Hello!
I am struggling with interpretation of factorial design with 4 elements. Before I tried experiments with 2 elements, and results were straightforward.
Here in the attachment you can see my jmp dataset file with the experiment.
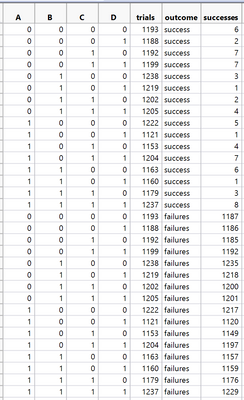
As a result of removing non-significant elements I came to a conclusion that only C and В are significant with negative effect.
Is my conclusion correct?
Rsquare value is very low however chisquare value for elements is significant.
Maybe the experiment is underpowered and needs bigger sample size?
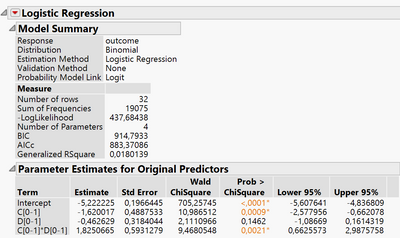
PS.
Sorry for newbie questions, I am still learning.
In general, trying to interpret different DoE experiments when I reduce the degree of factorial design from full to a degree of 2 or remove some variables from a model the effect of the same variable changes significantly sometimes to an opposite direction.
Do I need to look at misinterpretation rate to choose a model in this case?
Are obliged then to run a follow-up experiment with the winning variable, or you can just ship it? What is the approach here?
Can you please point me to an article or a book with a structured approach how to interpret results of binary DoE?
Thanks
Learning DOE