stress approach to reduce test time even more and still predict reliability at low stress
- Create a safe operating area (SOA) from accelerated life data for two factors
Some background on Accelerated Life Tests:
- Mean Time to Failure – An average failure time for a non-repairable product.
- Response Surface Methodology - Way to explore relationships between several explanatory variables and one or more response variables.
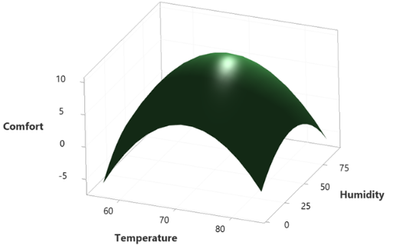
- Arrhenius Equation or Model - Describes the relation between the rate of reaction and temperature for many physical and chemical processes. The equation relates the dependence of the degradation mechanism on absolute temperature (T) and therefore, the time to failure. Time to fail ~ exp(Ea/T) where Ea is the activation energy. Can be used to estimate reliability parameters as temperature changes.
- Power Law Model - Equation that gives the dependence between failure time and the stress, where the failure time is a function of the stress to some exponent (or power). Time to fail ~ stress^n where n is an exponent.
- Censoring - Censoring a datapoint means that the datapoint is excluded as a failure time but still included in the analysis as the operating time of the part. Right censoring – stress on the part was stopped before the part failed. Interval censoring – failure of the part occurred within some known time interval. Left censoring – the part failed at some point before the start of the test. Left censoring is not common in industrial experiments.
Below is a demo on stress testing not covered at the 2024 webinar. It may be useful for people needing to get results even faster than when modeling constant stress. To use it you must be confident that 1) the stress doesn't get so high that the failure mode changes and 2) that a changing stress has no additional effects.
Questions answered by Jerry Fish @JerryFish and Charlie Whitman @charlie_whitman during the live webinar demo, plus some answers from previous demos.
Q: What happens if your data show slopes are different for different stress conditions.
A: That can be a problem. Remember, when we're extrapolating to use conditions and the slopes are different, that assumption of constant shape factor has been violated. So, there are some sophisticated ways to address that. For example, suppose at your highest stress you're seeing that the shape factor is somehow very different than the lower stresses. In that case, you could just exclude the higher stress data. Lots of times if the if the slope is changing, so the shape factor is changing, that suggests, but doesn’t necessarily mean, that you are failure mechanism is changing. And you want to avoid that. So, you might exclude that data and analyze it with the lower stress and then do your extrapolation, that's a problem. You may need to go to some even lower stresses because you want that shape factor to be constant so you can make your predictions with confidence.
Q: Are quantile lines always straight?
A: The lines will be straight because the plot has been scaled appropriately for the chosen model, like for the Arrhenius model in the example in the demo.
Q: How do you decide to use lognormal?
A: You can compare fits of a number of distributions, decide which fitting best, and go with that model. Or you may have historic info that says part fails using a particular distribution.
Q: Why do we use three different temperatures?
A: It is so you can detect "curvature" in the Temperature vs. Life plot. If you only use 2 temps, you are assuming a straight line, and you'll never know whether curvature affected your results.
Q: Should ALT testing be considered equivalent to natural aging over time?
A: For ALT, you are assuming that the acceleration doesn’t change the failure mechanism you would see under normal usage conditions.
Q: Are ALTs representative of real-world situations?
A: Yes. They are used all the time when it would take too long to get results under standard operating conditions. The key is that the accelerating factor results in the SAME FAILURE MODE as if you were running at standard conditions. It depends on what is meant by representative. Although, they are not representative of real life conditions, they are representative of how reliability studies are conducted.
Q: On the Arrhenius scatterplot, it feels like 'extrapolating beyond the bounds of the data", a no-no in regression. Is that true?
A: It is, kind of, but it’s done in a different context than regression. In the case of reliability, you’re trying to find the distribution that best fits the failure probabilities represented by the data. You don’t have data from the later failure times, but knowing the earlier failures may do a good enough job modeling the distribution. On top of this, you’re typically interested in the failure probabilities at these earlier times. So you may be extrapolating, but the confidence intervals are not as wide as those found when extrapolating beyond endpoints in regression.
Q: Can this Fit Life by X, Arrhenius analysis be done with only one data point at each temperature setting?
A: Only if you plan on making some assumptions about the failure distribution and acceleration factor and use Bayesian methods.
Q: For Fit Life by X, Arrhenius, how many stressed conditions do you need to extrapolate the in-use condition?
A: Mathematically I think you would only need 2. But as with any statistics problem, more data is always better, giving tighter confidence bounds, etc. So it becomes a tradeoff against resources needed for the test.
Q: For Fit Life by X, Arrhenius, if temperature was modeled as ordinal, would this demo still work or does the Fit Life by X analysis need continuous data?
A: No. The assumption with these models is that X is either continuous or nominal.
Q: For Fit Life by X, Arrhenius , will you briefly explain how JMP is calculating the Lower and Upper Confidence Bounds for the predicted lifetimes?
A: JMP is using maximum likelihood to estimate the parameters for the selected distribution. From that, you calculate the confidence intervals for the quantile of interest.
Q: I know its preferable to obtain data at 3 different temps, but would it be possible to use just one?
A: No. It's like fitting a line through a single point. You need at least 2 points to fit the line.
Q: Can this Power Law model (also called Log Model or Voltage Model) be applicable for destructive samples?
A: Everything Charlie has talked about to this point assumes a single measurement per sample. He has not discussed repeated measurements.
Q: When do you use the Power Law vs Arrhenius?
A: In practice, many use Power for voltage and Arrhenius for temperature. If it is a stressor with which you are not familiar, consider going to the literature to find out what has been used historically. You can also do a check. See below.
Q: How would 'bad' data behave in these analysis? Say we went too aggressive and induced another failure mode. Are there signatures in the model that would reveal this, before calling in subject matter experts or inspecting the parts?
A: You would likely see observations falling outside of the confidence bands at some point.
Q: Do you always use the Lognormal transformation for the Arrhenius model and the Weibull for the Power Law model?
A: No. You can use any distribution you want.
Q: What does the shape factor mean?
A: It’s a generic term that dictates how the shape of the distribution changes. Think of it as a parameter that controls a given characteristic of the distribution.
Q: Is it normal to validate extrapolated ALT testing with longer aging studies at smaller stress ramp rates?
A: Validating (or confirming) predictions is always a good idea, whether in ALT, or general DOE analysis, or even regression models on observational data. To paraphrase George Box, "All models are wrong, some are useful." The only way to know if your model is "useful" is to run some confirmation tests.
Q: Does JMP have a sample size calculator for this to help us know how many samples to test at each condition to get a desired prediction interval?
A: Under the DOE menu, Special Purpose, there is an ALT Designs option.
Q: For Step Stress, when will you consider using a lognormal curve - when it's not linear or parabolic?
A: Keep in mind you’re modeling lifetime distributions. Anything that has a skewed distribution (more failures at longer times) would benefit from being fit to a non-normal distribution (like the lognormal).
Q: How do you deal with interval censoring? For example, how do you code it in the data table to input it in the reliability analysis platform?
A: You'll have 2 censor columns. The first will list the lower intervalue, the 2nd will be the upper. Enter both Censor columns in the inputs, and JMP knows you are talking about Interval Censoring.
Q: If I have two products and want to see how they do under two stresses conditions (time and voltage like what Charlie is showing), do you recommend adding product as a covariate or perform different analysis by each product?
A: I tend to favor adding it as a covariate so I can look at the interaction between product and accelerating factor conditions (e.g., different products may have different spreads of their distributions).
Q: For the Survival Fit platform it does not seem you can get some of the nice output from the Fit Life by X platform such as the Distribution plots. Is this true?
A: Correct. You can save the predicted values to the dataset and plot them in Graph Builder to get nice graphical output.
Q: Would you still use the ALT platform if you are temperature accelerating degradation of mechanical properties of an elastomer, and want to extrapolate prediction of properties to lower temperatures/longer times, or would you just use the DOE platform here?
A: Using the ALT platform is probably preferable.
The analysis typically uses nonlinear models. For more information about nonlinear models: