JMP version 17
I have a question about the fit model pValues.
When you put interactions into the fit model and then perform the "stepwise" personality. You can remove extraneous interactions using the pValue threshold. See below:
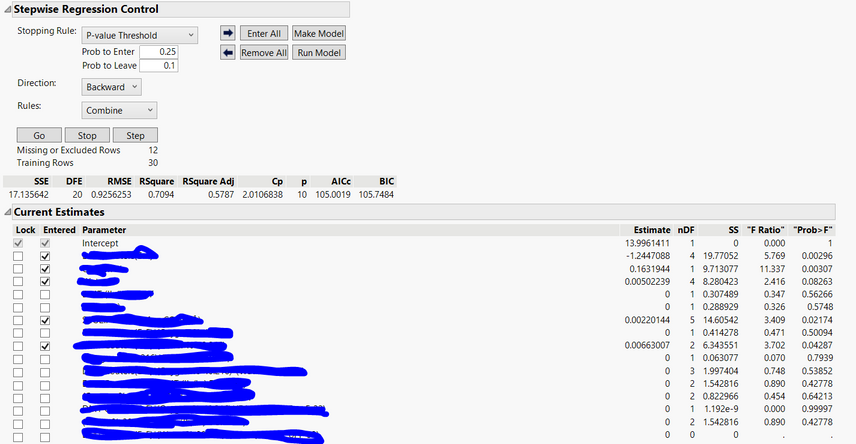
Here I have the regression set to remove any interaction that has a Pvalue more than 0.1. However, when I go to make the model with "standard least squares" personality and "Effect Leverage" Emphasis, I get Pvalues above 0.1 for the same interactions that I just saw in the Regression Control. Why? See below:
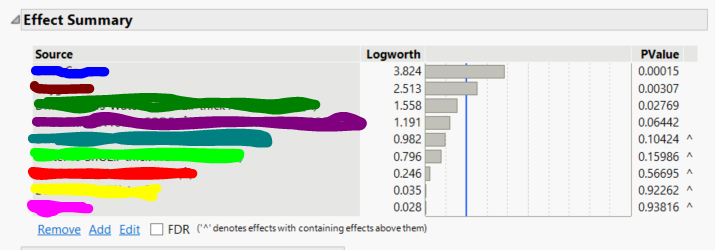
Additionally, when you remove the worst of the interactions (based on highest pValue), some of the other interactions get better pValues--to the point of becoming statistically significant, when before the deletion, they were not. I assume that the interactions removed do have some effect and that effect must then be redistributed to the other variables, but I don't understand why it makes such a big difference or what is really going on. See below (yes, matching colors indicate the same interactions in the two "effect summary" images).
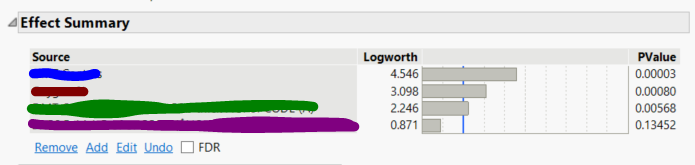
I am a chemist and I have only the most basic of knowledge of statistics. So, this may be a simple enough answer for an experienced user, but I feel like I am running blind with these models. At what point do you actually know that your model is good? When do you know to remove certain variables? What is the reasoning behind removing certain interactions over others-purely based on the statistics?
Any help would be appreciated. Thank you.