Sarah Battenfield, PhD, Hybrid Wheat Breeder, Syngenta
Abstract:
Many wheat breeding programs have focused on increasing bread wheat yield, but processing and end-use quality are critical components when considering its use in feeding the rising population of the next century. The challenges with end-use quality trait improvements include its high cost and seed amounts for testing, the latter making selection in early breeding populations impossible. Here we describe a novel approach to identify marker-trait associations within a breeding program using a meta-genome wide association study (GWAS) using JMP Genomics. This method combines GWAS analysis from multi-year unbalanced breeding nurseries, in a manner reflecting meta-GWAS in human disease marker discovery. This method facilitated mapping of processing and end-use quality phenotypes from advanced breeding lines (n=4,095) of the CIMMYT bread wheat breeding program from 2009 to 2014. Using meta-GWAS, we identified marker-trait associations, allele effects and candidate genes, and can select using markers generated in this process. Finally, the scope of this approach can be broadly applied in breeding-assisted genomics across many crops to greatly increase our functional understanding of plant genomes.
Introduction:
Meta-Genome Wide Association Studies (meta-GWAS) are commonly utilized in human genomic analyses, relying on allele replication over several studies to gain power. In plant breeding and research, these analyses are uncommon due to the ability to replicate genotypes over space and time. However, in an era of big data and when traits are cost prohibitive to phenotype, this method becomes an interesting alternative.
Methodology:
GWAS analyses have long been supported in JMP-Genomics. Easy to follow work flows are available for Q-K Analysis, or GWAS accounting for population structure and kinship.
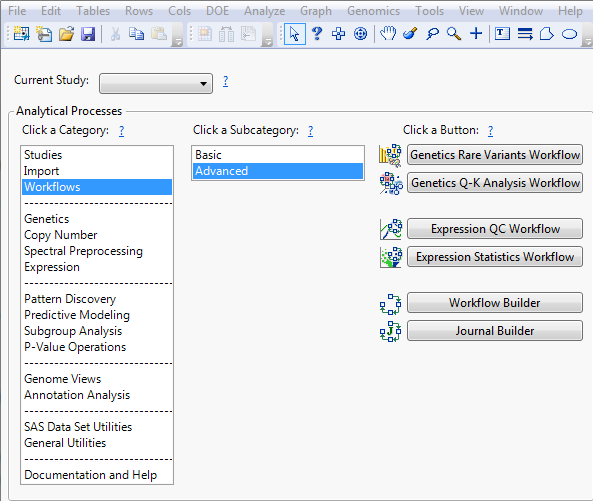
Figure 1: Q-K Mixed Model Workflow
The present research was to map loci impacting wheat quality traits, which were measured in a large applied wheat breeding program. Structure mapping populations for wheat quality are not common, since this testing would be very expensive, take many years and replicates, and require large amounts of seed. Since the focus of data generation was breeding for yield with wheat quality being expensive and serving as a secondary priority, the lines were tested in only one replicate in one year, crossing several years. This left no degrees of freedom for developing a standard Q-K mixed model. However, rich data was available to be exploited relying on allele replication in a meta-GWAS across years.
Harvest Year
|
Total in Yield Trial
|
Quality Tested & Genotyped
|
2010
|
4,956
|
250
|
2011
|
6,685
|
995
|
2012
|
10,196
|
850
|
2013
|
9,436
|
886
|
2014
|
7,672
|
1,114
|
Total
|
38,945
|
4,095
|
Table 1: Entries available across years.
Thus, the objective was to:
- Use large amount of breeder generated data in GWAS to identify QTL impacting processing and end-use quality in CIMMYT breeding program.
- Leverage large amounts of lines not replicated over years using meta-GWAS model.
First Q and K matrices were solved within the data for each year:
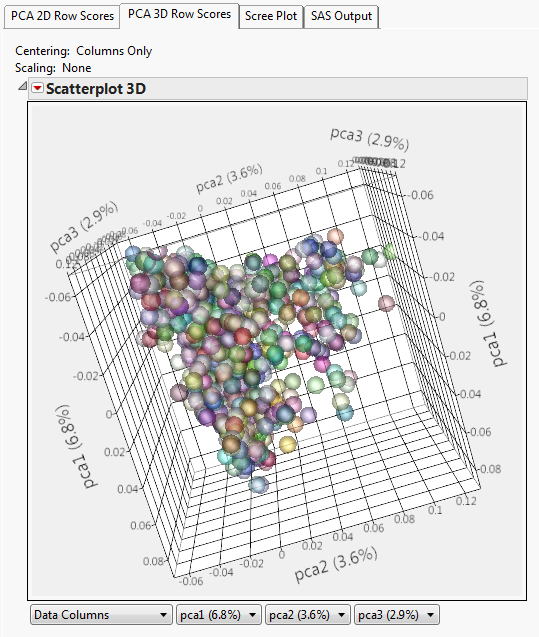
Figure 2: Q-Matrix Population Structure PCA
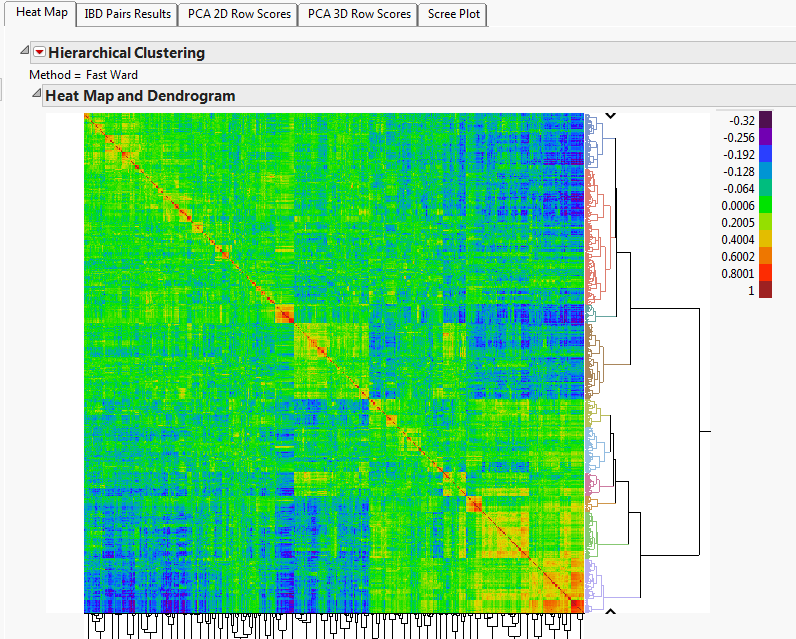
Figure 3: K-Matrix Cryptic Relationship Heat Map
Next the Q-K Mixed Model was solved within each year with application of FDR multiple testing correction:
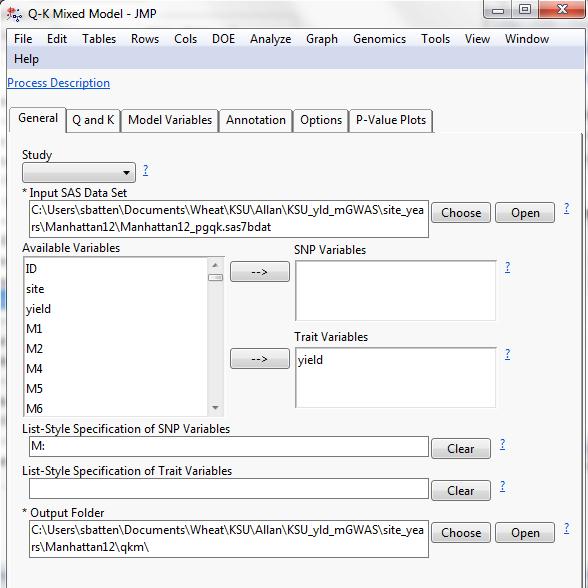
Figure 4: Q-K Mixed-Model
Now a meta-GWAS can be applied over all site years by running a GWAS Meta-Analysis inputting all (_qkm) files derived from individual Q-K Mixed Models. We used the inverse-variance, fixed effect model with FDR multiple testing correction option, resulting in an estimate and standard error for each marker studied, since effect of the marker was the desired outcome.
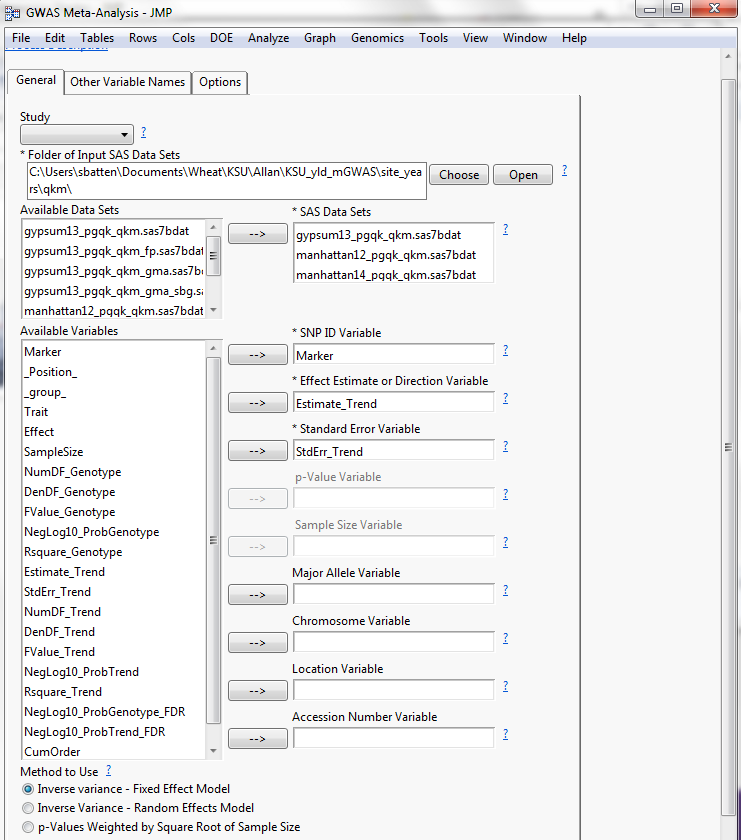
Figure 5: GWAS Meta-Analysis
Resulting in output of significant meta-QTL for wheat processing and end-use quality traits.
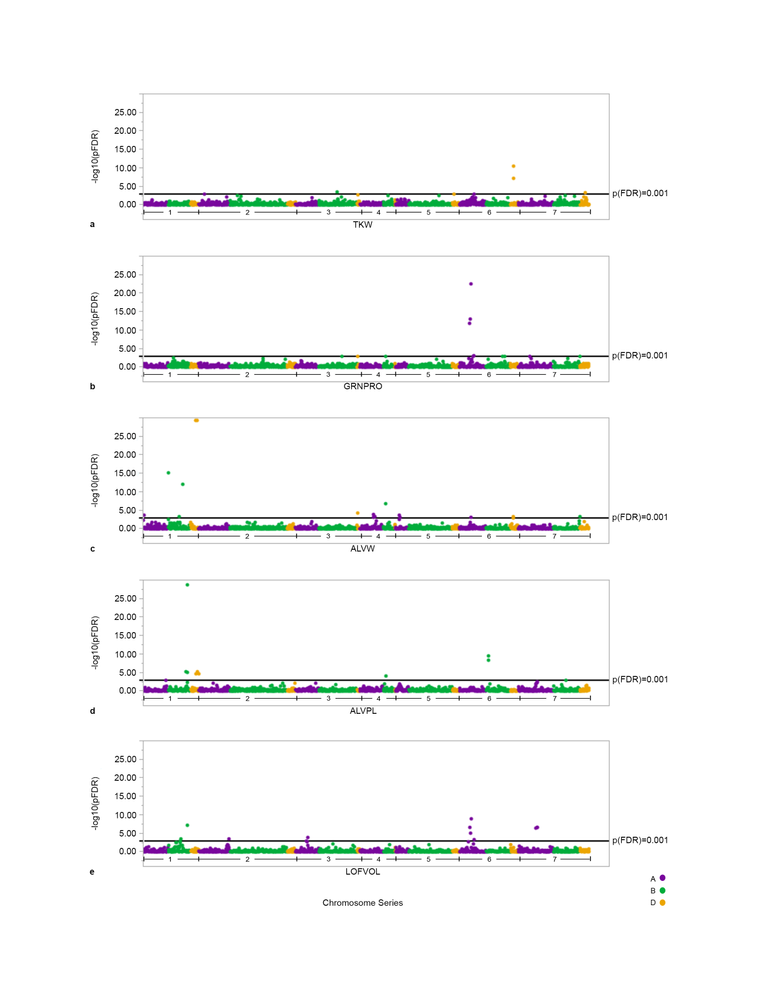
Figure 6: Manhattan Plots of significant meta marker trait analyses for several quality traits
Digging deeper, we wanted to know the effect of the QTL, rather than single SNPs, so the next step was to determine the effect of significant haplotypes. Note, haplotypes could be determined before mapping, and used as the unit of mapping, but wheat has a LARGE, not fully referenced genome, and this haplotype GWAS was not feasible at the time.
So, we reduced the genotype file to only the significant markers that were in similar regions and estimated the haplotypes by region and year.
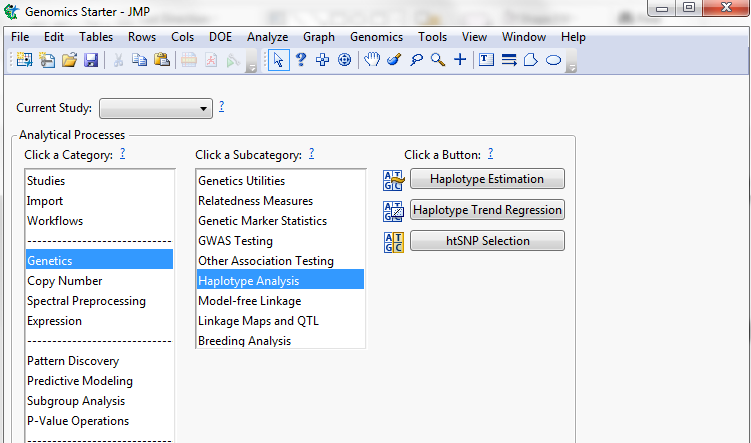
Figure 7: Haplotype Estimation path
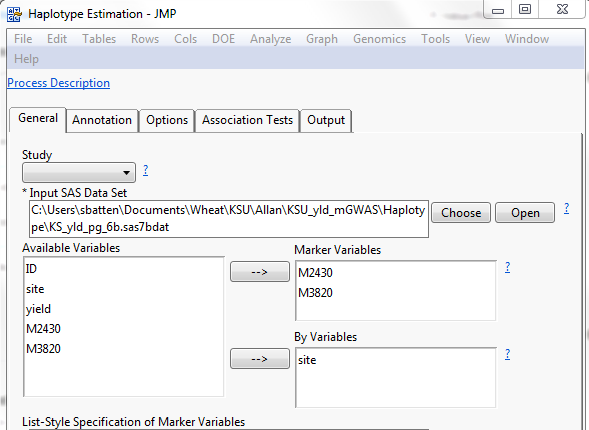
Figure 8: Haplotype Analysis setup
In the Haplotype Trend Regression Results, open the haplotype frequency estimates (_hfr) files. Look for clearly differentiated haplotypes which make up 90% of the material (the remainder could be errors in genotyping or very rare haplotypes). Take note of the haplotype number and allele calls for future reference.
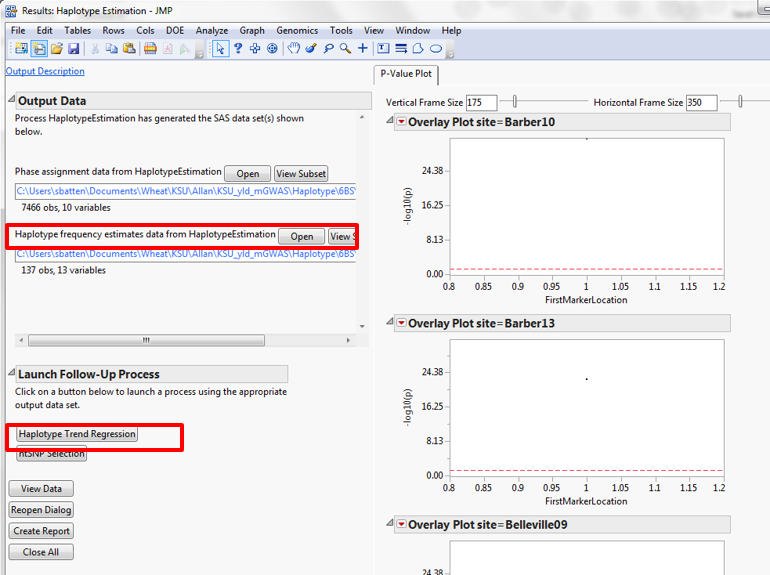
Figure 9: Haplotype analysis results window
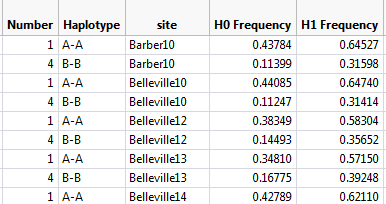
Figure 10: File needed (_hfr) that will be added on for meta-haplotype analysis
Then launch haplotype trend regression, which results in SAS output. Look for the haplotype number within the given site or year and append estimate and standard error in the (_hfr) file. Save file with new name for meta-analysis of the significant haplotypes.
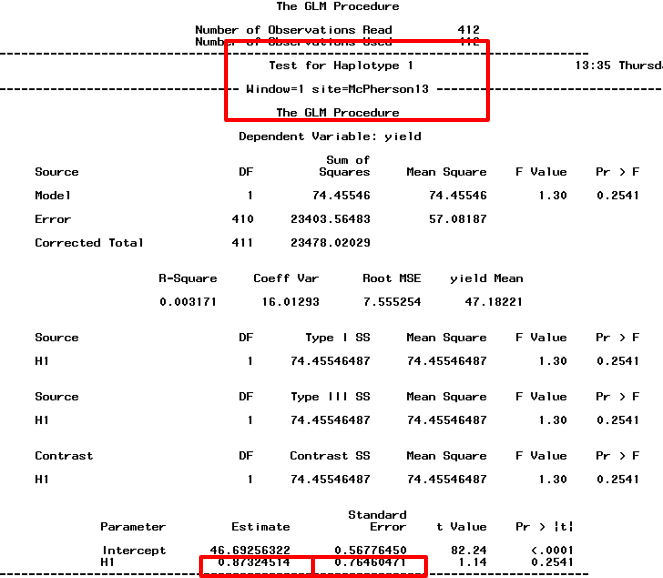
Figure 11: SAS output file of haplotype trend regression
Applying a meta-analysis over the significant haplotypes in all years and in all phenotypes we were able to see the effects of these QTL over all traits.
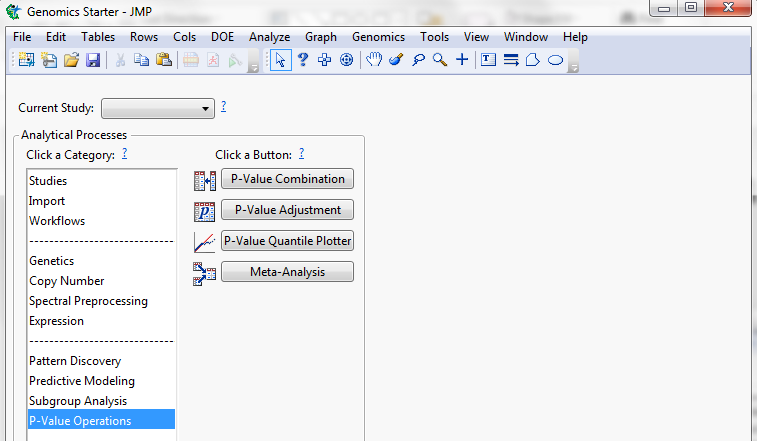
Figure 12: Path to haplotype meta-analysis
Thus, able to do candidate gene postulation by searching the literature for known and named QTL, BLAST search between haplotype boundaries and investigate genes likely to affect the target trait expression.
Conclusions
Using Meta-GWAS in JMP-Genomics:
- Methodology is supported to conduct meta-GWAS in JMP-Genomics. This was developed with JMP-Genomics team and improvements are coming online to better streamline the process.
- Mapping of expensive and economically necessary traits is possible in breeding programs without structured populations
- This allows use additional use of data already generated in breeding program
- Meta-GWAS allows De novo detection and derivation of markers and haplotypes for QTL currently present in breeding program
- Effect estimates determined are relevant to breeding program over several years