Hello,
please help me to understand this - working with large datasets with continuous variables - most of the times I use models to a binary outcome (alive/dead).
Many of my continuous variables are not normally distributed, hence I usually approach the test by Fit Model (Y=outcome, model effects - weight, nominal logistic). The distribution of the primary variable - weight: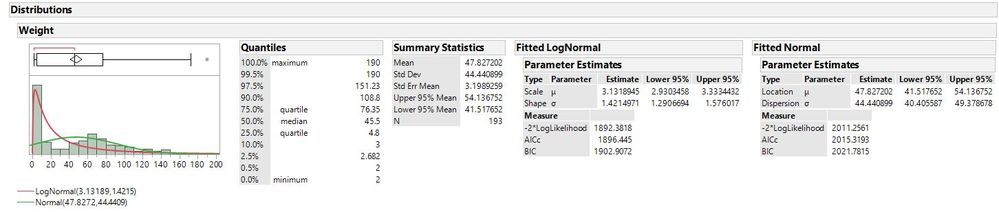
The outcome of the logistic model looks like this:
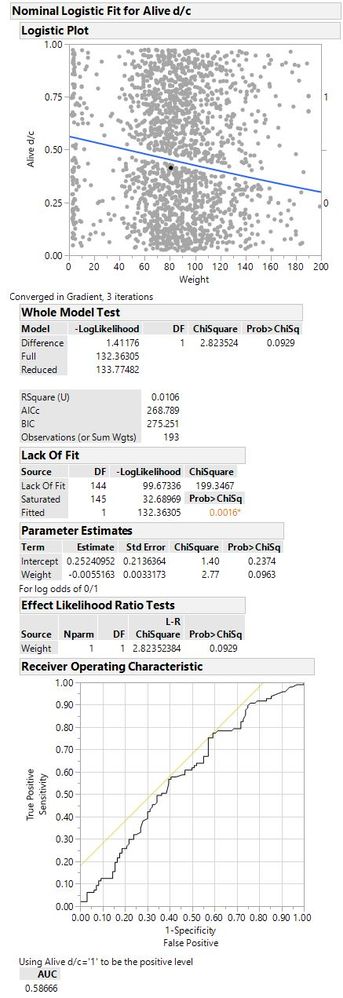
Not really a nice model, and I tried different transformations, but the distribution of my variables is a lot of time non-normally or logarithmic.
So, for better understanding for the audience, I turn the reponse and variables around: Fit Y by X (Y: Weight, X: outcome) and doing non-parametric Wilcoxon test - giving me significant result (p<0.05), while my logistic regression with a bad fit test shows a trend but no significance (p=0.09).
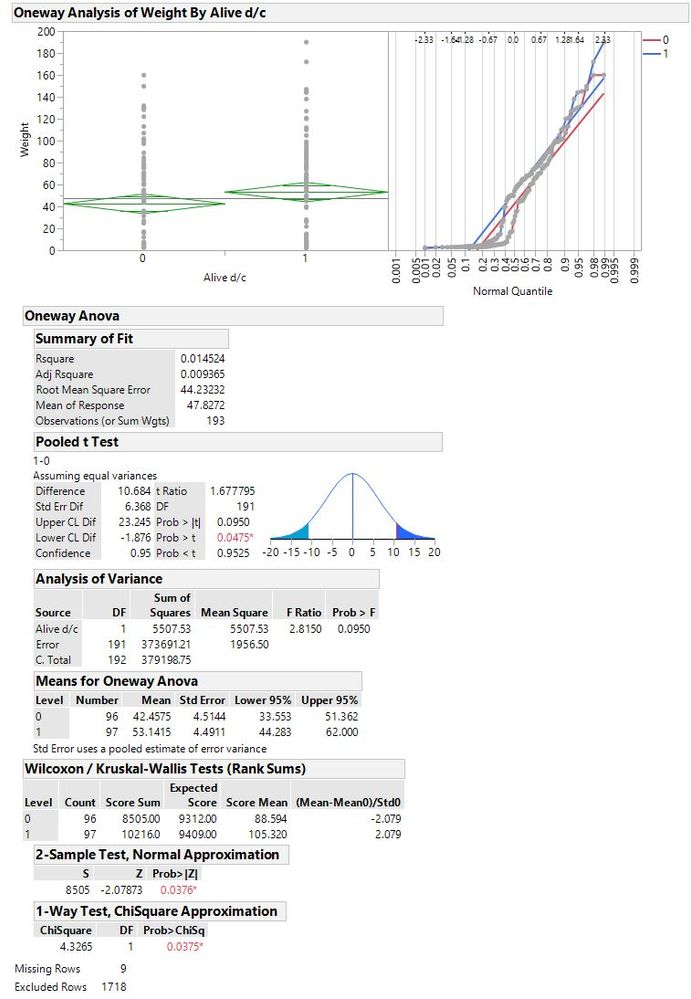
So what do ?
The response is the clearly the outcome (Y), so should I stick to this test even when the lack of fit test is positive. Many readers are not used to logistic regression and rather prefer quantile charts , but this would require the outcome to be weight (Y).
Which test would be legit to use ?
Can I overcome the lack of fit in the logistic regression ?
Which p value can I use at the end ? - is there a way to define OR for non-parametric test results ?
Thanks a lot, Marc