Level: Intermediate
Tony Cooper, Principal Analytical Consultant, SAS
Sam Edgemon, Principal Analytical Consultant, SAS
Machine learning is about creating predictive models. If the target (aka response) is nominal, then a good model will maximize true positives and minimize false positives. Designed experiments will use many of the same analytical algorithms, but the focus is on causality and the appropriate recipe for success. JMP users may be more used to this paradigm, and often look at models and ask, “Why is the prediction so high? How do we improve it? What is important about the business?” These questions are asking predictive models to become explanatory and therefore can be hard to answer; issues such as restricted ranges on the variables and multicollinearity can make it difficult to go from a predictive model to an explanatory model. Further, numeric summaries of models do not encourage subject matter experts to ask questions. The difference between a predictive model and root cause analysis can be overlooked; yet we know correlation does not imply causation. The art of explaining data is getting lost in the push for advanced modeling techniques. The humble Profiler in JMP is a powerful tool for making models talk. This presentation reminds us that, even for a data miner, it is the Profiler and Graph Builder that will make the difference.
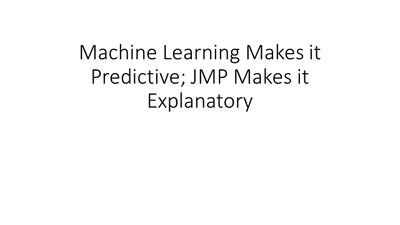
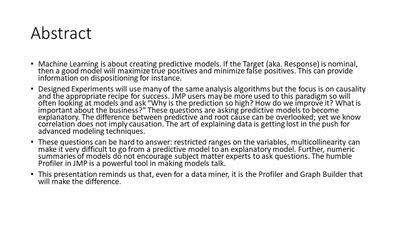
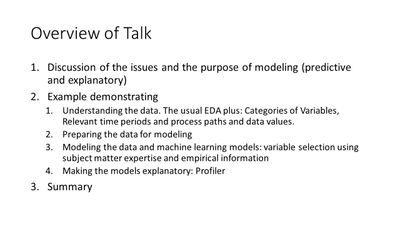
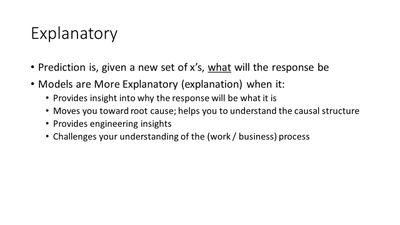
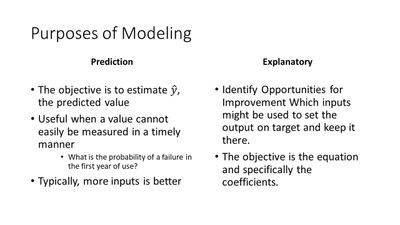
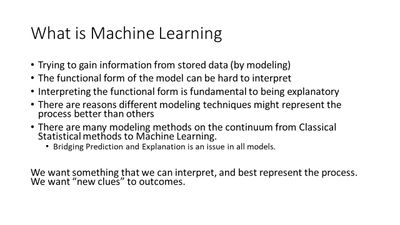
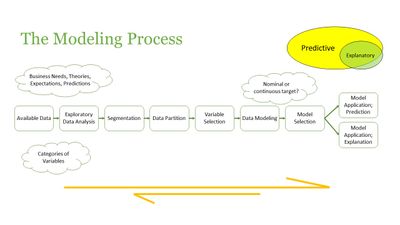
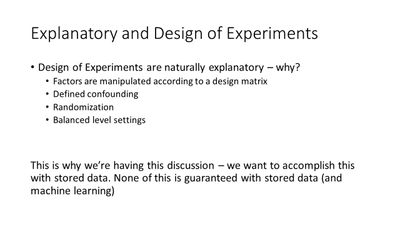
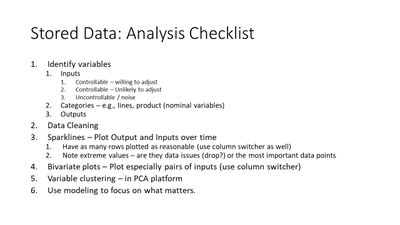
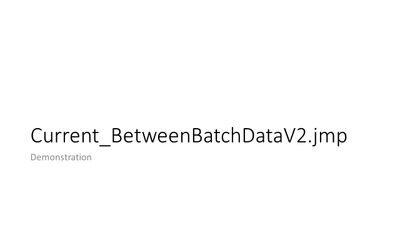
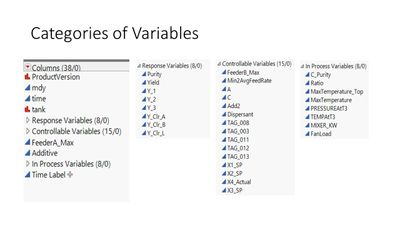
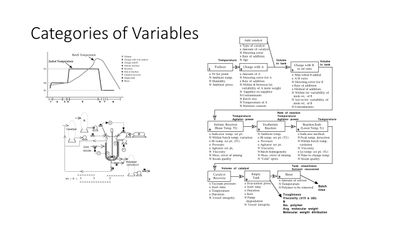
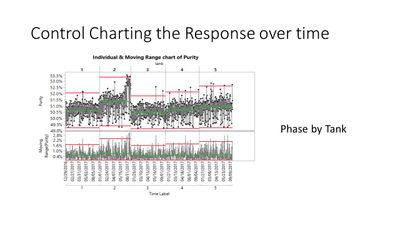
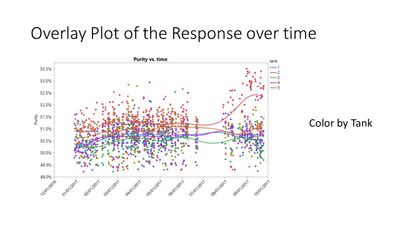
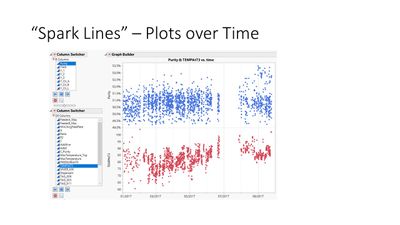
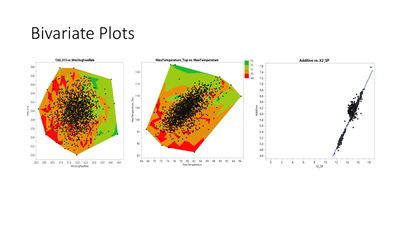
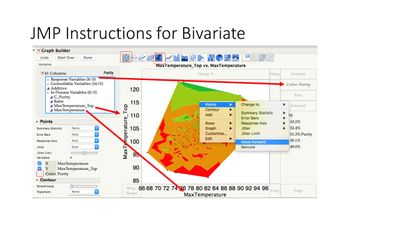
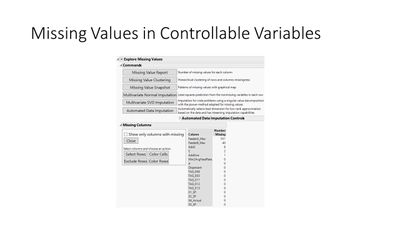
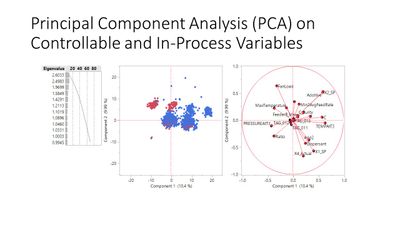
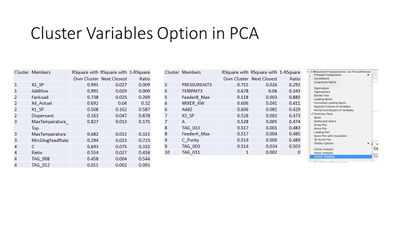
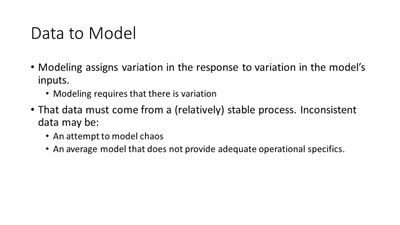
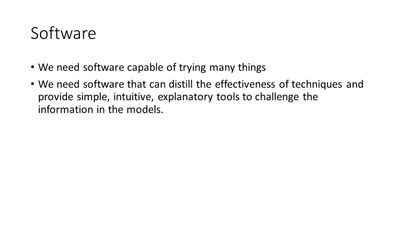
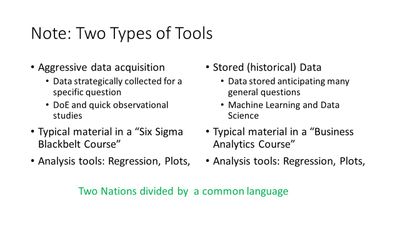
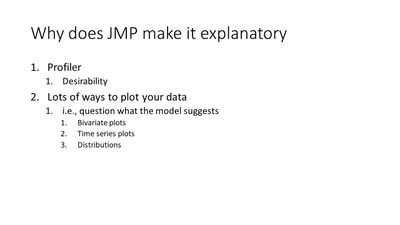
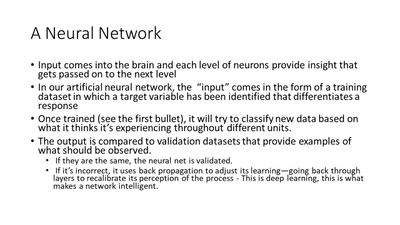
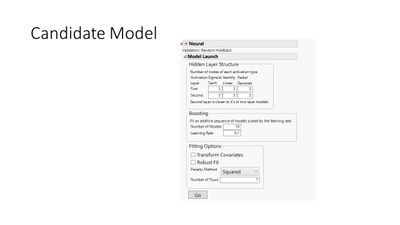
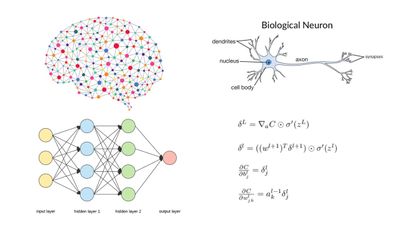
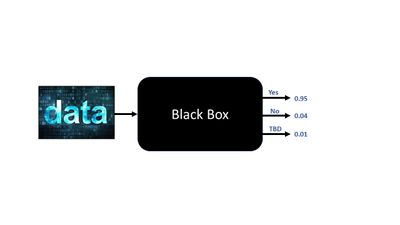
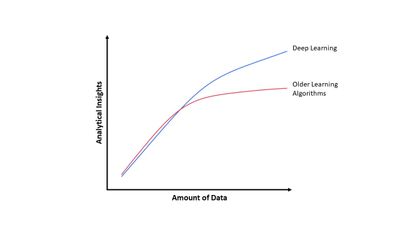
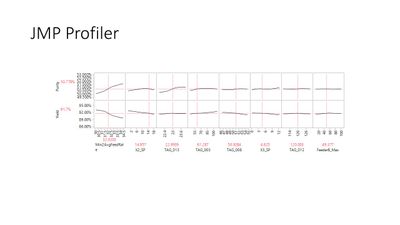