Decision trees use a classification or partition as the basis for a predictive model to explore patterns, uncover relationships, screen the most influential factors and make conclusions about a response.
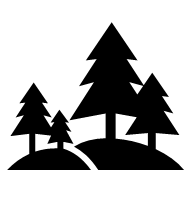
JMP® provides an interactive approach to creating decision trees with numerous options for optimization and interpreting results. View this Health and Life Science case study to see how tree based machine learning methods can be very good models to identify relationships between factors and a response.
Situation: As the research manager, you want to understand if there is a relationship between patient factors and diabetes classification.
Task: The problem is that there are multiple patient factors and not all of the relationships and factor interactions are fully understood.
Action: You use Decision Trees to create simple partitions then advanced Bootstrap Forest and Boosted Trees methods to find the best model.
Result: Using these Machine Learning techniques you compare the best models and identify the optimal values to minimize diabetes.
Next Steps: Your research is shared interactively with your colleagues and leads to more research which helps to improve patient health.
Predictive Models and Decision Trees.zip
You must be a registered user to add a comment. If you've already registered, sign in. Otherwise, register and sign in.