In this blog, I introduced a workflow for modelization of gene expression data in JMP Pro 17. Keep reading to see how to apply those steps.
Modelization
The Predictor Screening platform is useful for analyzing large data sets, in which hundreds or thousands of measurements on a sample are taken and require innovative approaches. For example, it can be used to help identify biomarkers from thousands tested in samples from patients with and without a condition to predict the condition. It can also be used to quickly screen the important biomarkers before doing any types of further analysis, such as modelization for reducing the complexity of the model and improving the accuracy of the predictive models.
Since the Predictor Screening platform uses a different methodology from Response Screening, it finds variables that drive large changes in the data by using a bootstrap forest model to screen for potentially predictors of your response.
Here we can see the outcome of ranked predictor (biomarker) contributions:
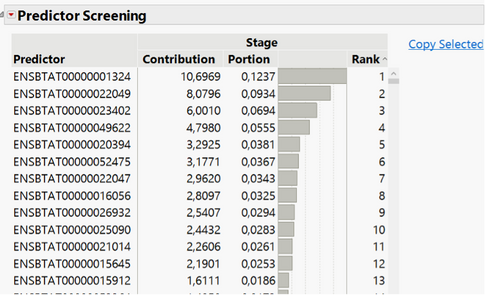
Frequently, gene expression profiles display similarity, which suggests some co-regulation; it is often beneficial for reducing the number of candidates that are highly correlated. The Cluster Variables platform performs dimension reduction on the number of input variables to be used in a predictive model. It reduces inputs by finding groups of similar variables so that a single variable can represent each group.
It incorporates principal component analysis (PCA) in the clustering of the samples Statistical Details for the Cluster Variables Platform. PCA is a tool for understanding both the relationship between many variables and some of the underlying effects by revealing which variables are behaving similarly. It also reveals which variables are unique among the important variables.
Below, I selected the 50 most important variables from Predictor Screening and used them in the Cluster Variables.

In the cluster summary, we see only five unique groups of variables with the most representative variable. Note that the number of clusters can vary from one analysis to another, since the variables picked from Predictor Screening can vary from one analysis to another.
In this example, in Cluster 1, we can put 23 variables together because they are performing similarly. From this group, we only must look at Variable -15645. This single variable explains 81.3 % of the variation in the first principal component that it is occurring among those 23 variables. This single variable also explains 37.4% of all the variability among the 50 variables. Variable -15645 has the highest correlation with the variables in its cluster. In summary, Cluster Variables enables very quick analysis of uniqueness to allow us to further identify important variables that need to be analyzed in greater detail.
The next step is to model our genes to see how the level of their expression influences the response (Tissue Stage). The Model Screening allows us to automatically find the model that best fits the data. From the list of models suggested, we can choose the ones that we want to apply. In our case, the XGBoost performed the best with an RSquare of 97%.
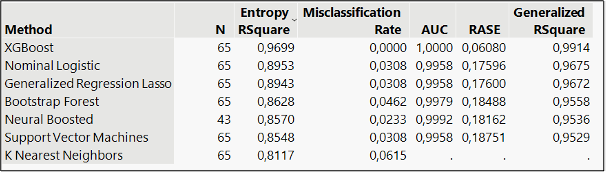
From the XG Boost model, the Prediction Profiler enables us to detect how the level of gene expression will affect the predicted value of the response. Here we can see the level of expression of five genes have an impact of the differentiation stage of the tissue.
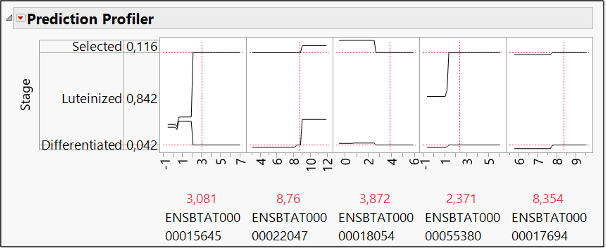
And just like that, JMP Pro 17 successfully identified a handful of biomarkers that differ in expression for different tissue types and tissue stages of preovulatory follicle development.
You must be a registered user to add a comment. If you've already registered, sign in. Otherwise, register and sign in.