Tony Cooper, PhD, Analytic Consultant, SAS
Sam Edgemon, Principal Technical Consultant, SAS
Principal components analysis (PCA) allows variable reduction and an understanding of the underlying structure of the data. JMP offers estimation methods for PCA such as REML, wide and sparse. The different methods can use different math. More importantly, the methods are applicable for different applications.
The motivation for this talk was data that included 50+ Symptoms every week for two years. One analysis path considered ways to summarize the data across symptoms (syndromes?) Each column described the count for a certain symptom in a time period.
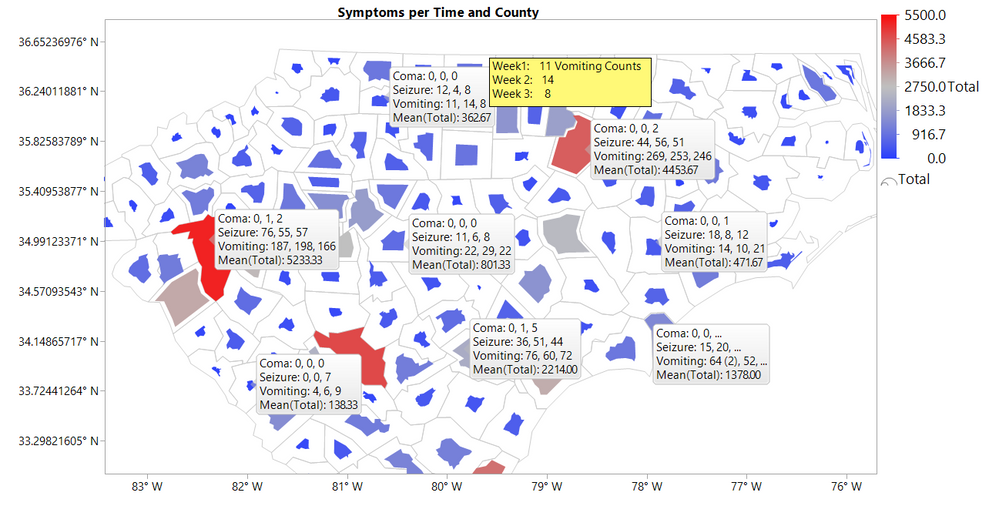
The author was very familiar with PCA on continuous data, but PCA requires a covariance matrix to summarize continuous data and these measures are counts. At the same time Text Mining was becoming more accessible and it often consider data in a Doc-Term Matrix:
This matrix also depends on counts! The math behind the dimension reduction in Text Mining is typically Singular Value Decomposition (SVD).
Then in recent years JMP has expanded it's Principal Components platform.
Wide and Sparse both implement SVD. There are options for PCA and SVD based. There are options for centering and scaling. There are modifications to the algotithms for particular situations. Examples will demonstrate implementing the options.
.