Level: Intermediate
Job Function: Analyst / Scientist / Engineer
William Q. Meeker, Distinguished Professor of Statistics, Iowa State University
Christopher Gotwalt, JMP, Director of Statistical Research and Development, SAS
The development of theory and application of Monte Carlo Markov Chain methods, vast improvements in computationally capabilities and emerging software alternatives, especially in JMP, have made it possible for the wide use of Bayesian methods in reliability applications. Bayesian methods, however, remain controversial in reliability (and other applications) because of the concern about where the needed prior distributions should come from. On the other hand, there are many applications where engineers have solid prior information on certain aspects of their reliability problems based on physics of failure or previous experience with the same failure mechanism (e.g., imprecise knowledge about the activation energy in a temperature-accelerated life test or about the Weibull shape parameter in analysis of fatigue failure data). In such applications, the use of Bayesian methods offers an appropriate, if not compelling, compromise between assuming that such quantities are known and assuming that nothing is known. In this talk, I compare the use of Bayesian methods with traditional maximum likelihood methods for a set of examples including the analysis of field-failure data, accelerated life test data and accelerated degradation test data.
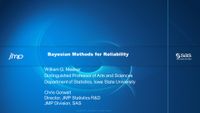
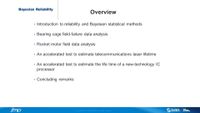
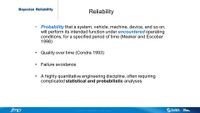
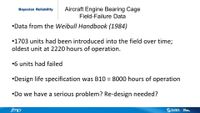
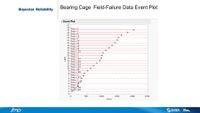
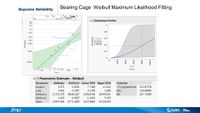
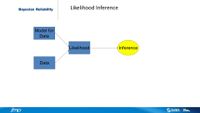
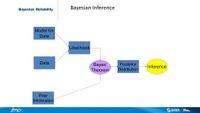
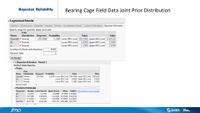
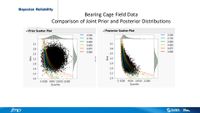
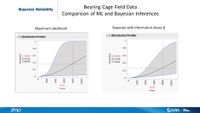
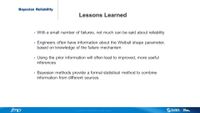
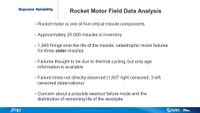
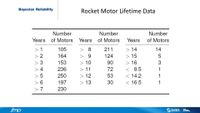
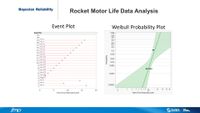
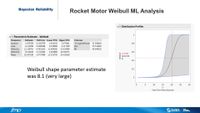
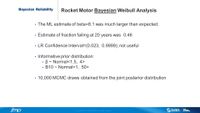
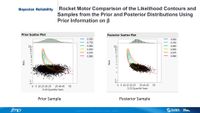
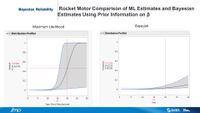
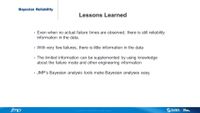
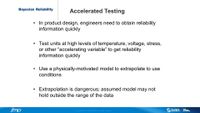
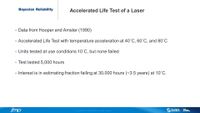
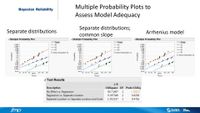
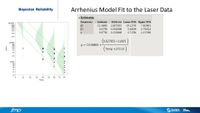
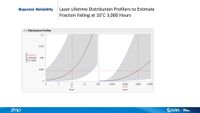
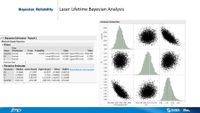
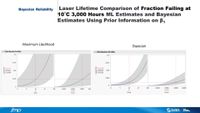
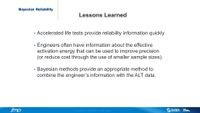
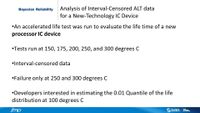
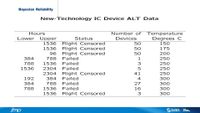
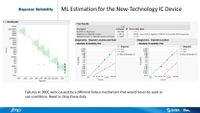
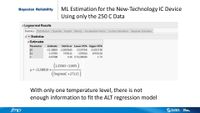
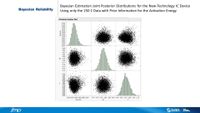
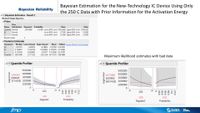
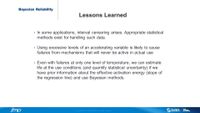
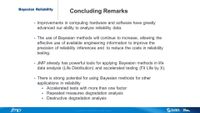
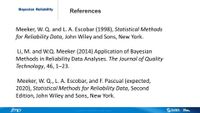