To clarify @dale_lehman's example, lift is a factor. Lift equal to 4 means that 4 times as many targets were conditionally predicted by the model than would be predicted by the marginal probability. See this slide from our copyrighted JMP training materials provides an example:
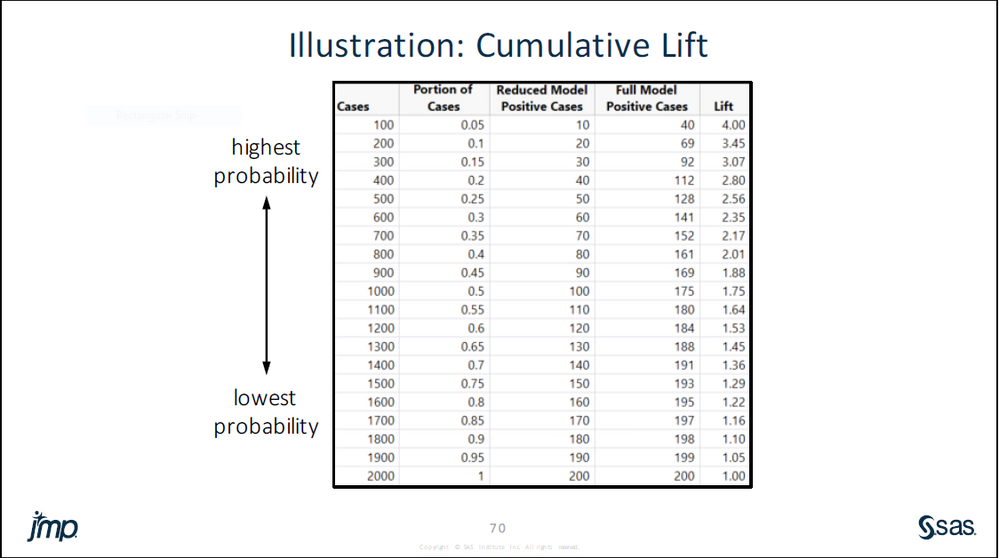
The reduce model is the marginal probability, 0.1 in this case. The full model is conditioned on the predictors or factors.
Yes, it only drops to 1 because it is a factor, comparing the true predicted targets to the marginal targets.
Also, the domain is always from the top or left of the axis. So a value of 0.4 means the top 40% of the cases ranked by predicted probability.
Note that there are different definitions of lift in use.