Level: Beginner
Karen Copeland, Owner, Boulder Statistics
Tarek Zikry, Technical Intern, SAS
Mia Stephens, JMP Academic Ambassador, SAS
For dichotomous classification models (good versus bad, negative versus positive, yes versus no, etc.), an ROC curve and the associated area under the curve (AUC) are often used to describe the ability of the model to differentiate the two groups. While this is a start, the ROC curve can be hard to understand, and the AUC can be misleading outside the context of the modeling problem. For some models, the overall misclassification rate is key; for other models, there is a cost associated with misclassifications. This cost can be very different for a false negative versus a false positive. We will discuss dichotomous models for prediction and their associated ROC curves, lift curves and alternative displays of model performance. We will also discuss methods for selecting optimal cutoffs for classification and measures of performance based on the specified cutoff. To facilitate the selection of the optimal cutoff for classification models and the calculation of performance metrics, we have developed an interactive and graphical JMP application. We will demonstrate this application with a compelling case study.
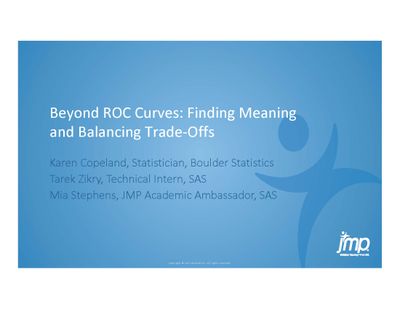
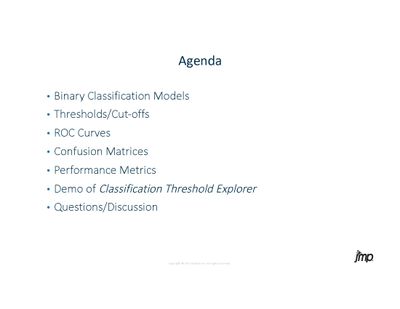
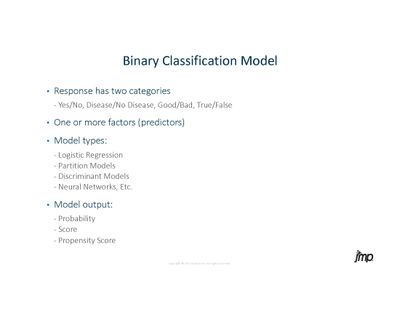
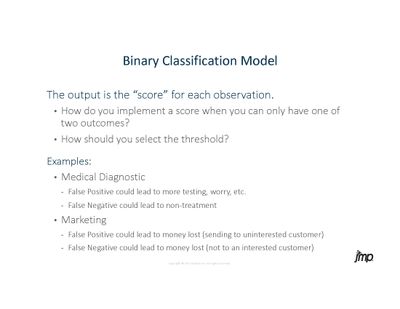
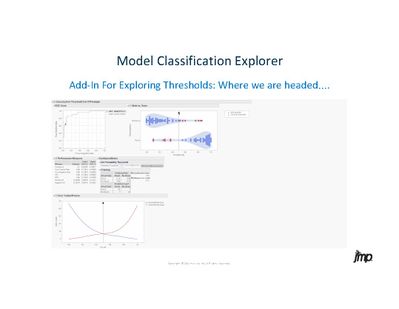
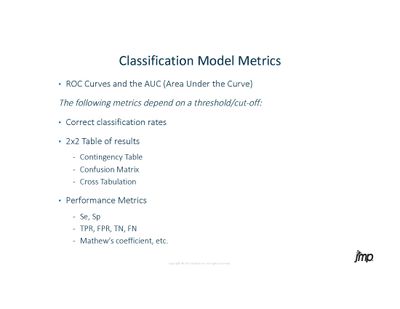
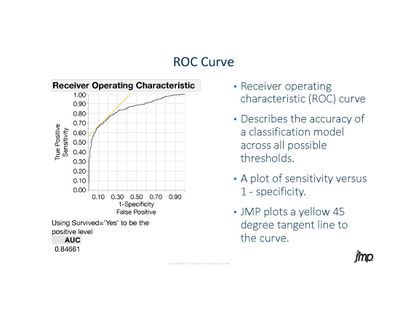
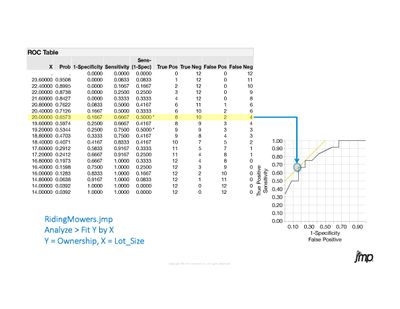
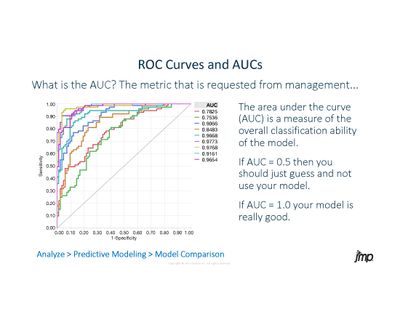
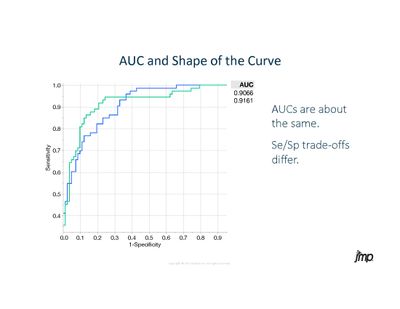
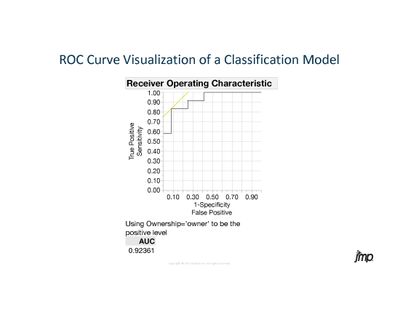
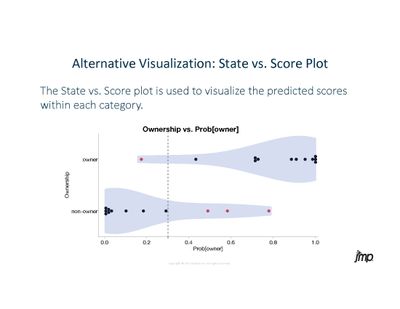
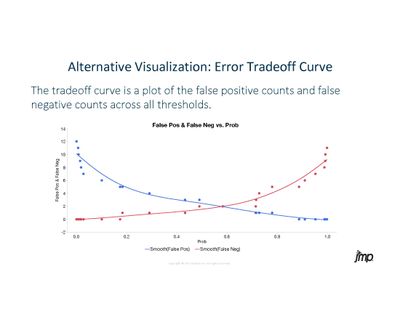
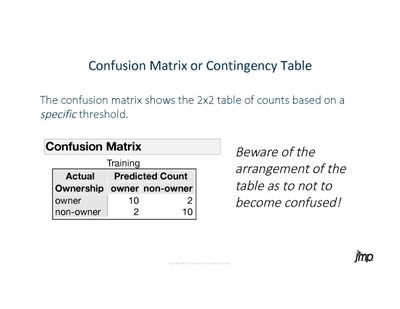
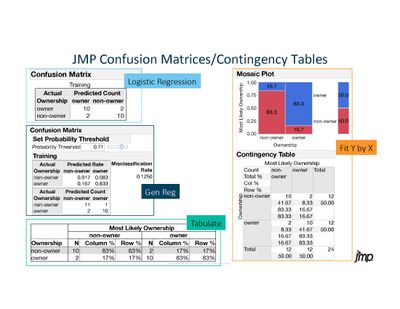
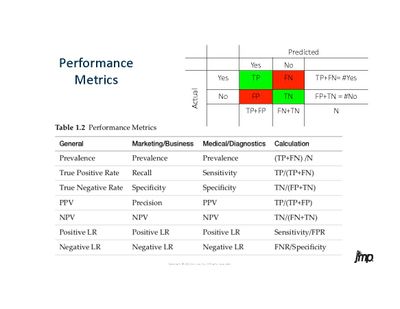
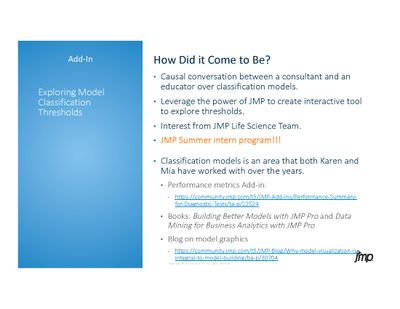
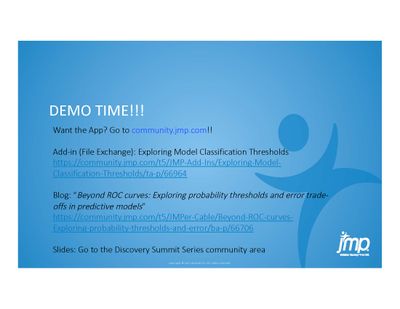
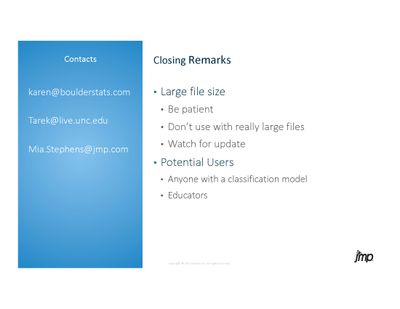